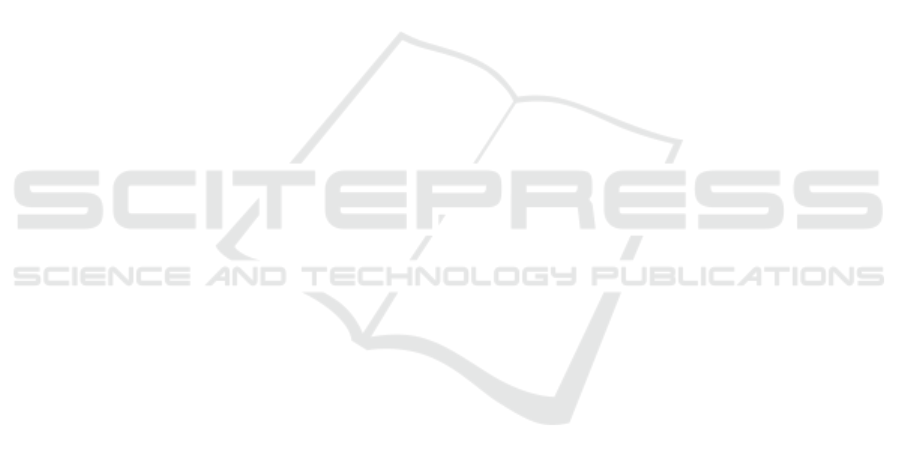
use of other explainable models (e.g. interpretable
ANNs) or representation learning. To increase the
amount of input image, augmentation will be taken
into consideration.
REFERENCES
Bajcsi, A. and Chira, C. (2023). Textural and shape features
for lesion classification in mammogram analysis. In
Hybrid Artificial Intelligent Systems, pages 755–767.
Springer Nature Switzerland.
Chhikara, B. S. and Parang, K. (2022). Global cancer statis-
tics 2022: the trends projection analysis. Chemical
Biology Letters, 10(1):451.
Falcon
´
ı, L. G., P
´
erez, M. H., Aguila, W. G., and Conci,
A. (2020). Transfer learning and fine tuning in breast
mammogram abnormalities classification on CBIS-
DDSM database. Advances in Science, Technology
and Engineering Systems Journal, 5(2):154–165.
George Melekoodappattu, J., Sahaya Dhas, A., Kumar K.,
B., and Adarsh, K. S. (2022). Malignancy detection on
mammograms by integrating modified convolutional
neural network classifier and texture features. Inter-
national Journal of Imaging Systems and Technology,
32(2):564–574.
Gurudas, V. R., Shaila, S. G., and Vadivel, A. (2022). Breast
cancer detection and classification from mammogram
images using multi-model shape features. SN Com-
puter Science, 3(5):404.
Heath, M., Bowyer, K., Kopans, D., Kegelmeyer, P., Moore,
R., Chang, K., and Munishkumaran, S. (1998). Cur-
rent Status of the Digital Database for Screening
Mammography, pages 457–460. Springer Nether-
lands, Dordrecht.
Heath, M., Bowyer, K., Kopans, D., Moore, R., and
Kegelmeyer, P. (2001). The digital database for
screening mammography. In Yaffe, M., editor, Pro-
ceedings of the Fifth International Workshop on Dig-
ital Mammography, pages 212–218. Medical Physics
Publishing.
Kumari, D., Yannam, P. K. R., Gohel, I. N., Naidu, M. V.
S. S., Arora, Y., Rajita, B., Panda, S., and Christo-
pher, J. (2023). Computational model for breast can-
cer diagnosis using hfse framework. Biomedical Sig-
nal Processing and Control, 86:105121.
Lee, R. S., Gimenez, F., Hoogi, A., Miyake, K. K., Gorovoy,
M., and Rubin, D. L. (2017). A curated mammogra-
phy data set for use in computer-aided detection and
diagnosis research. Sci Data, 4:170–177.
Li, H., Meng, X., Wang, T., Tang, Y., and Yin, Y. (2017).
Breast masses in mammography classification with lo-
cal contour features. BioMedical Engineering OnLine,
16(1):44.
Paramkusham, S., Thotempuddi, J., and Rayudu, M. S.
(2021). Breast masses classification using contour
shape descriptors based on beam angle statistics. In
2021 Third International Conference on Inventive Re-
search in Computing Applications (ICIRCA), pages
1340–1345.
Rani, J., Singh, J., and Virmani, J. (2023). Mammographic
mass classification using dl based roi segmentation
and ml based classification. In 2023 International
Conference on Device Intelligence, Computing and
Communication Technologies, (DICCT), pages 302–
306.
Sajid, U., Khan, R. A., Shah, S. M., and Arif, S. (2023).
Breast cancer classification using deep learned fea-
tures boosted with handcrafted features. Biomedical
Signal Processing and Control, 86:105353.
Salama, W. M. and Aly, M. H. (2021). Deep learning
in mammography images segmentation and classifi-
cation: Automated cnn approach. Alexandria Engi-
neering Journal, 60(5):4701–4709.
Shanmugam, S., Shanmugam, A. K., Mayilswamy, B., and
Muthusamy, E. (2020). Analyses of statistical feature
fusion techniques in breast cancer detection. Inter-
national Journal of Applied Science and Engineering,
17:311–317.
Singh, V. K., Rashwan, H. A., Romani, S., Akram, F.,
Pandey, N., Sarker, M. M. K., Saleh, A., Arenas, M.,
Arquez, M., Puig, D., and Torrents-Barrena, J. (2020).
Breast tumor segmentation and shape classification in
mammograms using generative adversarial and con-
volutional neural network. Expert Systems with Ap-
plications, 139:112855.
ICAART 2024 - 16th International Conference on Agents and Artificial Intelligence
124