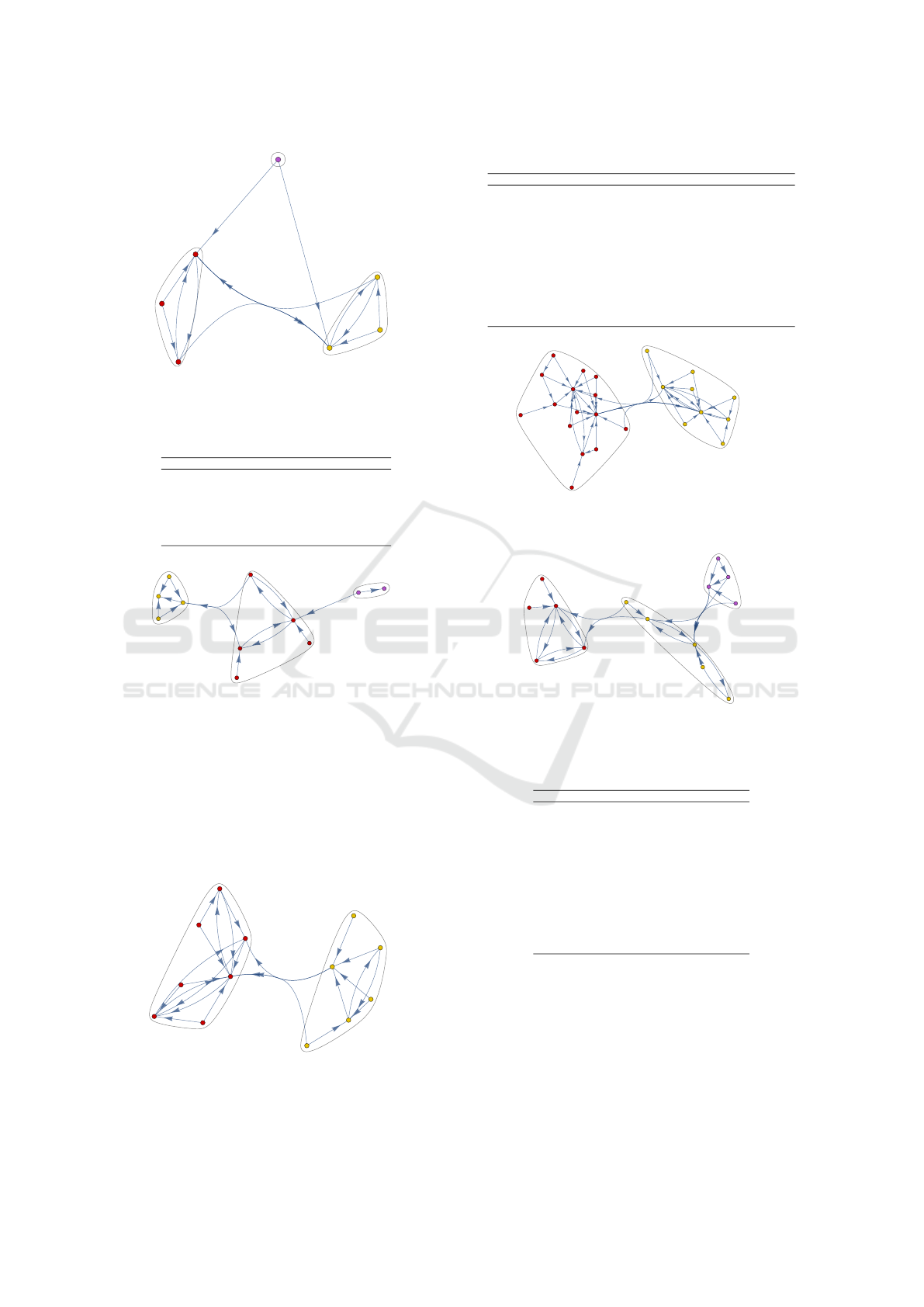
In[9]:=
stelle Gemeinschaft graphisch dar
CommunityGraphPlot[atcGraph]
Out[9]=
J01A
J01C
J01F
J01D
J01X
J01E
J01M
2
Figure 7: Prescription switch between drug groups within
an antibiotic therapy 2021 with clusters.
Table 5: ATC 4 drug groups in transition graphs.
