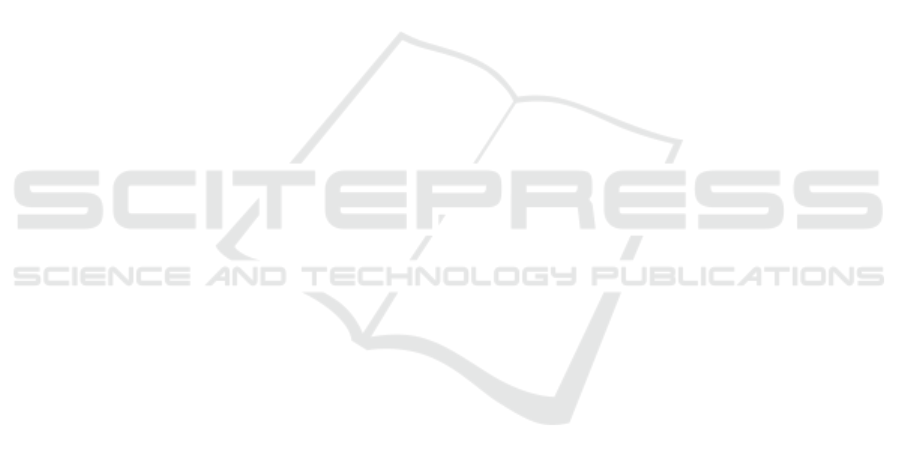
ACKNOWLEDGEMENTS
This research is partially supported by JSPS KAK-
ENHI Grant Number 21K11971.
REFERENCES
Carion, N., Massa, F., Synnaeve, G., Usunier, N., Kirillov,
A., and Zagoruyko, S. (2020). End-to-end object de-
tection with transformers. In European conference on
computer vision, pages 213–229. Springer.
Chen, J., Lu, Y., Yu, Q., Luo, X., Adeli, E., Wang, Y., Lu, L.,
Yuille, A. L., and Zhou, Y. (2021). Transunet: Trans-
formers make strong encoders for medical image seg-
mentation. arXiv preprint arXiv:2102.04306.
Chu, P., Wang, J., You, Q., Ling, H., and Liu, Z.
(2021). Transmot: Spatial-temporal graph trans-
former for multiple object tracking. arXiv preprint
arXiv:2104.00194.
Dosovitskiy, A., Beyer, L., Kolesnikov, A., Weissenborn,
D., Zhai, X., Unterthiner, T., Dehghani, M., Minderer,
M., Heigold, G., Gelly, S., et al. (2020). An image is
worth 16x16 words: Transformers for image recogni-
tion at scale. arXiv preprint arXiv:2010.11929.
Gerhard, S., Funke, J., Martel, J., Cardona, A., and Fet-
ter, R. (2013). Segmented anisotropic sstem dataset of
neural tissue. figshare, pages 0–0.
Guibas, J., Mardani, M., Li, Z., Tao, A., Anandkumar,
A., and Catanzaro, B. (2021). Efficient token mixing
for transformers via adaptive fourier neural operators.
In International Conference on Learning Representa-
tions.
Liu, Z., Lin, Y., Cao, Y., Hu, H., Wei, Y., Zhang, Z., Lin,
S., and Guo, B. (2021). Swin transformer: Hierarchi-
cal vision transformer using shifted windows. In Pro-
ceedings of the IEEE/CVF International Conference
on Computer Vision, pages 10012–10022.
McInnes, L., Healy, J., and Melville, J. (2018). Umap: Uni-
form manifold approximation and projection for di-
mension reduction. arXiv preprint arXiv:1802.03426.
Meinhardt, T., Kirillov, A., Leal-Taixe, L., and Feichten-
hofer, C. (2022). Trackformer: Multi-object tracking
with transformers. In Proceedings of the IEEE/CVF
Conference on Computer Vision and Pattern Recogni-
tion, pages 8844–8854.
Ronneberger, O., Fischer, P., and Brox, T. (2015). U-
net: Convolutional networks for biomedical image
segmentation. In Medical Image Computing and
Computer-Assisted Intervention–MICCAI 2015: 18th
International Conference, Munich, Germany, October
5-9, 2015, Proceedings, Part III 18, pages 234–241.
Springer.
Roth, K., Pemula, L., Zepeda, J., Sch
¨
olkopf, B., Brox, T.,
and Gehler, P. V. (2021). Towards total recall in indus-
trial anomaly detection. CoRR, abs/2106.08265.
Sakaridis, C., Dai, D., and Van Gool, L. (2021). Acdc: The
adverse conditions dataset with correspondences for
semantic driving scene understanding. In Proceedings
of the IEEE/CVF International Conference on Com-
puter Vision, pages 10765–10775.
Sethi, A. et al. (2021). Wavemix: Multi-resolution token
mixing for images.
Tan, C.-H., Chen, Q., Wang, W., Zhang, Q., Zheng, S., and
Ling, Z.-H. (2021). Ponet: Pooling network for effi-
cient token mixing in long sequences. arXiv preprint
arXiv:2110.02442.
Vaswani, A., Shazeer, N., Parmar, N., Uszkoreit, J., Jones,
L., Gomez, A. N., Kaiser, Ł., and Polosukhin, I.
(2017). Attention is all you need. Advances in neural
information processing systems, 30.
Xie, E., Wang, W., Yu, Z., Anandkumar, A., Alvarez, J. M.,
and Luo, P. (2021). Segformer: Simple and efficient
design for semantic segmentation with transformers.
Advances in Neural Information Processing Systems,
34:12077–12090.
Yu, W., Luo, M., Zhou, P., Si, C., Zhou, Y., Wang, X., Feng,
J., and Yan, S. (2022). Metaformer is actually what
you need for vision. In Proceedings of the IEEE/CVF
Conference on Computer Vision and Pattern Recogni-
tion, pages 10819–10829.
Zeng, F., Dong, B., Zhang, Y., Wang, T., Zhang, X., and
Wei, Y. (2022). Motr: End-to-end multiple-object
tracking with transformer. In European Conference
on Computer Vision, pages 659–675. Springer.
Improvement of TransUNet Using Word Patches Created from Different Dataset
385