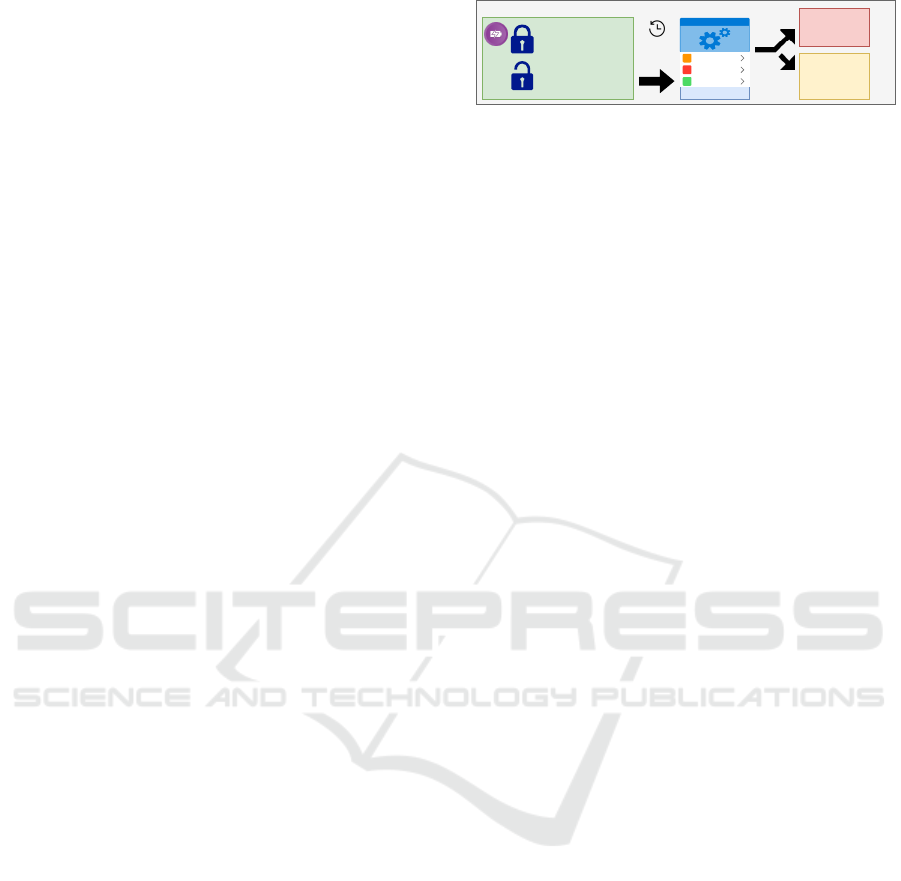
However, developing an actionable strategy for in-
vesting in diverse modalities to achieve carbon neu-
trality is challenging due to (1) the complexity of the
heterogeneous infrastructures and their components
which interact with each other during operation in a
non-trivial way; (2) the need to balance financial, en-
vironmental and quality of service KPIs, particularly
when these objectives conflict; (3) the dependence
of investment performance on operational efficiency
(e.g., over 30-minute operational intervals) (4) the
management of uncertainties arising from fluctuations
in supply, demand, and market prices which are typi-
cally not steady-state but transient; (5) the adaptation
to the ever-evolving landscape of carbon-reducing
technologies and regulations; and (6) interoperation
with pre-existing infrastructures constructed without
considering emissions.
Due to the challenges outlined, progress toward
carbon neutrality has not met the anticipated rates
or scales. While there have been notable enhance-
ments in the energy efficiency of some infrastruc-
tures, investments across mixed infrastructures are not
achieving their full potential. Therefore, it is essential
for stakeholders to formulate strategies that consider
multiple infrastructures and resources simultaneously,
placing a spotlight on the synergy between these in-
frastructures during operations.
In our review of past case studies designed
to assist stakeholders in attaining carbon neutrality
goals, represented by studies like (Alshuwaikhat and
Abubakar, 2008) (Roberto et al., 2018) and (Kourgio-
zou et al., 2021), a common limitation became ap-
parent. While these studies aimed to provide prac-
tical guidance, many of them presented frameworks
that, rather than offering a clear and direct action plan,
tended to provide broad theoretical guidelines.
Many case studies, exemplified by (Hanus et al.,
2019) and (Li and Jia, 2022), develop actionable plans
to assist stakeholders in formulating effective strate-
gies to achieve carbon neutrality. However, a common
drawback in these studies is their tendency to either
narrowly focus on limited interventions or neglect the
exploration of the intricate dynamics of operational
interplay.
Some case studies utilize design guidance tools
to craft actionable plans using a mix of modali-
ties for power systems in microgrids to achieve car-
bon targets. These studies aim to utilize operational
control to optimize system configurations, attaining
cost-effective and efficient solutions in carbon reduc-
tion. Examples of such studies are (Danish et al.,
2023) and (Venkatachary et al., 2017), which utilize
HOMER (Lambert et al., 2006), a simulation model
developed by the National Renewable Energy Lab-
Analytical Models
Fixed Parameter
Controllable Parameter
Capacity
Depreciation rate
...
Number of Battery
...
Battery
Extendable library
Metrics
Constraints
cost
co2e
...
capacity constraint
flow balancing
...
...
Solar Panels
Battery
Contract
Input
Operational
Interval
(e.g., 30 min)
Figure 2: Atomic Analytical Models.
oratory (NREL), to reach optimal system configura-
tions. However, these tools depend on simulations
to reach optimal solutions. In contrast, optimization
tools grounded in mathematical programming tend
to yield superior results, excelling in both optimal-
ity of results and computational efficiency. Addition-
ally, these tools were not initially designed for multi-
period investment optimization; they often enforce in-
vestments at the beginning, which may not be opti-
mally cost-effective, especially when dealing with rel-
atively new technologies subject to changing maturity
levels and prices over time.
In our recent study (Alyahya and Brodsky, 2023),
we introduced the Green Assessment and Decision
GuidancE Tool (GADGET) to address the aforemen-
tioned limitations. GADGET produces actionable
Pareto-optimal recommendations with respect two
sets of metrics: (1) financial metrics including Present
Value Cost (PVC) and IRR, and (2) environmental
metrics, such as CO
2
e emissions. GADGET de-
ploys a Mixed Integer Linear Programming (MILP)
solver and is based on the Service Network Invest-
ment Model (SNIM) (Alyahya and Brodsky, 2021),
which calculates cash flows and performance met-
rics. SNIM formalizes both (1) nontrivial operational
interactions within these infrastructures (e.g., at 30-
minute intervals), and (2) investment controls at dif-
ferent stages in the time horizon (e.g., every 5 years
over 25 years). Simultaneous optimization of these
two control levels enables a more effective utilization
of the infrastructure, minimizing waste that could oc-
cur when addressing each component in isolation.
In this study we employ GADGET to provide ac-
tionable investment recommendations to George Ma-
son University’s stakeholders on a mix of interrelated
infrastructures for its Fairfax campus with the ini-
tial scope considering renewable energy certificates
(RECs) and carbon offsets, gas and electric boilers, on
site solar arrays, energy storage, and Dominion Vir-
ginia Energy contract schedule. Our analysis covers
the investment time horizon from 2025 to 2050, con-
sidering investment decisions at five-year intervals,
with the overarching goal of achieving carbon neu-
trality by 2040.
We produce optimal actionable recommendations
for a number of scenarios associated with carbon neu-
trality targets ranging from 2040 to 2025, as well
as baseline scenarios of business-as-usual, RECs and
Toward Pareto-Optimal Investment Mix to Achieve Carbon Neutrality: A Case Study
373