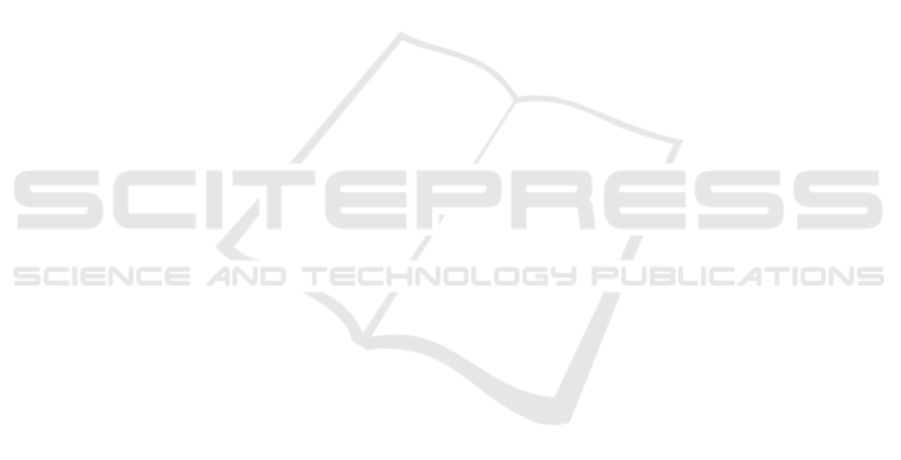
Fernando, J. and Dawson, L. (2008). Clinician assessments
of workplace security training-an informatics perspec-
tive. Electron. J. Health Inform, 3(1):e7.
Forssberg, K. S., V
¨
anje, A., and Parding, K. (2022). Bring-
ing in gender perspectives on systematic occupa-
tional safety and health management. Safety Science,
152:105776.
Gajera, K., Jangid, M., Mehta, P., and Mittal, J. (2019). A
novel approach to detect phishing attack using artifi-
cial neural networks combined with pharming detec-
tion. In 2019 3rd International conference on Elec-
tronics, Communication and Aerospace Technology
(ICECA), pages 196–200. IEEE.
Guo, Y., Zhang, Z., and Guo, Y. (2019). Optiwords: A new
password policy for creating memorable and strong
passwords. Computers & Security, 85:423–435.
Gupta, B. B. and Jain, A. K. (2020). Phishing attack detec-
tion using a search engine and heuristics-based tech-
nique. Journal of Information Technology Research
(JITR), 13(2):94–109.
Hoofnagle, C. J., van der Sloot, B., and Borgesius, F. Z.
(2019). The european union general data protection
regulation: what it is and what it means. Information
& Communications Technology Law, 28(1):65–98.
Ishikawa, K., Ohmichi, H., Umesato, Y., Terasaki, H.,
Tsukuma, H., Iwata, N., Tanaka, T., Kawamura, A.,
Sakata, K., Sainohara, T., et al. (2007). The guideline
of the personal health data structure to secure safety
healthcare: The balance between use and protection
to satisfy the patients’ needs. international journal of
medical informatics, 76(5):412–418.
K
¨
avrestad, J., Lennartsson, M., Birath, M., and Nohlberg,
M. (2020). Constructing secure and memorable pass-
words. Information & Computer Security.
Khando, K., Gao, S., Islam, S. M., and Salman, A. (2021).
Enhancing employees information security awareness
in private and public organisations: A systematic lit-
erature review. Computers & Security, 106:102267.
Kierkegaard, P. (2012). Medical data breaches: Notifica-
tion delayed is notification denied. Computer Law &
Security Review, 28(2):163–183.
Mammadova, M. (2015). The Problems of Information
Security of Electronic Personal Health Data. In 7th
International Conference on Information Technology
in Medicine and Education (ITME), pages 678–682.
IEEE.
McDermott, D. S., Kamerer, J. L., and Birk, A. T. (2019).
Electronic health records: A literature review of cyber
threats and security measures. International Journal
of Cyber Research and Education (IJCRE), 1(2):42–
49.
Murphy, J., Stramer, K., Clamp, S., Grubb, P., Gosland,
J., and Davis, S. (2004). Health informatics educa-
tion for clinicians and managers—What’s holding up
progress? International journal of medical informat-
ics, 73(2):205–213.
Price, W. N. and Cohen, I. G. (2019). Privacy in the age of
medical big data. Nature medicine, 25(1):37–43.
Saura, J. R., Ribeiro-Soriano, D., and Palacios-Marqu
´
es, D.
(2022). Assessing behavioral data science privacy is-
sues in government artificial intelligence deployment.
Government Information Quarterly, page 101679.
Sharma, T. and Bashir, M. (2020). An analysis of phish-
ing emails and how the human vulnerabilities are ex-
ploited. In International Conference on Applied Hu-
man Factors and Ergonomics, pages 49–55. Springer.
Sivan, R. and Zukarnain, Z. A. (2021). Security and privacy
in cloud-based e-health system. Symmetry, 13(5):742.
ˇ
Sv
´
abensk
`
y, V.,
ˇ
Celeda, P., Vykopal, J., and Bri
ˇ
s
´
akov
´
a, S.
(2021). Cybersecurity knowledge and skills taught in
capture the flag challenges. Computers & Security,
102:102154.
Tseng, H.-T., Ibrahim, F., Hajli, N., Nisar, T. M., and Shab-
bir, H. (2022). Effect of privacy concerns and en-
gagement on social support behaviour in online health
community platforms. Technological Forecasting and
Social Change, 178:121592.
US-CERT (2016). How to protect your networks from ran-
somware. Technical report, Department of Homeland
Security. United States Computer Emergency Readi-
ness Team.
Veroni, E., Ntantogian, C., and Xenakis, C. (2022). A large-
scale analysis of wi-fi passwords. Journal of Informa-
tion Security and Applications, 67:103190.
Vincent, A. (2019). Don’t feed the phish: how to avoid
phishing attacks. Network Security, 2019(2):11–14.
Zabihimayvan, M. and Doran, D. (2019). Fuzzy rough set
feature selection to enhance phishing attack detection.
In 2019 IEEE International Conference on Fuzzy Sys-
tems (FUZZ-IEEE), pages 1–6. IEEE.
Zegers, C. M., Witteveen, A., Schulte, M. H., Henrich, J. F.,
Vermeij, A., Klever, B., and Dekker, A. (2021). Mind
your data: Privacy and legal matters in ehealth. JMIR
formative research, 5(3):e17456.
Zhang, L., Guo, Y., Guo, X., and Shao, X. (2021). Does the
layout of the android unlock pattern affect the security
and usability of the password? Journal of Information
Security and Applications, 62:103011.
An Empirical Analysis of Undergraduate Information Systems Security Behaviors
627