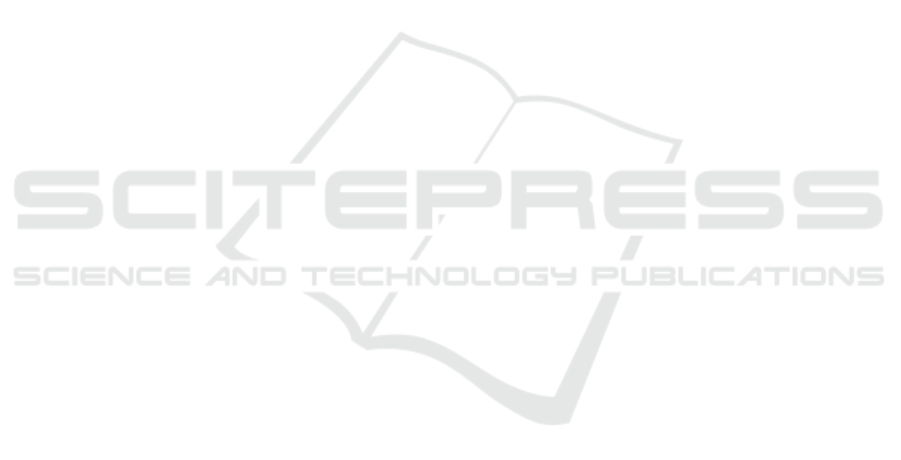
Chen, S., Liu, B., Feng, C., Vallespi-Gonzalez, C., and
Wellington, C. (2021b). 3d point cloud processing and
learning for autonomous driving: Impacting map cre-
ation, localization, and perception. IEEE Signal Pro-
cessing Magazine, 38(1):68–86.
Dai, A., Chang, A. X., Savva, M., Halber, M., Funkhouser,
T., and Nießner, M. (2017). Scannet: Richly-
annotated 3d reconstructions of indoor scenes. In
Proc. Computer Vision and Pattern Recognition
(CVPR). IEEE.
Dimitrov, A. and Golparvar-Fard, M. (2015). Segmentation
of building point cloud models including detailed ar-
chitectural/structural features and mep systems. Au-
tomation in Construction, 51(C):32–45. Publisher
Copyright: © 2014 Elsevier B.V. All rights reserved.
Engelmann, F., Kontogianni, T., Schult, J., and Leibe, B.
(2019). Know what your neighbors do: 3d semantic
segmentation of point clouds, page 395–409. Springer
International Publishing.
Gumhold, S., Wang, X., and Macleod, R. (2001). Feature
extraction from point clouds. In International Mesh-
ing Roundtable Conference.
Guo, Y., Bennamoun, M., Sohel, F., Lu, M., Wan, J., and
Kwok, N. (2015). A comprehensive performance
evaluation of 3d local feature descriptors. Interna-
tional Journal of Computer Vision, 116.
Guo, Y., Wang, H., Hu, Q., Liu, H., Liu, L., and Ben-
namoun, M. (2020). Deep learning for 3d point
clouds: A survey.
Gyawali, D. (2023). Lrtransformer: Learn-region trans-
former for object-agnostic point cloud segmentation.
Master’s thesis, Louisiana State University.
Hu, P., Held, D., and Ramanan, D. (2019). Learning to
optimally segment point clouds.
Kang, C. L., Wang, F., Zong, M. M., Cheng, Y., and Lu,
T. N. (2020). Research on improved region growing
point cloud algorithm. The International Archives of
the Photogrammetry, Remote Sensing and Spatial In-
formation Sciences, XLII-3/W10:153–157.
Ling, C. F., Dang, S. W., Zhang, C. Y., and Chen, Y. (2021).
Research and application of semantic point cloud on
indoor robots. In 2021 5th International Conference
on Communication and Information Systems (ICCIS),
pages 108–113.
Mirzaei, K., Arashpour, M., Asadi, E., et al. (2022). Auto-
matic generation of structural geometric digital twins
from point clouds. Sci Rep, 12:22321.
