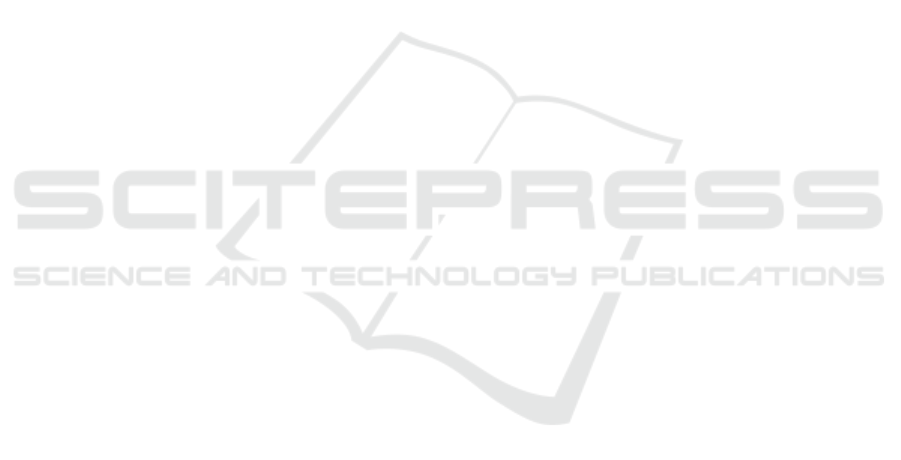
Consent) is inevitable. The up-to-date review of e-
consent by (Skelton et al., 2020) presented a work-
flow of the informed consent processes, but this was
more theoretical than practical and did not consider
evolving privacy mandates and the longitudinal na-
ture of research data and evolving research demands.
In this paper we explore the complexities of aligning
e-Consent mechanisms with stringent global data pro-
tection regulations, notably GDPR.
Through the exploration of the biomedical re-
search registry Australasian Diabetes Data Network’s
(ADDN)’s consent app, we illuminate practical im-
plementations that adhere to such regulations while
enhancing the patient experience.
We also presented an improved GDPR-compliant
consent workflow in biomedical research settings.
This provides guidance to other biomedical research
registries attempting to navigate the complexities of
GDPR-compliant e-consent implementations.
We note that the mobile application is undergoing
advanced testing and will be rolled out as part of the
ADDN project in due course. The adoption, use and
feedback of the application will be explored in down-
stream work.
ACKNOWLEDGEMENTS
The authors would like to thank the ADDN project
partners for the ongoing work and support.
REFERENCES
Ausloos, J. (2012). The ’right to be forgotten’ - worth re-
membering? Computer Law and Security Review,
28(2):143–152.
Australian Government (2022). Privacy act review - discus-
sion paper. Webpage. Accessed on 2023-11-29.
Chimonas, S., Lipitz-Snyderman, A., Matsoukas, K., and
Kuperman, G. (2023). Electronic consent in clinical
care: an international scoping review. BMJ Health &
Care Informatics, 30(1).
Cohen, E., Byrom, B., Becher, A., J
¨
ornt
´
en-Karlsson, M.,
and Mackenzie, A. K. (2023). Comparative effective-
ness of econsent: Systematic review. Journal of med-
ical Internet research, 25.
Dankar, F. K., Gergely, M., Malin, B., Badji, R., Dankar,
S. K., and Shuaib, K. (2020). Dynamic-informed con-
sent: A potential solution for ethical dilemmas in pop-
ulation sequencing initiatives. Comput Struct Biotech-
nol J, 18:913–921.
de Man, Y., Wieland-Jorna, Y., Torensma, B., de Wit, K.,
Francke, A. L., Oosterveld-Vlug, M. G., and Verheij,
R. A. (2023). Opt-in and opt-out consent procedures
for the reuse of routinely recorded health data in scien-
tific research and their consequences for consent rate
and consent bias: Systematic review. Journal of med-
ical Internet research, 25.
De Sutter, E., Zac¸e, D., Boccia, S., Di Pietro, M. L., Geerts,
D., Borry, P., and Huys, I. (2020). Implementa-
tion of electronic informed consent in biomedical re-
search and stakeholders’ perspectives: systematic re-
view. Journal of medical Internet research, 22(10).
Del Carmen, M. G. and Joffe, S. (2005). Informed consent
for medical treatment and research: a review. The on-
cologist, 10(8):636–641.
Garrison, N. A., Sathe, N. A., Antommaria, A. H. M.,
Holm, I. A., Sanderson, S. C., Smith, M. E.,
McPheeters, M. L., and Clayton, E. W. (2016). A sys-
tematic literature review of individuals’ perspectives
on broad consent and data sharing in the united states.
Genetics in Medicine, 18(7):663–671.
Haas, M. A., Teare, H., Prictor, M., Ceregra, G., Vidgen,
M. E., Bunker, D., Kaye, J., and Boughtwood, T.
(2021). ’ctrl’: an online, dynamic consent and partic-
ipant engagement platform working towards solving
the complexities of consent in genomic research. Eur
J Hum Genet, 29(4):687–698.
Hewitt, R. and Watson, P. (2013). Defining biobank. Biop-
reservation and biobanking, 11(5):309–315.
Holm, S. and Ploug, T. (2017). Big data and health re-
search—the governance challenges in a mixed data
economy. Journal of bioethical inquiry, 14:515–525.
Kaye, J., Whitley, E. A., Lund, D., Morrison, M., Teare, H.,
and Melham, K. (2015). Dynamic consent: a patient
interface for twenty-first-century research networks.
Eur J Hum Genet, 23(2):141–146.
Lee, H. and Lee, U. (2022). Toward dynamic consent
for privacy-aware pervasive health and well-being: A
scoping review and research directions. IEEE Perva-
sive Computing.
Mamo, N., Martin, G. M., Desira, M., Ellul, B., and Ebe-
jer, J. P. (2020). Dwarna: a blockchain solution for
dynamic consent in biobanking. Eur J Hum Genet,
28(5):609–626.
Mascalzoni, D., Melotti, R., Pattaro, C., Pramstaller, P. P.,
G
¨
ogele, M., De Grandi, A., and Biasiotto, R. (2022).
Ten years of dynamic consent in the chris study: in-
formed consent as a dynamic process. Eur J Hum
Genet, 30(12):1391–1397.
Mirza, A. B., Khoja, A. K., Ali, F., El-Sheikh, M., Bibi-
Shahid, A., Trindade, J., Rocos, B., Grahovac, G.,
Bull, J., and Montgomery, A. (2023). The use of e-
consent in surgery and application to neurosurgery:
a systematic review and meta-analysis. Acta Neu-
rochirurgica, pages 1–32.
Ploug, T. and Holm, S. (2015). Meta consent: a flexible
and autonomous way of obtaining informed consent
for secondary research. BMJ, 350:h2146.
Skelton, E., Drey, N., Rutherford, M., Ayers, S., and Mala-
mateniou, C. (2020). Electronic consenting for con-
ducting research remotely: A review of current prac-
tice and key recommendations for using econsent-
e-Consent in Biomedical Research Registries: A GDPR-Compliant Approach Explored in the Context of the Australasian Diabetes Data
Network
53