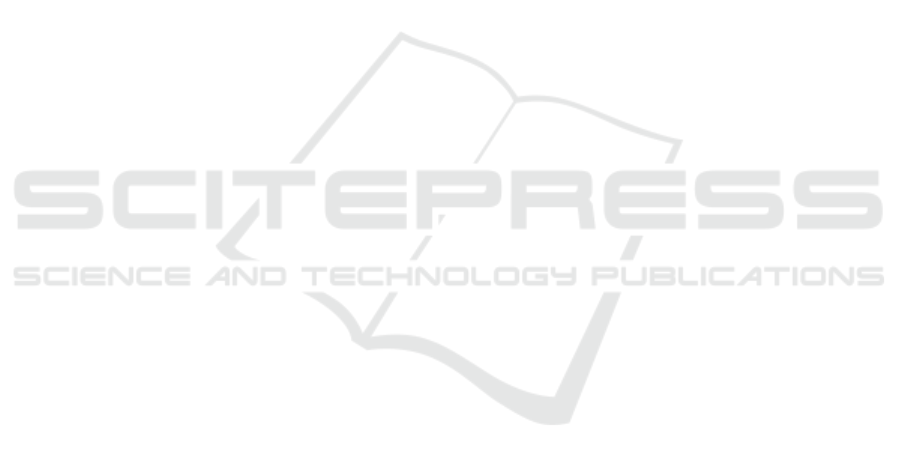
techniques, with classification performance serving as
the evaluation metric.
ACKNOWLEDGEMENTS
This work was funded by the German Ministry of
Education and Research (Bundesministerium f
¨
ur Bil-
dung und Forschung) as part of the project “KI-
unterst
¨
utzter Telenotarzt (KIT2)” under grant number
13N16402. We would like to thank them for their sup-
port.
REFERENCES
Aachen, U. (2023). https://www.telenotarzt.de/. Teleno-
tarzt.
Abiri, N., Linse, B., Ed
´
en, P., and Ohlsson, M. (2019). Es-
tablishing strong imputation performance of a denois-
ing autoencoder in a wide range of missing data prob-
lems. Neurocomputing, 365:137–146.
Adhikari, D., Jiang, W., Zhan, J., He, Z., Rawat, D. B.,
Aickelin, U., and Khorshidi, H. A. (2022). A Com-
prehensive Survey on Imputation of Missing Data
in Internet of Things. ACM Computing Surveys,
55(7):133:1–133:38.
Chang, Y.-W., Natali, L., Jamialahmadi, O., Romeo, S.,
Pereira, J. B., Volpe, G., and Initiative, f. t. A. D. N.
(2022). Neural network training with highly incom-
plete medical datasets. Machine Learning: Science
and Technology, 3(3):035001. Publisher: IOP Pub-
lishing.
Chen, S. and Guo, W. (2023). Auto-encoders in deep learn-
ing—a review with new perspectives. Mathe-
matics, 11(8).
Coto-Jimenez, M., Goddard-Close, J., Di Persia, L.,
and Leonardo Rufiner, H. (2018). Hybrid Speech
Enhancement with Wiener filters and Deep LSTM
Denoising Autoencoders. In 2018 IEEE Interna-
tional Work Conference on Bioinspired Intelligence
(IWOBI), pages 1–8.
Emmanuel, T., Maupong, T., Mpoeleng, D., Semong, T.,
Mphago, B., and Tabona, O. (2021). A survey on
missing data in machine learning. Journal of Big
Data, 8(1):140.
Hameed, W. M. and Ali, N. A. (2023). Missing value im-
putation techniques: A survey. UHD J. Sci. Technol.,
7(1):72–81.
Ho, N.-H., Yang, H.-J., Kim, J., Dao, D.-P., Park, H.-R., and
Pant, S. (2022). Predicting progression of Alzheimer’s
disease using forward-to-backward bi-directional net-
work with integrative imputation. Neural Networks,
150:422–439.