ATC 4 drug group
J01A tetracyclines
J01C beta-lactam antibacterials, penicillins
J01D other beta-lactam antibacterials
J01E sulfonamides and trimethoprim
J01F macrolides, lincosamides and streptogramins
J01M quinolone antibacterials
J01X other antibacterials
In[12]:=
stelle Gemeinschaft graphisch dar
CommunityGraphPlot[atcGraph]
Out[12]=
J01CA
J01FA
J01MA
J01CR
J01DC
J01AA
J01DD
J01EE
J01XX
J01FF
J01XE
2
Figure 8: Prescription switch between drug groups within
an antibiotic therapy 2018 with clusters.
was changed to another more frequently than another
was changed to this group. Due to the extended spec-
trum of action of J01CA, the replacement may be mo-
tivated by theapeutic safety reasons, cf. (Holstiege
et al., 2022), (Langford et al., 2021), (Kern et al.,
2006) and (Filippini et al., 2006). Another possibil-
ity could be a change due to delivery difficulties.
In[ ]:=
Eigensystem
Eigensystem[ad2]
Out[]=
2., -1., - 1., - 1., 1., 1.11022 × 10
-16
, -5.55112 × 10
-17
, 0., 0., 0., 0., 0., 0.,
{0.285714, 0.285714, 0.285714, 0.285714, 0.285714, 0.285714,
0.285714, 0.285714, 0.142857, 0.285714, 0.285714, 0.285714, 0.285714},
{0.229416, 0.229416, -0.458831, 0.229416, 0.229416, 0.229416, 0.573539,
-0.114708, - 0.229416, 0.229416, -0.114708, 0.229416, - 0.114708},
{0.229416, 0.229416, -0.458831, 0.229416, 0.229416, 0.229416, 0.573539,
-0.114708, - 0.229416, 0.229416, -0.114708, 0.229416, - 0.114708},
{-0.229416, - 0.229416, 0.458831, -0.229416, - 0.229416, -0.229416,
-0.573539, 0.114708, 0.229416, - 0.229416, 0.114708, - 0.229416, 0.114708},
5.97306 × 10
-17
, -4.61881 × 10
-17
, 6.15841 × 10
-17
, -4.61881 × 10
-17
, 5.97306 × 10
-17
,
-4.61881 × 10
-17
, 0.5, 0.5, -4.65664 × 10
-17
, -4.61881 × 10
-17
, 0.5, 5.97306 × 10
-17
, 0.5,
1.84183 × 10
-16
, -3.70074 × 10
-17
, 3.70074 × 10
-17
, 3.70074 × 10
-17
,
1.84183 × 10
-16
, -3.70074 × 10
-17
, 0.666667, 3.70074 × 10
-17
, -0.333333,
-3.70074 × 10
-17
, 3.70074 × 10
-17
, 0.666667, -9.24072 × 10
-17
,
1.4457 × 10
-16
, 9.45405 × 10
-18
, -9.45405 × 10
-18
, -9.45405 × 10
-18
, 1.4457 × 10
-16
,
9.45405 × 10
-18
, -0.574192, 4.13281 × 10
-17
, 0.744501, -4.13281 × 10
-17
, 4.13281 × 10
-17
,
0.340618, 5.27262 × 10
-17
, {1., 0., 0., 0., 0., 0., 0., 0., 0., 0., 0., 0., 0.},
{0., 0., 0., 0., 1., 0., 0., 0., 0., 0., 0., 0., 0.},
{0., 0., 0., 0., 0., 0., 1., 0., 0., 0., 0., 0., 0.},
{0., 0., 0., 0., 0., 0., 0., 0., 1., 0., 0., 0., 0.},
{0., 0., 0., 0., 0., 0., 0., 0., 0., 0., 0., 1., 0.},
{0., 0., 0., 0., 0., 0., 0., 0., 0., 0., 0., 0., 1.}
In[14]:=
stelle Gemeinschaft graphisch dar
CommunityGraphPlot[atcGraph]
Out[14]=
J01AA
J01FA
J01CA
J01CR
J01CE
J01DC
J01DD
J01EE
J01EA
J01XX
J01MA
J01FF
J01XE
2
Figure 9: Prescription switch between drug groups within
an antibiotic therapy 2021 with clusters.
Table 6: ATC 5 drug groups in transition graphs.
