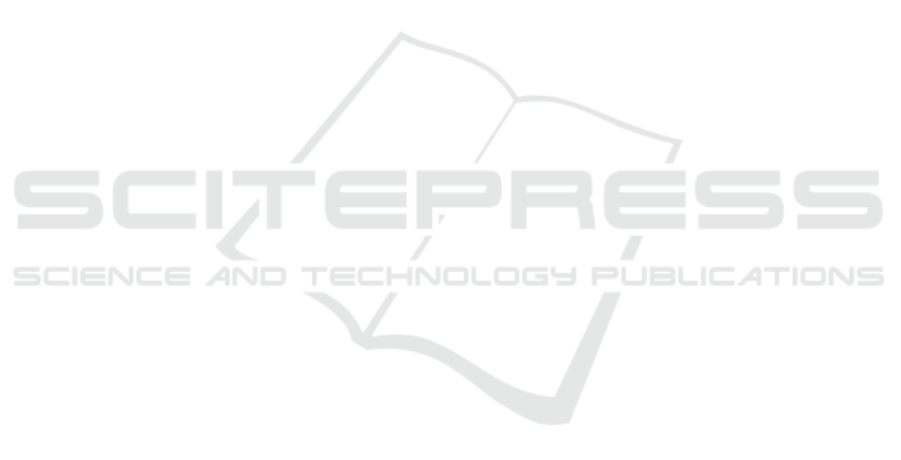
shops, Eindhoven, The Netherlands, September 7-8,
2014, Revised Papers 12, pages 3–15. Springer.
Jo
ˇ
st, G., Heri
ˇ
cko, M., and Polan
ˇ
ci
ˇ
c, G. (2019). Theo-
retical foundations and implementation of business
process diagrams’ complexity management technique
based on highlights. Software & Systems Modeling,
18:1079–1095.
Kluza, K. and Nalepa, G. J. (2012). Proposal of square met-
rics for measuring business process model complexity.
In 2012 Federated Conference on Computer Science
and Information Systems (FedCSIS), pages 919–922.
IEEE.
Kurz, M., Schmidt, W., Fleischmann, A., and Lederer, M.
(2015). Leveraging cmmn for acm: examining the
applicability of a new omg standard for adaptive case
management. In Proceedings of the 7th international
conference on subject-oriented business process man-
agement, pages 1–9.
Lenz, R., Peleg, M., and Reichert, M. (2012). Healthcare
process support: achievements, challenges, current re-
search. International Journal of Knowledge-Based
Organizations (IJKBO), 2(4).
Lenz, R. and Reichert, M. (2007). It support for healthcare
processes–premises, challenges, perspectives. Data &
Knowledge Engineering, 61(1):39–58.
Mans, R. S., Van der Aalst, W. M., and Vanwersch, R. J.
(2015). Process mining in healthcare: evaluating and
exploiting operational healthcare processes. Springer.
Marin, M. A. Complexity metrics for the case management
modeling notation (cmmn).
Mei, J., Li, J., Yu, Y., Li, X., Liu, H., and Xie, G. (2014).
Embracing case management for computerization of
care pathways. In MIE, pages 3–7.
Mulyar, N., Pesic, M., Van Der Aalst, W. M., and Peleg, M.
(2008a). Declarative and procedural approaches for
modelling clinical guidelines: addressing flexibility
issues. In Business Process Management Workshops:
BPM 2007 International Workshops, BPI, BPD, CBP,
ProHealth, RefMod, semantics4ws, Brisbane, Aus-
tralia, September 24, 2007, Revised Selected Papers
5, pages 335–346. Springer.
Mulyar, N., van der Aalst, W. M., and Russell, N. (2008b).
Process flexibility patterns.
Neskovic, S. and Kirchner, K. (2016). Using context infor-
mation and cmmn to model knowledge-. pages 17–21.
OMG (2011). Business Process Model and Notation . (Jan-
uary):538.
OMG (2016). Case Management Model and Notation 1.1
Beta. (March):124.
OMG Healthcare Domain Taskforce (2020). The BPM+
Field Guide to Shareable Clinical Pathways. page 125.
Pufahl, L., Zerbato, F., Weber, B., and Weber, I. (2022).
Bpmn in healthcare: Challenges and best practices.
Information Systems, 107:102013.
Rojo, M. G., Rol
´
on, E., Calahorra, L., Garc
´
ıa, F.
´
O.,
S
´
anchez, R. P., Ruiz, F., Ballester, N., Armenteros,
M., Rodr
´
ıguez, T., and Espartero, R. M. (2008). Im-
plementation of the business process modelling nota-
tion (bpmn) in the modelling of anatomic pathology
processes. In Diagnostic pathology, volume 3, pages
1–4. BioMed Central.
Rol
´
on, E., Aguilar, E., Garc
´
ıa, F., Ruiz, F., Piattini, M.,
Calahorra, L., et al. (2008). Process modeling of the
health sector using bpmn: a case study. In Proceed-
ings of First International Conference on Health In-
formatics, HEALTHINF 2008, volume 2, pages 173–
8.
Routis, I., Bardaki, C., Nikolaidou, M., Dede, G., and
Anagnostopoulos, D. (2023). Exploring cmmn ap-
plicability to knowledge-intensive process modeling:
An empirical evaluation by modelers. Knowledge and
Process Management, 30(1):33–54.
Routis, I., Nikolaidou, M., and Anagnostopoulos, D.
(2020). Empirical evaluation of CMMN models: a
collaborative process case study. Software and Sys-
tems Modeling, 19(6):1395–1413.
Rzepi
´
nski, T. M. (2007). The structure of diagnosis in
medicine: Introduction to interrogative characteris-
tics. Theoretical Medicine and Bioethics, 28:63–81.
S
´
anchez-Gonz
´
alez, L., Ruiz, F., Garc
´
ıa, F., and Cardoso, J.
(2011). Towards thresholds of control flow complex-
ity measures for bpmn models. In Proceedings of the
2011 ACM symposium on Applied computing, pages
1445–1450.
Taj, M., Brenner, M., Sulaiman, Z., and Pandian, V. (2022).
Sepsis protocols to reduce mortality in resource-
restricted settings: A systematic review. Intensive and
Critical Care Nursing, page 103255.
Von Rosing, M., White, S. A., Cummins, F., and De Man,
H. (2014). Business process model and notation-
BPMN. The Complete Business Process Handbook:
Body of Knowledge from Process Modeling to BPM,
1(January):429–453.
Wiemuth, M., Junger, D., Leitritz, M., Neumann, J., Neu-
muth, T., and Burgert, O. (2017). Application fields
for the new object management group (omg) standards
case management model and notation (cmmn) and de-
cision management notation (dmn) in the periopera-
tive field. International journal of computer assisted
radiology and surgery, 12:1439–1449.
Ye, J., Sun, S., Song, W., and Wen, L. (2008). Formal se-
mantics of bpmn process models using yawl. In 2008
Second International Symposium on Intelligent Infor-
mation Technology Application, volume 2, pages 70–
74. IEEE.
Zensen, A. and Kuster, J. (2018). A comparison of flex-
ible BPMN and CMMN in practice: A case study
on component release processes. Proceedings - 2018
IEEE 22nd International Enterprise Distributed Ob-
ject Computing Conference, EDOC 2018, pages 105–
114.
HEALTHINF 2024 - 17th International Conference on Health Informatics
528