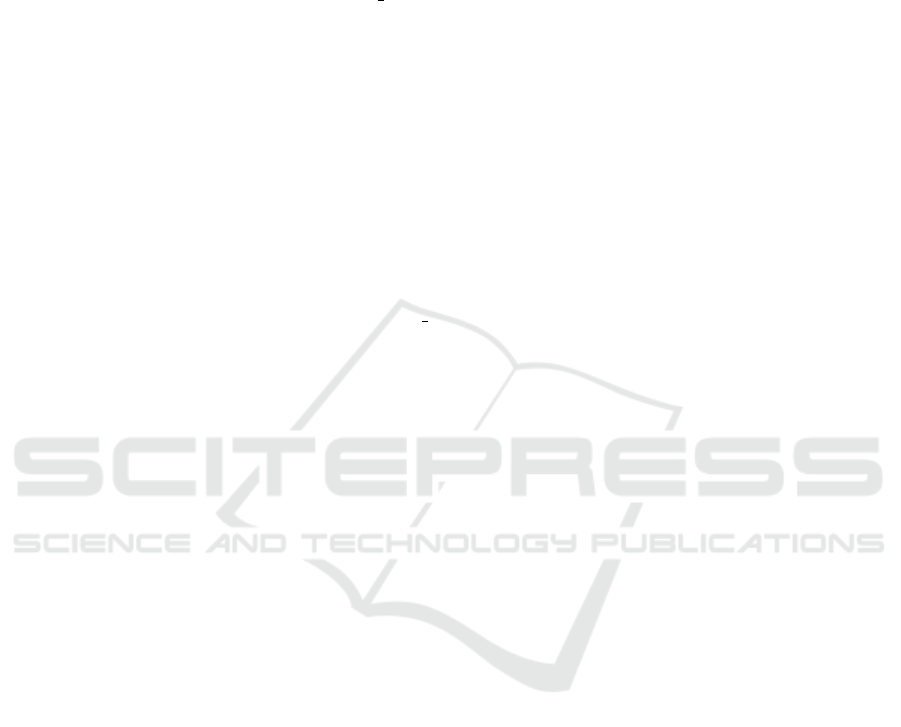
ACKNOWLEDGEMENTS
This research has been developed as part of a
Ph.D. program in collaboration with the Univer-
sity of Pisa and the National Institute for Nu-
clear Physics (INFN). Research partly supported
by: Artificial Intelligence in Medicine (next AIM,
https://www.pi.infn.it/aim) project, funded by INFN-
CSN5; FAIR-AIM project funded by Tuscany Gov-
ernment (POR FSE 2014-2020); PNRR - M4C2 -
Partenariato Esteso ”FAIR - Future Artificial Intel-
ligence Research” - Spoke 8, and PNRR - M4C2
- Centro Nazionale ”ICSC – Centro Nazionale di
Ricerca in High Performance Computing, Big Data
and Quantum Computing” - Spoke 8, funded by
the European Commission under the NextGeneration
EU programme; the Italian Ministry of Health Grant
RC and 5×1000 Health Research; AIMS2-Trials,
http://aims-2-trials.eu; the European Union NextGen-
erationEU through the Italian Ministry of University
and Research under PNRR M4C2-I1.3 Project PE
00000019 ”HEAL ITALIA” to Maria Evelina Fan-
tacci and Arman Zafaranchi CUP I53C22001440006.
The views and opinions expressed are those of the au-
thors only and do not necessarily reflect those of the
European Union or the European Commission Nei-
ther the European Union nor the European Commis-
sion can be held responsible for them.
REFERENCES
Barca, P., Giannelli, M., Fantacci, M. E., and Caramella,
D. (2018a). Computed tomography imaging with the
adaptive statistical iterative reconstruction (asir) algo-
rithm: dependence of image quality on the blending
level of reconstruction. Australasian physical & engi-
neering sciences in medicine, 41:463–473.
Barca, P., Palmas, F., Fantacci, M. E., and Caramella, D.
(2018b). Evaluation of the adaptive statistical iter-
ative reconstruction algorithm in chest ct (computed
tomography).
Beister, M., Kolditz, D., and Kalender, W. A. (2012). It-
erative reconstruction methods in x-ray ct. Physica
medica, 28(2):94–108.