ATC 5 drug group
J01AA tetracyclines
J01CA penicillins with extended spectrum
J01CE beta-lactamase sensitive penicillins
J01CR combinations of penicillins, incl. beta-lactamase inhibitors
J01DC second-generation cephalosporins
J01DD third-generation cephalosporins
J01EA trimethoprim and derivatives
J01EE combinations of sulfonamides and trimethoprim, incl. derivatives
J01FA macrolides
J01FF lincosamides
J01MA fluoroquinolones
J01XE nitrofuran derivatives
J01XX other antibacterials
In[ ]:=
Eigensystem
Eigensystem[ad2]
Out[]=
{{2, -1, -1, -1, 1, 0, 0, 0, 0, 0, 0, 0, 0, 0, 0, 0, 0, 0, 0, 0, 0, 0, 0, 0},
{{2, 2, 2, 2, 2, 2, 2, 1, 2, 2, 2, 1, 2, 2, 2, 2, 2, 2, 2, 2, 2, 2, 2, 2},
{-1, 1, 0, -1, 0, 0, 0, 0, 0, -1, 1, 0, 1, 1, 1, 0, 0, 0, 0, 0, 0, 0, 1, 1},
{0, 0, 0, 0, 0, 0, 0, 0, 1, -1, 0, 0, -1, 0, 0, 0, 0, 0, 0, 1, 1, 1, 0, 0},
{-1, 0, 1, -1, 1, 1, 1, - 1, 0, 0, 0, -1, 1, 0, 0, 1, 1, 1, 1, 0, 0, 0, 0, 0},
{1, 0, 1, 1, 1, 1, 1, 1, 0, 0, 2, 1, 1, 0, 0, 3, 1, 1, 1, 0, 0, 0, 0, 0},
{0, 0, 0, 0, 0, 0, 0, 0, 0, 0, 0, 0, 0, 0, 0, 0, 0, 0, 0, 0, 0, 0, 0, 1},
{0, 0, 0, 0, 0, 0, 0, 0, 0, 0, 0, 0, 0, 0, 0, 0, 0, 0, 0, 0, 0, 0, 1, 0},
{0, 0, 0, 0, 0, 0, 0, 0, 0, 0, 0, 0, 0, 0, 0, 0, 0, 0, 0, 0, 0, 1, 0, 0},
{0, 0, 0, 0, 0, 0, 0, 0, 0, 0, 0, 0, 0, 0, 0, 0, 0, 0, 0, 0, 1, 0, 0, 0},
{0, 0, 0, 0, 0, 0, 0, 0, 0, 0, 0, 0, 0, 0, 0, 0, 0, 0, 0, 1, 0, 0, 0, 0},
{0, 0, 0, 0, 0, 0, 0, 0, 0, 0, 0, 0, 0, 0, 0, 0, 0, 0, 1, 0, 0, 0, 0, 0},
{0, 0, 0, 0, 0, 0, 0, 0, 0, 0, 0, 0, 0, 0, 0, 0, 0, 1, 0, 0, 0, 0, 0, 0},
{0, 0, 0, 0, 0, 0, 0, 0, 0, 0, 0, 0, 0, 0, 0, 0, 1, 0, 0, 0, 0, 0, 0, 0},
{0, 0, 0, 0, 0, 0, 0, 0, 0, 0, 0, 0, 0, 0, 0, 1, 0, 0, 0, 0, 0, 0, 0, 0},
{0, 0, 0, 0, 0, 0, 0, 0, 0, 0, 0, 0, 0, 1, 0, 0, 0, 0, 0, 0, 0, 0, 0, 0},
{0, 0, 0, 0, 0, 0, 0, 0, 0, 0, 0, 0, 1, 0, 0, 0, 0, 0, 0, 0, 0, 0, 0, 0},
{0, 0, 0, 0, 0, 0, 0, 0, 0, 0, 0, 1, 0, 0, 0, 0, 0, 0, 0, 0, 0, 0, 0, 0},
{0, 0, 0, 0, 0, 0, 0, 1, 0, 0, 0, 0, 0, 0, 0, 0, 0, 0, 0, 0, 0, 0, 0, 0},
{0, 0, 0, 0, 0, 0, 1, 0, 0, 0, 0, 0, 0, 0, 0, 0, 0, 0, 0, 0, 0, 0, 0, 0},
{0, 0, 0, 0, 0, 1, 0, 0, 0, 0, 0, 0, 0, 0, 0, 0, 0, 0, 0, 0, 0, 0, 0, 0},
{1, 0, 0, 0, 0, 0, 0, 0, 0, 0, 0, 0, 0, 0, 0, 0, 0, 0, 0, 0, 0, 0, 0, 0},
{0, 0, 0, 0, 0, 0, 0, 0, 0, 0, 0, 0, 0, 0, 0, 0, 0, 0, 0, 0, 0, 0, 0, 0},
{0, 0, 0, 0, 0, 0, 0, 0, 0, 0, 0, 0, 0, 0, 0, 0, 0, 0, 0, 0, 0, 0, 0, 0},
{0, 0, 0, 0, 0, 0, 0, 0, 0, 0, 0, 0, 0, 0, 0, 0, 0, 0, 0, 0, 0, 0, 0, 0}}}
In[16]:=
stelle Gemeinschaft graphisch dar
CommunityGraphPlot[atcGraph]
Out[16]=
J01AA02
J01DC02
J01FA10
J01CA04
J01CE02
J01CR02
J01CR04
J01DB05
J01MA02
J01XX01
J01DC04
J01DD08
J01DD13
J01EA01
J01EE01
J01FA01
J01FA06
J01FA09
J01FF01
J01MA01
J01MA06
J01MA12
J01XE01
J01XX07
2
Figure 10: Prescription switch between drug groups within
an antibiotic therapy 2018 with clusters.
