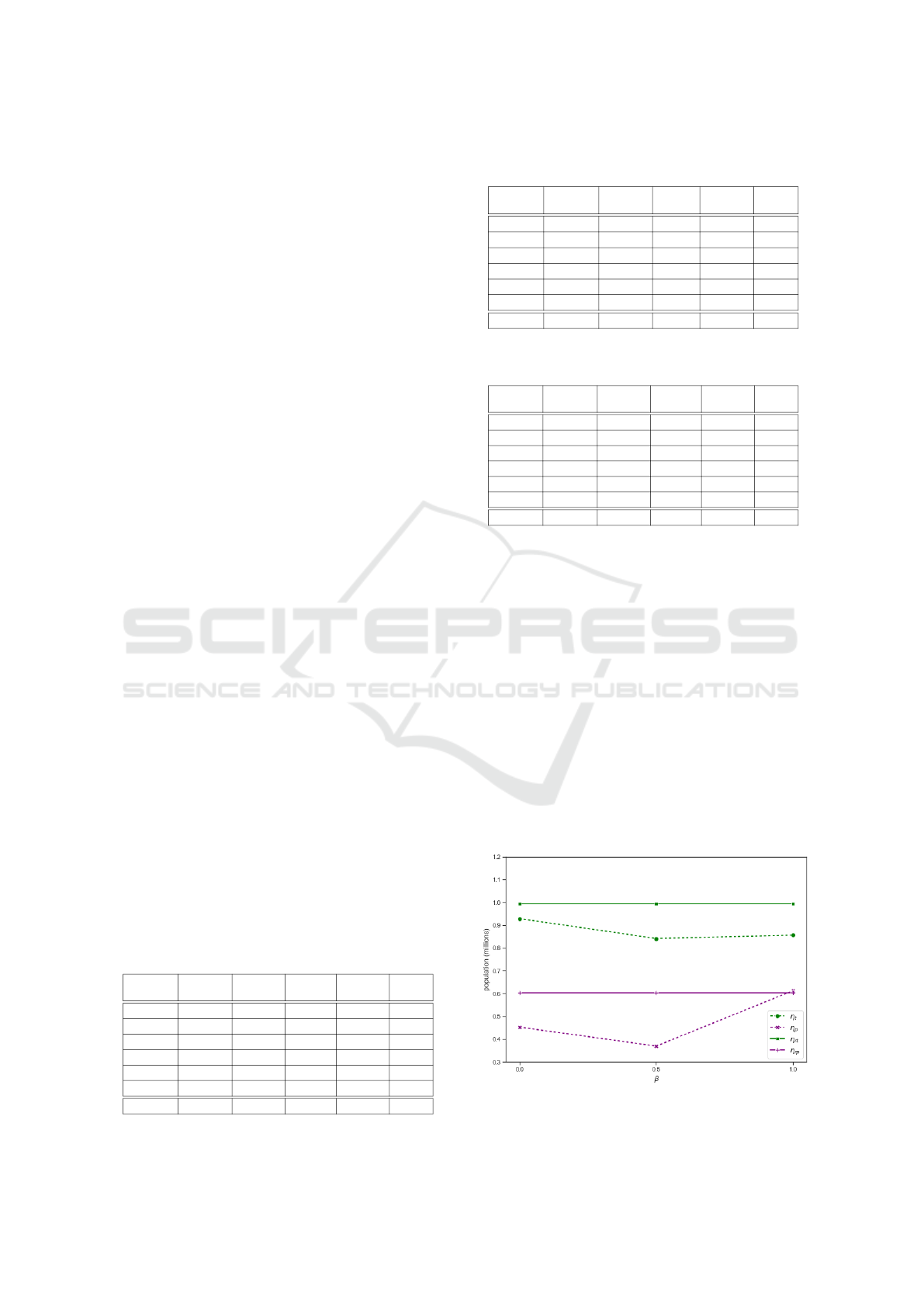
values of the parameter β ∈ {0,0.5,1}.
4.3 Results
Tables 3-5 and Figure 4-6 display the results obtained
for each value of β ∈ {0.0,0.5, 1.0}. Recall that β
determines which healthcare services are a priority
to minimize the overall population surplus concern-
ing its medical offer. When β = 0.0, the emphasis
is solely on the primary healthcare level (β = 1.0).