Burgess, A. (1994). Statistically defined backgrounds:
performance of a modified nonprewhitening observer
model. JOSA A, 11(4):1237–1242.
Cao, C.-F., Ma, K.-L., Shan, H., Liu, T.-F., Zhao, S.-Q.,
Wan, Y., and Wang, H.-Q. (2022). Ct scans and cancer
risks: A systematic review and dose-response meta-
analysis. BMC cancer, 22(1):1238.
Caramella, D., Fantacci, M. E., Palmas, F., Barca, P., et al.
(2018). Evaluation of the adaptive statistical itera-
tive reconstruction algorithm in chest ct (computed
tomography)-a preliminary study toward its employ-
ment in low dose applications, also in conjunction
with cad (computer aided detection). In Proceedings
of the 11th International Joint Conference on Biomed-
ical Engineering Systems and Technologies-Volume 5:
AI4Health, volume 5, pages 688–694.
Dodge, C. T., Tamm, E. P., Cody, D. D., Liu, X., Jensen,
C. T., Wei, W., Kundra, V., and Rong, X. J. (2016).
Performance evaluation of iterative reconstruction al-
gorithms for achieving ct radiation dose reduction—a
phantom study. Journal of applied clinical medical
physics, 17(2):511–531.
Forte, G. C., Altmayer, S., Silva, R. F., Stefani, M. T., Liber-
mann, L. L., Cavion, C. C., Youssef, A., Forghani, R.,
King, J., Mohamed, T.-L., et al. (2022). Deep learning
algorithms for diagnosis of lung cancer: a systematic
review and meta-analysis. Cancers, 14(16):3856.
Kennedy, K., Hulbert, A., Pasquinelli, M., and Feldman,
L. E. (2022). Impact of ct screening in lung cancer:
Scientific evidence and literature review. In Seminars
in Oncology. Elsevier.
Mail, T. B. (2013). Catphan® 500 and 600 m anual. The
Phantom Laboratory.
Manickavasagam, R., Selvan, S., and Selvan, M. (2022).
Cad system for lung nodule detection using deep
learning with cnn. Medical & Biological Engineering
& Computing, 60(1):221–228.
Muti, G., Riga, S., Berta, L., Curto, D., De Mattia, C.,
Felisi, M., Rizzetto, F., Torresin, A., Vanzulli, A.,
and Colombo, P. E. (2023). Performance of three
model-based iterative reconstruction algorithms using
a ct task-based image quality metric. arXiv preprint
arXiv:2301.08691.
Raju, S., Ghosh, S., and Mehta, A. C. (2017). Chest ct
signs in pulmonary disease: a pictorial review. Chest,
151(6):1356–1374.
Samei, E., Bakalyar, D., Boedeker, K. L., Brady, S., Fan, J.,
Leng, S., Myers, K. J., Popescu, L. M., Ramirez Gi-
raldo, J. C., Ranallo, F., et al. (2019). Performance
evaluation of computed tomography systems: sum-
mary of aapm task group 233. Medical physics,
46(11):e735–e756.
Smith-Bindman, R., Wang, Y., Chu, P., Chung, R., Einstein,
A. J., Balcombe, J., Cocker, M., Das, M., Delman,
B. N., Flynn, M., et al. (2019). International variation
in radiation dose for computed tomography examina-
tions: prospective cohort study. Bmj, 364.
Solomon, J., Wilson, J., and Samei, E. (2015). Character-
istic image quality of a third generation dual-source
mdct scanner: noise, resolution, and detectability.
Medical physics, 42(8):4941–4953.
Solomon, J. B. (2018). Performance evaluation of com-
puted tomography systems. resources: Aapm tg-233.
Vennart, W. (1997). Icru report 54: Medical imaging-the as-
sessment of image quality-isbn 0-913394-53-x. april
1996, maryland, usa. Radiography, 3(3):243–244.
Yeh, D.-M., Tsai, H.-Y., Tyan, Y.-S., Chang, Y.-C., Pan, L.-
K., and Chen, T.-R. (2016). The population effective
dose of medical computed tomography examinations
in taiwan for 2013. PLoS One, 11(10):e0165526.
BIOIMAGING 2024 - 11th International Conference on Bioimaging
296