In[ ]:=
Eigensystem
Eigensystem[ad2]
Out[]=
2., -1.41421, 1.41421, -1., -1., 9.7795 × 10
-17
, 0., 0., 0., 0., 0., 0., 0., 0.,
{-0.389867, -0.389867, -0.389867, -0.0974668, - 0.194934, - 0.194934, -0.2924,
-0.194934, -0.2193, -0.243667, -0.0974668, - 0.341134, - 0.2193, -0.231484},
{0., 0., 0., 0.3668, -0.518733, 0., 0.3668, 0., 0.290833, 0.107433, 0.3668,
-0.259367, 0.290833, -0.281617}, {0., 0., 0., 0.196808, 0.278329, 0.,
0.196808, 0., 0.434377, 0.335973, 0.196808, 0.139165, 0.434377, 0.54472},
0.267261, 0.267261, - 0.534522, 0.267261, -0.267261, -0.267261, 2.33274 × 10
-16
,
-0.267261, 3.63406 × 10
-16
, 0.267261, 0.267261, - 0.267261, 3.63406 × 10
-16
, -0.267261,
0.261341, -0.34631, 0.0849695, -0.34631, 0.34631, - 0.261341, 4.77034 × 10
-17
,
-0.261341, 0., -0.34631, -0.34631, -0.261341, 0., 0.34631,
0., 0., 0., 9.35299 × 10
-49
, -1.19937 × 10
-64
, 0., - 9.35299 × 10
-49
, 0., - 9.7795 × 10
-17
,
-9.56387 × 10
-33
, -1.22642 × 10
-48
, -9.56387 × 10
-33
, -9.7795 × 10
-17
, -1.,
{0., 0., 0., 0., 0., 1., 0., 0., 0., 0., 0., 0., 0., 0.},
{0., 0., 0., 0., 0., 0., 0., 1., 0., 0., 0., 0., 0., 0.},
{0., 0., 0., 0., 0., 0., 0., 0., 0., 0., 1., 0., 0., 0.},
{0., 0., 0., 0., 0., 0., 0., 0., 0., 0., 0., 1., 0., 0.},
{0., 0., 0., 0., 0., 0., 0., 0., 0., 0., 0., 0., 1., 0.},
{0., 0., 0., 0., 0., 0., 0., 0., 0., 0., 0., 0., 0., 1.},
{0., 0., 0., 0., 0., 0., 0., 0., 0., 0., 0., 0., 0., 0.},
{0., 0., 0., 0., 0., 0., 0., 0., 0., 0., 0., 0., 0., 0.}
In[18]:=
stelle Gemeinschaft graphisch dar
CommunityGraphPlot[atcGraph]
Out[18]=
J01CA04
J01CR02
J01FF01
J01CA08
J01XX01
J01CE02
J01DC02
J01DC04
J01DD13
J01EE01
J01EA01
J01FA10
J01MA02
J01XE01
2
Figure 11: Prescription switch between drug groups within
an antibiotic therapy 2021 with clusters.
Table 7: ATC 7 drug groups in transition graphs.
ATC 7 drug group
J01CA04 amoxicillin
J01CA08 pivmecillinam
J01CE02 phenoxymethylpenicillin
J01CR02 amoxicillin and beta-lactase inhibitors
J01DC02 cefuroxime
J01DC04 cefaclor
J01DD13 cefpodoxime
J01EA01 trimethoprim
J01EE01 sulfamethoxazole and trimethoprim
J01FA10 azithromycin
J01FF01 clindamycin
J01MA02 ciprofloxacin
J01XE01 nitrofurantoin
J01XX01 fosfomycin
In contrast to the ATC 4 and ATC 5 drug groups,
the graph for the active ingredients according to ATC
7 is divided into two community clusters in 2018 and
three in 2021. The active ingredients J01FF01 (clin-
damycin), J01CR02 (amoxicillin and beta-lactase in-
hibitors) and J01CA04 (amoxicillin) appear in pair-
wise and in both directions as the main versions of a
therapy change.
HEALTHINF 2024 - 17th International Conference on Health Informatics
416