When β = 1.0, the focus is on the secondary level, Fi-
nally, with β = 0.5, there is a balanced consideration
of both the total exceeding of both the primary and
secondary healthcare level.
Table 3 (β = 1) shows a reduction of 25.07% in
the largest excess of population over resources at the
primary healthcare level, decreasing from 603,757 to
452,258 people. In addition to this reduction, Ta-
ble 3 also indicates a decrease in the largest abso-
lute deviation as a percentage among districts regard-
ing the excess of population over resources at the pri-
mary healthcare level, from 92.15% to 83.13%. These
positive results are also partially observed at the sec-
ondary healthcare level, where the largest excess of
population over resources is reduced from 992,968 to
928,651 people (6.48%). However, the largest mean
absolute deviations as a percentage at the secondary
healthcare level increases from 23.23% to 32.31%,
leading to a reduction in the homogeneity of resource
distribution among districts.
In Table 5 (β = 0), we observe that the largest ex-
cess of the population over resources is reduced from
992,968 to 856,352 people (13.76%), and the largest
mean absolute deviation as a percentage among dis-
tricts is reduced from 23.23% to 13.38%. Nonethe-
less, these improvements come with a slight increase
in the largest excess of population over resources at
the primary healthcare level, rising from 603,753 to
612,519 people (1.45%), and an increase in the largest
mean absolute deviation as a percentage of 2.79%
(from 92.15% to 94.94%). This results in a deteri-
oration of the homogeneity in the distribution of re-
sources at the primary healthcare level.
Table 3: Composition of healthcare services in the
Metropolitan region of Santiago with β = 1.
District Population
Secondary
Capacity
Primary
Capacity
Secondary
Excess
Primary
Excess
Central 1,341,551 486,207 889,183 855,344 452,368
East 953,995 109,457 509,841 844,538 444,154
South 1,402,653 611,261 1,147,692 791,392 254,961
West 1,011,354 412,323 958,347 599,031 53,007
South-East 1,179,203 363,570 822,923 815,633 356,280
North 1,152,938 224,287 828,473 928,651 324,465
MAPD 19.52% 70.24% 40.68% 32.31% 83.13%
Table 4: Composition of healthcare services in the
metropolitan region of Santiago with β = 0.5.
District Population
Secondary
Capacity
Primary
Capacity
Secondary
Excess
Primary
Excess
Central 1,281,187 492,453 950,799 788,734 330,388
East 1,066,277 303,286 696,645 762,991 369,632
South 1,199,911 395,434 967,622 804,477 232,289
West 1,239,516 427,337 983,002 812,179 256,514
South-East 1,094,376 269,316 735,223 825,060 359,153
North 1,160,427 319,279 823,168 841,148 337,259
MAPD 9.17% 33.9% 18.94% 5.31% 26.07%
Table 5: Composition of healthcare services in the
metropolitan region of Santiago with β = 0.
District Population
Secondary
Capacity
Primary
Capacity
Secondary
Excess
Primary
Excess
Central 1,254,276 397,924 641,757 856,352 612,519
East 1,130,793 303,286 665,257 827,507 465,536
South 1,354,982 519,389 1,233,226 835,593 121,756
West 1,202,137 504,195 1,117,597 697,942 84,540
South-East 1,152,682 363,570 793,088 789,112 359,594
North 946,824 118,741 705,534 828,083 241,290
MAPD 19.3% 67.72% 43.50% 13.38% 94.94%
Results in Table 4 (β = 0.5) equate the importance
of the primary and secondary healthcare level in the
objective function. This results in the decrease of both
maximum absolute deviations as a percentaje. For
the case of the secondary healthcare level, this goes
from 23.23% to 5.31%, while at the primary health-
care level, it decreases from 92.15% to 26.07%. On
the other hand, considering the largest excess of popu-
lation over available resources compared to the initial
case, these decrease from 992,968 to 841,148, result-
ing in a reduction of 15.28% for secondary healthcare
level; meanwhile, the excess for primary healthcare
level goes from 603,757 to 369,632, decreasing by
38.77%.
Table 6 and Figure 3 summarize the results for
each value of β. In the case that the decision maker
is more interested in minimizing the largest excess in
Figure 3: Capacity-demand match at primary and secondary
levels according to different values of β.
Balancing Resources and Demand: A Bi-Objective Mixed-Integer Programming Approach of Healthcare Districts in Chile
347