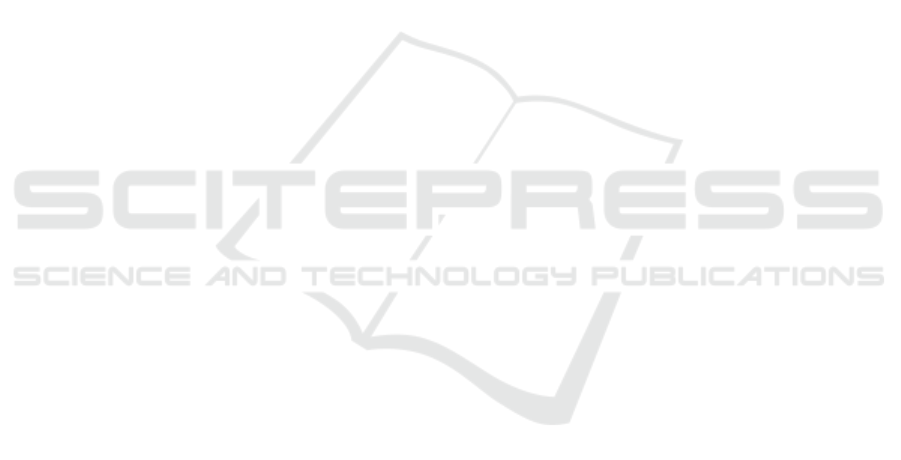
ACKNOWLEDGEMENTS
This work was supported by the National Sci-
ence Foundation under Award NSF FRR CAREER
2144348.
REFERENCES
Aslan, S., Liu, X., Wu, Q., Mass, P., Loke, Y.-H., Hib-
ino, N., Olivieri, L., and Krieger, A. (2022). Virtual
planning and simulation of coarctation repair in hy-
poplastic aortic arches: is fixing the coarctation alone
enough? In BIOINFORMATICS, pages 138–143.
Azadani, A. N., Chitsaz, S., Matthews, P. B., Jaussaud, N.,
Leung, J., Wisneski, A., Ge, L., and Tseng, E. E.
(2012). Biomechanical comparison of human pul-
monary and aortic roots. European journal of cardio-
thoracic surgery, 41(5):1111–1116.
Boekhoven, R. W., Peters, M. F., Rutten, M. C., van Sam-
beek, M. R., van de Vosse, F. N., and Lopata, R. G.
(2016). Inflation and bi-axial tensile testing of healthy
porcine
´
acarotid arteries. Ultrasound in Medicine &
Biology, 42(2):574–585.
Caimi, A., Sturla, F., Pluchinotta, F. R., Giugno, L., Secchi,
F., Votta, E., Carminati, M., and Redaelli, A. (2018).
Prediction of stenting related adverse events through
patient-specific finite element modelling. Journal of
Biomechanics, 79:135–146.
Fegan, K. L., Green, N. C., Britton, M. M., Iqbal, A. J.,
and Thomas-Seale, L. E. (2022). Design and simula-
tion of the biomechanics of multi-layered composite
poly (vinyl alcohol) coronary artery grafts. Frontiers
in cardiovascular medicine, 9:883179.
Fung, Y. (1967). Elasticity of soft tissues in simple elon-
gation. American Journal of Physiology-Legacy Con-
tent, 213(6):1532–1544.
Gasser, T. C., Ogden, R. W., and Holzapfel, G. A. (2006).
Hyperelastic modelling of arterial layers with dis-
tributed collagen fibre orientations. Journal of the
royal society interface, 3(6):15–35.
Hayashi, K. (2003). Mechanical properties of soft tissues
and arterial walls. In Biomechanics of soft tissue in
cardiovascular systems, pages 15–64. Springer.
He, R., Zhao, L., Silberschmidt, V. V., Liu, Y., and Vogt,
F. (2019). Finite element modelling of stent deploy-
ment in a patient-specific coronary artery. Procedia
Structural Integrity, 15:28–32.
Hoffman, A. H., Teng, Z., Zheng, J., Wu, Z., Woodard,
P. K., Billiar, K. L., Wang, L., and Tang, D. (2017).
Stiffness properties of adventitia, media, and full
thickness human atherosclerotic carotid arteries in
the axial and circumferential directions. Journal of
biomechanical engineering, 139(12):124501.
Humphrey, J. D. (1995). Mechanics of the arterial wall: re-
view and directions. Critical Reviews™ in Biomedical
Engineering, 23(1-2).
Lally, C., Reid, A., and Prendergast, P. J. (2004). Elastic
behavior of porcine coronary artery tissue under uni-
axial and equibiaxial tension. Annals of biomedical
engineering, 32:1355–1364.
Lashkarinia, S. S., Coban, G., Kose, B., Salihoglu, E.,
and Pekkan, K. (2021). Computational modeling of
vascular growth in patient-specific pulmonary arte-
rial patch reconstructions. Journal of Biomechanics,
117:110274.
Lashkarinia, S. S., Piskin, S., Bozkaya, T. A., Salihoglu, E.,
Yerebakan, C., and Pekkan, K. (2018). Computational
pre-surgical planning of arterial patch reconstruction:
parametric limits and in vitro validation. Annals of
biomedical engineering, 46:1292–1308.
Liu, X., Hibino, N., Loke, Y.-H., Kim, B., Mass, P., Fuge,
M. D., Olivieri, L., and Krieger, A. (2022). Surgical
planning and optimization of patient-specific fontan
grafts with uncertain post-operative boundary condi-
tions and anastomosis displacement. IEEE Transac-
tions on Biomedical Engineering, 69(11):3472–3483.
Mueller-Graf, F., Merz, J., Bandorf, T., Albus, C. F.,
Henkel, M., Krukewitt, L., Kuehn, V., Reuter, S., Voll-
mar, B., Pulletz, S., et al. (2021). Correlation of
pulse wave transit time with pulmonary artery pres-
sure in a porcine model of pulmonary hypertension.
Biomedicines, 9(9):1212.
Ogden, R. W. (1972). Large deformation isotropic
elasticity–on the correlation of theory and experiment
for incompressible rubberlike solids. Proceedings of
the Royal Society of London. A. Mathematical and
Physical Sciences, 326(1567):565–584.
Pillalamarri, N. R., Patnaik, S. S., Piskin, S., Gueldner, P.,
and Finol, E. A. (2021). Ex vivo regional mechanical
characterization of porcine pulmonary arteries. Exper-
imental mechanics, 61:285–303.
Pourmodheji, R., Jiang, Z., Tossas-Betancourt, C.,
Figueroa, C. A., Baek, S., and Lee, L.-C. (2021). In-
verse modeling framework for characterizing patient-
specific microstructural changes in the pulmonary ar-
teries. journal of the mechanical behavior of biomed-
ical materials, 119:104448.
Razaghi, R., Karimi, A., and Taheri, R. A. (2018). Patient-
specific finite element model of coronary artery stent-
ing. Current pharmaceutical design, 24(37):4492–
4502.
Sanders, S. N., Lopata, R. G., van Breemen, L. C., van de
Vosse, F. N., and Rutten, M. C. (2020). A novel
technique for the assessment of mechanical proper-
ties of vascular tissue. Biomechanics and Modeling
in Mechanobiology, 19:1585–1594.
Tian, L. and Chester, N. C. (2012). In vivo and in vitro
measurements of pulmonary arterial stiffness: a brief
review. Pulmonary circulation, 2(4):505–517.
Computational Modeling of Arterial Walls: Evaluating Model Complexity and the Influence of Model Parameters on Deformation Outcomes
461