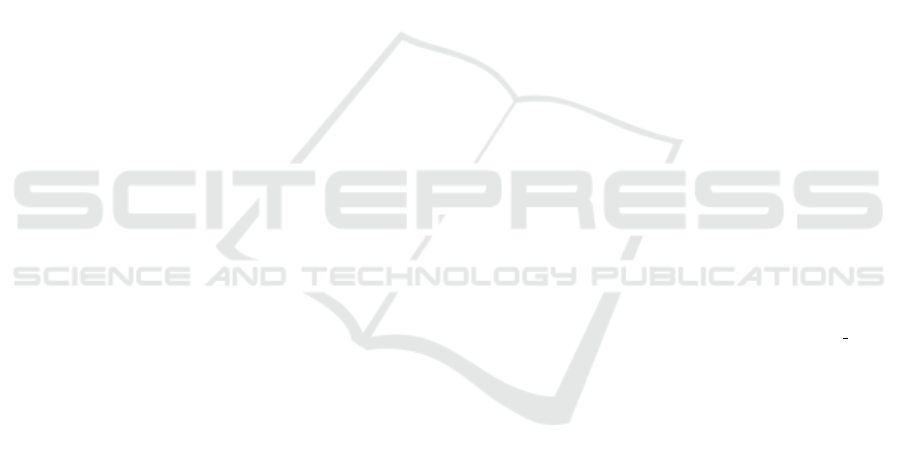
5 CONCLUSIONS AND
PERSPECTIVES
In this work, we propose a multi-compartment model
of postprandial D-xylose dynamics as a first step to-
wards a predictive model of intestinal glucose absorp-
tion. This model is based on three major parameters
representing the (linear) rates of gastric emptying, in-
testinal absorption and elimination, and models the
intestine as a succession of compartments, thus intro-
ducing a delay that models the intestinal transit. We
calibrated the model using a tailored dataset from sev-
eral minipig populations that underwent oral, intra-
venous or jejunal administration of D-xylose, as well
as intestinal resection. We studied the identifiability
and the sensitivity of its parameters.
This model presents good performances in terms
of goodness-of-fit, even with the data of jejunal injec-
tion, especially when compared with another model
where the gastric part is more complex but the intesti-
nal part is simplified, and which does not fit the data
of jejunal injection data as well. This suggests that the
chosen multi-compartment modeling of the intestine
is relevant, and emphasizes the important role of in-
testinal absorption. Furthermore, the model appeared
to be identifiable for all relevant parameters.
Finally, we also compared the rate of appearance
of D-xylose predicted by the model with the actual
rate of appearance of exogenous glucose (Ra
G
), that
is, glucose only coming from the meal and not from
kidney storage, for instance. These results are very in-
teresting as they corroborate that D-xylose could be a
valuable marker of intestinal absorption. It reinforces
the fact that our model is a good candidate to predict
Ra
G
, at least qualitatively.
Besides of experimental investigations, further
work is necessary to improve, or better take advan-
tage of, the ability of the model to predict Ra
G
. Also,
we plan to propose a simplified model of the glucose-
insulin regulation system based on the minimal-
model of (Bergman et al., 1979) with an accurate
D-xylose-based model of IGA. Finally, datasets on
humans that underwent glucose and D-xylose bolus
administrations could help translate this model to hu-
mans. In the long term, it is hoped that this model
could be applied to humans and could help in a medi-
cal setting to diagnose patients with abnormal intesti-
nal glucose absorption.
ACKNOWLEDGMENTS
This work was supported by project MIGAD (ANR-
21-CE45-0017) of the French National Research
Agency (ANR).
REFERENCES
Baud, G., Raverdy, V., Bonner, C., Daoudi, M., Caiazzo,
R., and Pattou, F. (2016). Sodium glucose trans-
port modulation in type 2 diabetes and gastric bypass
surgery. Surgery for Obesity and Related Diseases,
12(6):1206–1212. Diabetes Special Issue.
Bergman, M. et al. (2018). Lessons learned from the 1-hour
post-load glucose level during ogtt: Current screen-
ing recommendations for dysglycaemia should be re-
vised. Diabetes/metabolism research and reviews,
34(5):e2992.
Bergman, R. N., Ider, Y. Z., Bowden, C. R., and Cobelli, C.
(1979). Quantitative estimation of insulin sensitivity.
American Journal of Physiology-Endocrinology and
Metabolism, 236(6):E667.
Dalla Man, C., Camilleri, M., and Cobelli, C. (2006). A
system model of oral glucose absorption: Validation
on gold standard data. IEEE Transactions on Biomed-
ical Engineering, 53(12):2472–2478.
Elashoff, J. D., Reedy, T. J., and Meyer, J. H. (1982).
Analysis of gastric emptying data. Gastroenterology,
83(6):1306–1312.
Fujita, Y., Kojima, H., Hidaka, H., Fujimiya, M., Kashi-
wagi, A., and Kikkawa, R. (1998). Increased intestinal
glucose absorption and postprandial hyperglycaemia
at the early step of glucose intolerance in otsuka long-
evans tokushima fatty rats. Diabetologia, 41:1459–
1466.
Goutchtat, R., Marciniak, C., Caiazzo, R., Verkindt, H.,
Quenon, A., Rabier, T., Lapiere, S., Raverdy, V., Hu-
bert, T., and Pattou, F. (2022). 1361-p: D-xylose test
as a biomarker for glucose intestinal absorption in hu-
mans and minipigs. Diabetes, 71(Supplement 1).
Hansen, N. (2023). The cma evolution strategy: A tutorial.
Raue, A., Kreutz, C., Maiwald, T., Bachmann, J., Schilling,
M., Klingm
¨
uller, U., and Timmer, J. (2009). Struc-
tural and practical identifiability analysis of partially
observed dynamical models by exploiting the profile
likelihood. Bioinformatics, 25(15):1923–1929.
Salinari, S., Bertuzzi, A., and Mingrone, G. (2011). Intesti-
nal transit of a glucose bolus and incretin kinetics: a
mathematical model with application to the oral glu-
cose tolerance test. American Journal of Physiology-
Endocrinology and Metabolism, 300(6):E955–E965.
Sobo
´
l, I. (1993). Sensitivity estimates for nonlinear mathe-
matical models. Math. Model. Comput. Exp., 1:407.
Toffolo, G., Basu, R., Dalla Man, C., Rizza, R., and
Cobelli, C. (2006). Assessment of postprandial
glucose metabolism: conventional dual-vs. triple-
tracer method. American Journal of Physiology-
Endocrinology And Metabolism, 291(4):E800–E806.
Tric
`
o, D., Mengozzi, A., Frascerra, S., Scozzaro, M. T.,
Mari, A., and Natali, A. (2019). Intestinal glu-
cose absorption is a key determinant of 1-hour post-
load plasma glucose levels in nondiabetic subjects.
The Journal of Clinical Endocrinology & Metabolism,
104(6):2131–2139.
Modeling Intestinal Glucose Absorption from D-Xylose Data
445