Kabir, S. and Farrokhvar, L. (2022). Non-linear missing
data imputation for healthcare data via index-aware
autoencoders. Health Care Management Science,
25(3):484–497.
Liu, M., Li, S., Yuan, H., Ong, M. E. H., Ning, Y., Xie,
F., Saffari, S. E., Shang, Y., Volovici, V., Chakraborty,
B., and Liu, N. (2023a). Handling missing values in
healthcare data: A systematic review of deep learning-
based imputation techniques. Artificial Intelligence in
Medicine, 142:102587.
Liu, M., Li, S., Yuan, H., Ong, M. E. H., Ning, Y., Xie,
F., Saffari, S. E., Shang, Y., Volovici, V., Chakraborty,
B., and Liu, N. (2023b). Handling missing values in
healthcare data: A systematic review of deep learning-
based imputation techniques. Artificial Intelligence in
Medicine, 142:102587.
Lu, X., Yuan, L., Li, R., Xing, Z., Yao, N., and Yu,
Y. (2023). An Improved Bi-LSTM-Based Missing
Value Imputation Approach for Pregnancy Examina-
tion Data. Algorithms, 16(1):12. Number: 1 Pub-
lisher: Multidisciplinary Digital Publishing Institute.
Ma, Q., Lee, W.-C., Fu, T.-Y., Gu, Y., and Yu, G. (2020).
MIDIA: exploring denoising autoencoders for missing
data imputation. Data Mining and Knowledge Discov-
ery, 34(6):1859–1897.
Macias, E., Serrano, J., Vicario, J. L., and Morell, A.
(2021). Novel Imputation Method Using Average
Code from Autoencoders in Clinical Data. In 2020
28th European Signal Processing Conference (EU-
SIPCO), pages 1576–1579. ISSN: 2076-1465.
Mongia, A., Sengupta, D., and Majumdar, A. (2020).
deepMc: Deep Matrix Completion for Imputation of
Single-Cell RNA-seq Data. Journal of Computational
Biology, 27(7):1011–1019. Publisher: Mary Ann
Liebert, Inc., publishers.
Pan, H., Ye, Z., He, Q., Yan, C., Yuan, J., Lai, X., Su, J.,
and Li, R. (2022). Discrete Missing Data Imputation
Using Multilayer Perceptron and Momentum Gradi-
ent Descent. Sensors, 22(15):5645. Number: 15 Pub-
lisher: Multidisciplinary Digital Publishing Institute.
Psychogyios, K., Ilias, L., and Askounis, D. (2022). Com-
parison of Missing Data Imputation Methods us-
ing the Framingham Heart study dataset. In 2022
IEEE-EMBS International Conference on Biomed-
ical and Health Informatics (BHI), pages 1–5.
arXiv:2210.03154 [cs].
Sun, Y., Li, J., Xu, Y., Zhang, T., and Wang, X. (2023).
Deep learning versus conventional methods for miss-
ing data imputation: A review and comparative study.
Expert Systems with Applications, 227:120201.
Tyagi, K., Rane, C., Harshvardhan, and Manry, M. (2022).
Regression analysis. In Artificial Intelligence and Ma-
chine Learning for EDGE Computing, pages 53–63.
Elsevier.
Vincent, P., Larochelle, H., Bengio, Y., and Manzagol, P.-
A. (2008). Extracting and composing robust features
with denoising autoencoders. In Proceedings of the
25th international conference on Machine learning -
ICML ’08, pages 1096–1103, Helsinki, Finland. ACM
Press.
Vincent, P., Larochelle, H., Lajoie, I., Bengio, Y., and Man-
zagol, P.-A. Stacked Denoising Autoencoders: Learn-
ing Useful Representations in a Deep Network with a
Local Denoising Criterion.
Wang, Y., Yao, H., and Zhao, S. (2016). Auto-encoder
based dimensionality reduction. Neurocomputing,
184:232–242.
ICAART 2024 - 16th International Conference on Agents and Artificial Intelligence
1236