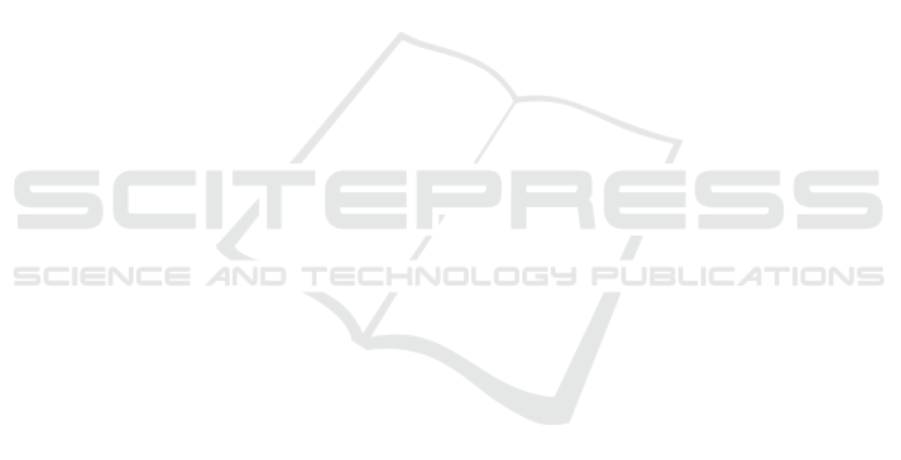
Kibalya, G., Serrat, J., Gorricho, J.-L., Okello, D., and
Zhang, P. (2023). A deep reinforcement learning-
based algorithm for reliability-aware multi-domain
service deployment in smart ecosystems. Neural
Computing and Applications, pages 23795–23817.
Maleki, E. F., Ma, W., Mashayekhy, L., and La Roche, H.
(2021). Qos-aware 5g component selection for con-
tent delivery in multi-access edge computing. In Pro-
ceedings of the 14th IEEE/ACM International Confer-
ence on Utility and Cloud Computing, pages 1–10.
Marshall, C. et al. (2016). Tool support for systematic re-
views in software engineering. PhD thesis, Keele Uni-
versity.
Mergel, G. D., Silveira, M. S., and da Silva, T. S. (2015).
A method to support search string building in system-
atic literature reviews through visual text mining. In
Proceedings of the 30th Annual ACM Symposium on
Applied Computing. Association for Computing Ma-
chinery.
Patel, S. B. and Lam, K. (2023). Chatgpt: the future of
discharge summaries? The Lancet Digital Health,
5(3):e107–e108.
Patel, Y. S., Reddy, M., and Misra, R. (2021). Energy and
cost trade-off for computational tasks offloading in
mobile multi-tenant clouds. Cluster Computing, pages
1–32.
Ray, P. P. (2023). Chatgpt: A comprehensive review on
background, applications, key challenges, bias, ethics,
limitations and future scope. Internet of Things and
Cyber-Physical Systems.
Sallam, M. (2023). Chatgpt utility in healthcare educa-
tion, research, and practice: systematic review on the
promising perspectives and valid concerns. Health-
care, page 887.
Sarker, I. H. (2022). Ai-based modeling: Techniques, ap-
plications and research issues towards automation, in-
telligent and smart systems. SN Computer Science.
Scells, H., Zuccon, G., and Koopman, B. (2019). Auto-
matic boolean query refinement for systematic review
literature search. In The World Wide Web Conference.
Association for Computing Machinery.
Scells, H., Zuccon, G., Koopman, B., and Clark, J. (2020).
Automatic boolean query formulation for systematic
review literature search. In Proceedings of The Web
Conference 2020. Association for Computing Ma-
chinery.
Sellak, H., Ouhbi, B., and Frikh, B. (2015). Using rule-
based classifiers in systematic reviews: a semantic
class association rules approach. In Proceedings of
the 17th International Conference on Information In-
tegration and Web-based Applications & Services. As-
sociation for Computing Machinery.
Sharma, H., Budhiraja, I., Consul, P., Kumar, N., Garg, D.,
Zhao, L., and Liu, L. (2022). Federated learning based
energy efficient scheme for mec with noma underlay-
ing uav. In Proceedings of the 5th international ACM
mobicom workshop on drone assisted wireless com-
munications for 5G and beyond, pages 73–78.
Snyder, H. (2019). Literature review as a research method-
ology: An overview and guidelines. Journal of busi-
ness research, pages 333–339.
Sun, P. and Naser, H. (2018). A service slicing strategy with
qos for lte-based cellular networks. In Proceedings of
the 14th ACM International Symposium on QoS and
Security for Wireless and Mobile Networks, pages 63–
69.
Thantharate, A. and Beard, C. (2023). Adaptive6g: adap-
tive resource management for network slicing archi-
tectures in current 5g and future 6g systems. Journal
of Network and Systems Management, page 9.
theresanaiforthat (2023). Easy-peasy ai accelerates content
creation. https://theresanaiforthat.com/ai/easy-peasy-
ai/?ref=search&term=Easy-peasy&fid=50. (Accessed
on 07/05/2023).
Theresanaiforthat (2023). pdf2gpt - summarize a pdf.
https://theresanaiforthat.com/ai/pdf2gpt/?ref=search
&term=Pdf2GPT. (Accessed on 07/04/2023).
theresanaiforthat (2023). Scispace - your ai
aopilot to decode any research paper.
https://theresanaiforthat.com/ai/scispace/?ref=search
&term=scispace. (Accessed on 07/04/2023).
ToolsPedia.io (2023). Chatpdf - chat with any pdf
files for free using ai. https://www.toolspedia.io/ai-
tool/chatpdf. (Accessed on 07/04/2023).
Tsafnat, G., Glasziou, P., Karystianis, G., and Coiera, E.
(2018). Automated screening of research studies for
systematic reviews using study characteristics. Sys-
tematic reviews.
van Dinter, R., Tekinerdogan, B., and Catal, C. (2021). Au-
tomation of systematic literature reviews: A system-
atic literature review. Information and Software Tech-
nology.
Velrajan, S. and Ceronmani Sharmila, V. (2023). Qos-aware
service migration in multi-access edge compute using
closed-loop adaptive particle swarm optimization al-
gorithm. Journal of Network and Systems Manage-
ment, page 17.
Verma, M. (2023). Novel study on ai-based chatbot (chat-
gpt) impacts on the traditional library management.
International Journal of Trend in Scientific Research
and Development (IJTSRD).
wondershare (2023). Hipdf’s ’chat with pdf’.
https://www.hipdf.com/chat-with-pdf. (Accessed
on 07/04/2023).
Yang, X., Li, Y., Zhang, X., Chen, H., and Cheng, W.
(2023). Exploring the limits of chatgpt for query
or aspect-based text summarization. arXiv preprint
arXiv:2302.08081.
Yenduri, G., Srivastava, G., Maddikunta, P. K. R., Jhaveri,
R. H., Wang, W., Vasilakos, A. V., Gadekallu, T. R.,
et al. (2023). Generative pre-trained transformer: A
comprehensive review on enabling technologies, po-
tential applications, emerging challenges, and future
directions. arXiv preprint arXiv:2305.10435.
Zhu, J.-J., Jiang, J., Yang, M., and Ren, Z. J. (2023). Chat-
gpt and environmental research. Environmental Sci-
ence & Technology.
Towards the Use of AI-Based Tools for Systematic Literature Review
603