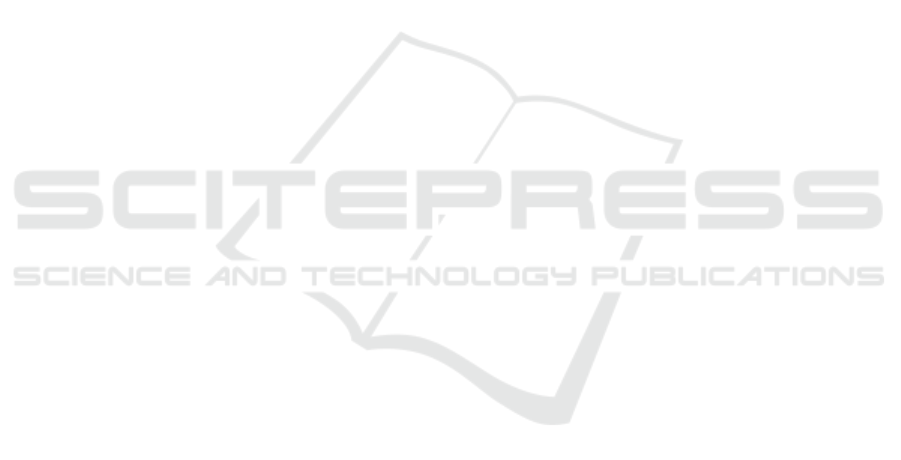
tion using random convolutional kernels. Data Mining
and Knowledge Discovery, 34(5):1454–1495.
Ehlers, J., Strauch, C., Georgi, J., and Huckauf, A. (2016).
Pupil size changes as an active information channel
for biofeedback applications. Applied psychophysiol-
ogy and biofeedback, 41:331–339.
Ehlers, J., Strauch, C., and Huckauf, A. (2018). A view
to a click: Pupil size changes as input command in
eyes-only human-computer interaction. International
journal of human-computer studies, 119:28–34.
Einh
¨
auser, W., Stout, J., Koch, C., and Carter, O.
(2008). Pupil dilation reflects perceptual selection
and predicts subsequent stability in perceptual rivalry.
Proceedings of the National Academy of Sciences,
105(5):1704–1709.
Fink, B. and Penton-Voak, I. (2002). Evolutionary psychol-
ogy of facial attractiveness. Current Directions in Psy-
chological Science, 11(5):154–158.
Gollan, B. and Ferscha, A. (2016). Modeling pupil dilation
as online input for estimation of cognitive load in non-
laboratory attention-aware systems. COGNITIVE.
Grimmer, J., Simon, L., and Ehlers, J. (2021). The cog-
nitive eye: Indexing oculomotor functions for mental
workload assessment in cognition-aware systems. In
Extended Abstracts of the 2021 CHI Conference on
Human Factors in Computing Systems, pages 1–6.
Hahn, A. C. and Perrett, D. I. (2014). Neural and behavioral
responses to attractiveness in adult and infant faces.
Neuroscience & Biobehavioral Reviews, 46:591–603.
Kardon, R. (1995). Pupillary light reflex. Current opinion
in ophthalmology, 6(6):20–26.
Karras, T., Laine, S., and Aila, T. (2019). A style-based
generator architecture for generative adversarial net-
works. In Proceedings of the IEEE/CVF conference
on computer vision and pattern recognition, pages
4401–4410.
Kucewicz, M. T., Dolezal, J., Kremen, V., Berry, B. M.,
Miller, L. R., Magee, A. L., Fabian, V., and Worrell,
G. A. (2018). Pupil size reflects successful encoding
and recall of memory in humans. Scientific reports,
8(1):4949.
Laeng, B. and Falkenberg, L. (2007). Women’s pupillary
responses to sexually significant others during the hor-
monal cycle. Hormones and behavior, 52(4):520–
530.
Leys, C., Ley, C., Klein, O., Bernard, P., and Licata, L.
(2013). Detecting outliers: Do not use standard devi-
ation around the mean, use absolute deviation around
the median. Journal of experimental social psychol-
ogy, 49(4):764–766.
Liao, H.-I., Kashino, M., and Shimojo, S. (2021). Attrac-
tiveness in the eyes: A possibility of positive loop be-
tween transient pupil constriction and facial attraction.
Journal of Cognitive Neuroscience, 33(2):315–340.
Love, J., Selker, R., Marsman, M., Jamil, T., Dropmann,
D., Verhagen, J., Ly, A., Gronau, Q. F.,
ˇ
Sm
´
ıra, M.,
Epskamp, S., et al. (2019). Jasp: Graphical statistical
software for common statistical designs. Journal of
Statistical Software, 88:1–17.
Mantiuk, R., Kowalik, M., Nowosielski, A., and Bazyluk,
B. (2012). Do-it-yourself eye tracker: Low-cost pupil-
based eye tracker for computer graphics applications.
In Advances in Multimedia Modeling: 18th Interna-
tional Conference, MMM 2012, Klagenfurt, Austria,
January 4-6, 2012. Proceedings 18, pages 115–125.
Springer.
Math
ˆ
ot, S., Dalmaijer, E., Grainger, J., and Van der
Stigchel, S. (2014). The pupillary light response re-
flects exogenous attention and inhibition of return.
Journal of vision, 14(14):7–7.
Naber, M., Fr
¨
assle, S., Rutishauser, U., and Einh
¨
auser, W.
(2013). Pupil size signals novelty and predicts later
retrieval success for declarative memories of natural
scenes. Journal of vision, 13(2):11–11.
Park, S., Zhang, X., Bulling, A., and Hilliges, O. (2018).
Learning to find eye region landmarks for remote gaze
estimation in unconstrained settings. In Proceedings
of the 2018 ACM symposium on eye tracking research
& applications, pages 1–10.
Pronk, T. M., Bogaers, R. I., Verheijen, M. S., and Sleegers,
W. W. (2021). Pupil size predicts partner choices in
online dating. Social Cognition, 39(6):773–786.
Rafiqi, S., Wangwiwattana, C., Fernandez, E., Nair, S.,
and Larson, E. (2015). Work-in-progress, pupilware-
m: Cognitive load estimation using unmodified smart-
phone cameras. In 2015 IEEE 12th International Con-
ference on Mobile Ad Hoc and Sensor Systems, pages
645–650. IEEE.
Rieger, G. and Savin-Williams, R. C. (2012). The eyes have
it: Sex and sexual orientation differences in pupil di-
lation patterns. PloS one, 7(8):e40256.
Ruiz, A. P., Flynn, M., Large, J., Middlehurst, M., and Bag-
nall, A. (2021). The great multivariate time series clas-
sification bake off: a review and experimental evalua-
tion of recent algorithmic advances. Data Mining and
Knowledge Discovery, 35(2):401–449.
Sawyer, A. N., Smith, E. R., and Benotsch, E. G.
(2018). Dating application use and sexual risk be-
havior among young adults. Sexuality Research and
Social Policy, 15:183–191.
Skakoon-Sparling, S., Cramer, K. M., and Shuper, P. A.
(2016). The impact of sexual arousal on sexual
risk-taking and decision-making in men and women.
Archives of sexual behavior, 45:33–42.
Wu, S. and Trottier, D. (2022). Dating apps: a literature
review. Annals of the International Communication
Association, 46(2):91–115.
Towards Automated Decision Making in Dating Apps Through Pupillary Responses
529