Nguyen, A. and Le, B. (2013). 3d point cloud segmentation:
A survey. In 2013 6th IEEE Conference on Robotics,
Automation and Mechatronics (RAM), page 225–230.
Nunes, L., Chen, X., Marcuzzi, R., Osep, A., Leal-Taixe,
L., and Stachniss, C. (2022). 3d point cloud clustering
with learnable robust geometric constraints.
Pham, Q.-H., Nguyen, D. T., Hua, B.-S., Roig, G., and
Yeung, S.-K. (2019). Jsis3d: Joint semantic-instance
segmentation of 3d point clouds with multi-task point-
wise networks and multi-value conditional random
fields.
Placitelli, A. P. and Gallo, L. (2011). Low-cost augmented
reality systems via 3d point cloud sensors. In 2011
Seventh International Conference on Signal Image
Technology & Internet-Based Systems, pages 188–
192.
Qi, C. R., Su, H., Mo, K., and Guibas, L. J. (2017a). Point-
net: Deep learning on point sets for 3d classification
and segmentation.
Qi, C. R., Yi, L., Su, H., and Guibas, L. J. (2017b). Point-
net++: Deep hierarchical feature learning on point sets
in a metric space.
Rabbani, T., van den Heuvel, F., and Vosselman, G. (2006).
Segmentation of point clouds using smoothness con-
straints. In Maas, H. and Schneider, D., editors, IS-
PRS 2006 : Proceedings of the ISPRS commission V
symposium, volume 35, pages 248–253. International
Society for Photogrammetry and Remote Sensing (IS-
PRS). ISPRS commission V symposium : image.
Rusu, R. B., Blodow, N., and Beetz, M. (2009). Fast point
feature histograms (fpfh) for 3d registration. In 2009
IEEE International Conference on Robotics and Au-
tomation, pages 3212–3217.
Sharma, A., Khan, N., Sundaramoorthi, G., and Torr, P.
(2020). Class-agnostic segmentation loss and its ap-
plication to salient object detection and segmentation.
Vaswani, A., Shazeer, N., Parmar, N., Uszkoreit, J., Jones,
L., Gomez, A. N., Kaiser, L., and Polosukhin, I.
(2023). Attention is all you need.
Wang, L. and Yuan, B. (2010). Curvature and density
based feature point detection for point cloud data. In
IET 3rd International Conference on Wireless, Mo-
bile and Multimedia Networks (ICWMNN 2010), page
377–380.
Xiao, A., Huang, J., Guan, D., Zhang, X., Lu, S., and Shao,
L. (2023). Unsupervised point cloud representation
learning with deep neural networks: A survey. IEEE
Transactions on Pattern Analysis and Machine Intel-
ligence, pages 1–20.
Xie, C., Xiang, Y., Mousavian, A., and Fox, D. (2021). Un-
seen object instance segmentation for robotic environ-
ments.
Yang, B., Wang, J., Clark, R., Hu, Q., Wang, S., Markham,
A., and Trigoni, N. (2019). Learning object bounding
boxes for 3d instance segmentation on point clouds.
Zhao, H., Jia, J., and Koltun, V. (2020). Exploring self-
attention for image recognition.
Zhao, H., Jiang, L., Jia, J., Torr, P., and Koltun, V. (2021a).
Point transformer.
Zhao, N., Chua, T.-S., and Lee, G. H. (2021b). Few-shot 3d
point cloud semantic segmentation.
Zhao, R., Pang, M., Liu, C., and Zhang, Y. (2019). Robust
normal estimation for 3d lidar point clouds in urban
environments. Sensors, 19(5).
Zhou, J., Jin, W., Wang, M., Liu, X., Li, Z., and Liu, Z.
(2021). Fast and accurate normal estimation for point
cloud via patch stitching.
VISAPP 2024 - 19th International Conference on Computer Vision Theory and Applications
348