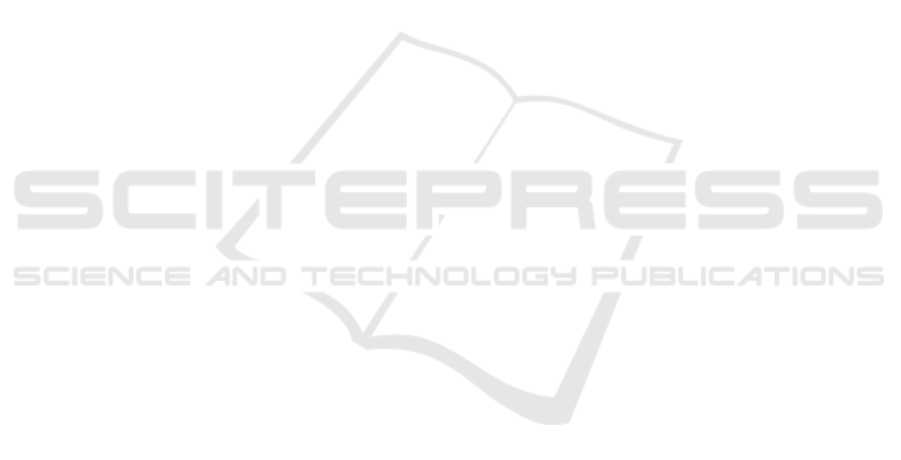
delivery system and the profitability of the retailer
business. Recent researches showed the potentials
for the establishment of new multi-level FCs to facili-
tate last-mile drone delivery services across European
cities (Aurambout et al., 2022).
The appealing features of drone-based delivery
solutions in terms of low environmental implications
(CO
2
footprint and noise pollution) have somehow
shifted the focus of practitioners and operational re-
searchers to its green sustainability, ignoring or even
downplaying the importance of addressing economic
viability measures in delivery systems.
The present study aims to address the economic
sustainability of drone-based delivery services with
FCs through the design of an efficient delivery sys-
tem operating on a daily basis, where drone-specific
features, as energy consumption and recharge time,
along with customers’ preferences in terms of deliv-
ery time are properly incorporated. To design such
a holistic delivery service, we adopt a multi-period
framework that enables us to capture the inherent dy-
namicity of problem in terms of time-variant tariffs
and delivery revenue.
We should highlight that the economic sustain-
ability of system is highly affected by the retailer de-
cisions on how (questioning the transport mode) and
when the delivery plans are scheduled. With the aim
of maximizing the retailer total profit over a short-
term planning horizon of one working day, we present
the optimal delivery plan specifying i) the transport
mode and the delivery schedule for each customer; ii)
the deployment schedule for each drone; iii) the set
of FCs used to host the deployed drones. We also in-
vestigate the impact of different tariff setting policies
on the system economic viability providing the logis-
tics companies/the FC manager with managerial in-
sights on how the appropriate share of FCs resources
among different retailers could help to establish a sta-
ble platform for e-logistics businesses. In this way,
we address the economic viability and sustainability
of drone-deliveries with FCs in B2C logistics, inves-
tigating the effect of tariffs on both the retailer’s rev-
enue and the sustainability of delivery system.
The rest of the paper is organized as follows. Sec-
tion 2 presents a review on pure-drone delivery prob-
lem in the literature pointing up the gaps. Section
3 describes the problem and presents the mathemat-
ical formulation. Section 4 is devoted to the compu-
tational experiments conducted on a real case study
for the Portland city adapted from (Chauhan et al.,
2019) and the discussion on managerial insights. Fi-
nally, Section 5 provides the concluding remarks and
potential directions for future research.
2 LITERATURE REVIEW
Large part of the literature on UAV-based logistics
systems is devoted to contributions on multi-modal
delivery models where a fleet of drones and au-
tonomous trucks cooperate to serve customers (Bruni
et al., 2022; Dell’Amico et al., 2022; Moshref-Javadi
and Winkenbach, 2021). However, the present con-
tribution falls in the class of pure-play drone-based
models where deliveries are handled just by drones.
The literature on pure drone delivery problem
is categorized in two main streams depending on
whether drones perform multi-visit or single visit
tours. The multi-visit case involves routing deci-
sions where drones can visit multiple customers per
trip (Torabbeigi et al., 2020).This, of course, limits
the applicability of proposed models to cases wherein
the drone payload capacity and battery charge al-
low multiple deliveries per trip and requires to ac-
count for load-dependent energy consumption rates in
drone battery. Following this stream, (Dorling et al.,
2016) and (Cheng et al., 2020) presented multi-visit
drone delivery models where the energy consumption
in drone battery is explicitly modeled. (Bruni et al.,
2023b) studied a routing problem for last-mile drone
delivery with shared FCs and homogeneous parcel
weight where the objective is to minimize the total
travel cost (Bruni et al., 2023b). The authors model
the energy consumption rates considering internal and
external factors that affect energy consumption, in-
cluding drone-specific features (such as the number
of rotors, the drone frame and battery mass), the envi-
ronmental factors (air density and gravitational force),
and the mass of order(s) carried by drone. There are
also other contributions on multi-visit drone delivery
problem that account for energy consumption rates
under the travel time uncertainty that is not the fo-
cus of present paper, and therefore, we refer the inter-
ested reader to (Bruni and Khodaparasti, 2022a; Bruni
et al., 2023a; Bruni and Khodaparasti, 2022b).
As mentioned earlier, the limited drone flight
endurance, payload and battery capacity makes the
adoption of routing plans in many drone delivery ap-
plications impractical. On the other hand, the sin-
gle visit drone delivery case where each drone per-
forms multiple trips delivering the order of one sin-
gle customer per trip is more realistic and practical,
as supported by many contributions in the literature
(Chauhan et al., 2019; Figliozzi, 2020; Pani et al.,
2020; Dukkanci et al., 2021).
Following the single visit drone delivery context,
we may recognize that some contributions model the
energy consumption rates in drone battery as a lin-
ear function in terms of drone payload and travel time
Economic Sustainability in Last-Mile Drone Delivery Problem with Fulfillment Centers: A Mathematical Formulation
383