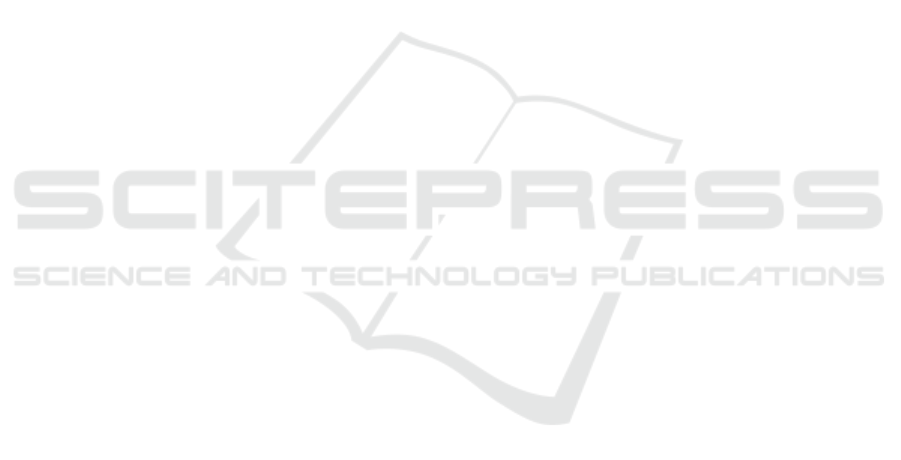
cancer types in size, shape, and location, which is
also very complex due to tumor diversity and inter-
individual variability. Due to these simulation com-
plexities, clinical trials with a sufficient and diverse
population are mandatory to validate wearable de-
vices for breast cancer detection.
Ethical and privacy considerations are of
paramount importance when developing and using
breast wearable devices for breast cancer detection.
These devices collect sensitive health data, and
their usage must adhere to strict ethical and privacy
standards. Obtaining informed consent from users is
crucial, users should fully understand the purpose of
the wearable device, how their data will be collected
and used, and any potential risks or benefits. Breast
wearable devices should employ robust encryption
and data protection measures to safeguard user
information from unauthorized access or breaches.
Ensuring data security is particularly important in the
healthcare context, where data can be sensitive and
personally identifiable.
Apart from diagnostic accuracy, clinical valida-
tion should assess the device’s usability in real-world
clinical settings. Factors such as ease of use, inte-
gration into existing healthcare workflows, and user
acceptance are important considerations to take in fu-
ture works.
6 CONCLUSION
In conclusion, the field of smart wearable devices
equipped with thermal sensors represents a promis-
ing frontier in breast cancer detection. These inno-
vative technologies offer a multitude of advantages,
from non-invasiveness and early detection to acces-
sibility and cost-effectiveness. However, as with any
new technology, there are many challenges to over-
come. Clinical validation, population diversity in tri-
als, ethical considerations, and privacy safeguards are
among the critical issues that demand careful atten-
tion.
Through this review, we can say that smart wear-
able devices with thermal sensors for breast cancer
detection projects are not mature enough to be clini-
cally and widely used, but addressing the challenges
can make these devices more effective, accessible,
and user-friendly. These devices hold the promise
of detecting breast cancer at earlier stages, reducing
healthcare disparities, and transforming breast health
awareness. With continued research, validation, and
collaboration between the medical community and
technology developers, they may well become an ac-
curate and validated breast detection method.
REFERENCES
Al Masry, Z., Zerhouni, N., Gay, C., Meraghni, S., Lodi,
and Devalland, C. (2021). D
´
etection du cancer du sein
`
a l’aide de soutiens-gorge connect
´
es en 2021 : analy-
ses et perspectives. 49(12):907–912.
Antony, L., Arathy, K., Sudarsan, N., Muralidharan, M. N.,
and Ansari, S. (2020). Breast tumor parameter estima-
tion and interactive 3d thermal tomography using dis-
crete thermal sensor data. Biomedical Physics &Engi-
neering Express, 7(1):015013.
Arathy, K., Ansari, S., and Malini, K. A. High reliability
thermistor probes for early detection of breast cancer
using skin contact thermometry with thermal imaging.
10(5):620–628.
Arnold, M., Morgan, E., Rumgay, H., Mafra, A., Singh, D.,
Laversanne, M., Vignat, J., Gralow, J. R., Cardoso, F.,
Siesling, S., and Soerjomataram, I. (2022). Current
and future burden of breast cancer: Global statistics
for 2020 and 2040. The Breast, 66:15–23.
Ashreetha, B., V, D. G., Anandaram, H., A, N. B., Gupta,
N., and Verma, B. K. (2023). Iot wearable breast tem-
perature assessment system.
Elouerghi, A., Bellarbi, L., Khomsi, Z., Jbari, A., Errachid,
A., and Yaakoubi, N. (2022). A flexible wearable ther-
mography system based on bioheat microsensors net-
work for early breast cancer detection: IoT technol-
ogy. Journal of Electrical and Computer Engineering,
2022:1–13.
Halim, A. A. A., Andrew, A. M., Yasin, M. N. M., Rahman,
M. A. A., Jusoh, M., Veeraperumal, V., Rahim, H. A.,
Illahi, U., Karim, M. K. A., and Scavino, E. (2021).
Existing and emerging breast cancer detection tech-
nologies and its challenges: A review. Applied Sci-
ences, 11(22):10753.
Hylton, N. (2005). Magnetic resonance imaging of the
breast: Opportunities to improve breast cancer man-
agement. Journal of Clinical Oncology, 23(8):1678–
1684.
Laila Fadhillah, U. D., Nur Afikah, Z. A., Safiee, N. E. N.,
Asnida, A. W., and Rafiq, M. (2018). Development
of a low-cost wearable breast cancer detection device.
pages 41–46.
Lee, A. H. (2005). Why is carcinoma of the breast more
frequent in the upper outer quadrant? a case series
based on needle core biopsy diagnoses. The Breast,
14(2):151–152.
Meijer, G. C., Wang, G., and Heidary, A. (2018). Smart
temperature sensors and temperature sensor systems.
In Smart Sensors and MEMs, pages 57–85. Elsevier.
Michaels, E., Worthington, R. O., and Rusiecki, J. (2023).
Breast cancer. Medical Clinics of North America,
107(2):271–284.
Ng, E., Acharya, U. R., Keith, L. G., and Lockwood, S.
(2007). Detection and differentiation of breast can-
cer using neural classifiers with first warning thermal
sensors. Information Sciences, 177(20):4526–4538.
Ng, E. Y. K. (2001). Statistical analysis of healthy and ma-
lignant breast thermography. Journal of Medical En-
gineering & Technology, 25(6):253–263.
BIODEVICES 2024 - 17th International Conference on Biomedical Electronics and Devices
32