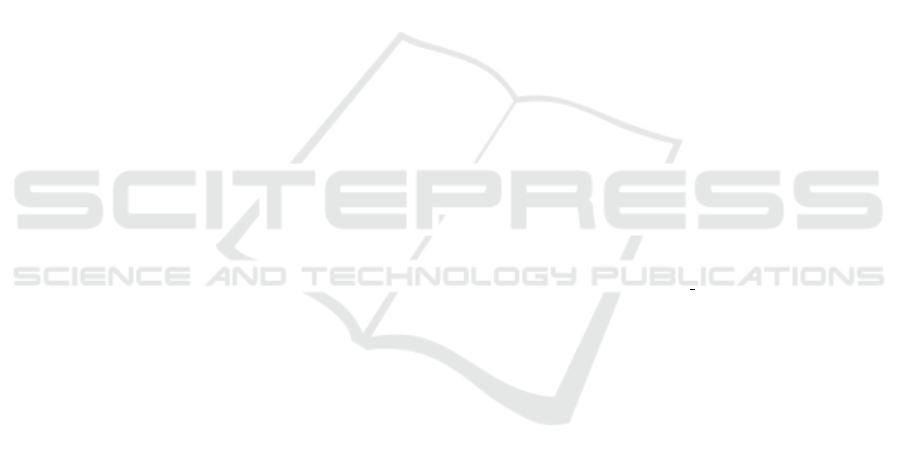
Gray, C. M., Santos, C., and Bielova, N. (2023). Towards a
preliminary ontology of dark patterns knowledge. In
Proc. of CHI EA, pages 1–9.
Gunawan, J., Santos, C., and Kamara, I. (2022). Redress for
dark patterns privacy harms? A case study on consent
interactions. In Proc. of CSLAW, pages 181–194.
Halvorsrud, R., Boletsis, C., and Garcia-Ceja, E. (2021).
Designing a modeling language for customer jour-
neys: Lessons learned from user involvement. In Proc.
of ACM/IEEE MODELS, pages 239–249.
Halvorsrud, R., Haugstveit, I. M., and Pultier, A. (2016).
Evaluation of a modelling language for customer jour-
neys. In Proc. of IEEE VL/HCC, pages 40–48.
Halvorsrud, R., Sanchez, O. R., Boletsis, C., and Skjuve,
M. (2023). Involving users in the development of
a modeling language for customer journeys. Softw.
Syst. Model., Online first. DOI: 10.1007/s10270-023-
01081-w.
Hedrick, E., Harper, M., Oliver, E., and Hatch, D. (2022).
Teaching & learning in virtual reality: Metaverse
classroom exploration. In Proc. of IETC, pages 1–5.
Heurix, J., Zimmermann, P., Neubauer, T., and Fenz, S.
(2015). A taxonomy for privacy enhancing technolo-
gies. Computers & Security, 53:1–17.
Holtz, L.-E., Nocun, K., and Hansen, M. (2011). Towards
displaying privacy information with icons. IFIPAICT,
352:338–348.
Hwang, G.-J., Tu, Y.-F., and Chu, H.-C. (2023). Concep-
tions of the metaverse in higher education: A draw-
a-picture analysis and surveys to investigate the per-
ceptions of students with different motivation levels.
Comput. Educ., 203:104868.
IFI Claims Patent Services (2022). Ifi insights: Inventing
the metaverse. https://www.ificlaims.com/news/view/
briefing-metaverse.htm. Accessed: 2023-09-07.
Jones, T. (2010). Electronic Frontier Foundation: Face-
book’s ”evil interfaces”. https://www.eff.org/deep
links/2010/04/facebooks-evil-interfaces. Accessed:
2023-09-07.
Lee, J., Lee, T. S., Lee, S., Jang, J., Yoo, S., Choi, Y., Park,
Y. R., et al. (2022). Development and application of
a metaverse-based social skills training program for
children with autism spectrum disorder to improve so-
cial interaction: Protocol for a randomized controlled
trial. JMIR Res. Protoc., 11(6):e35960.
Massironi, M. et al. (2001). The psychology of graphic im-
ages: Seeing, drawing, communicating. Psychology
Press.
Murphy, H. (2022). Financial Times: Facebook patents re-
veal how it intends to cash in on metaverse. https:
//www.ft.com/content/76d40aac-034e-4e0b-95eb-c
5d34146f647. Accessed: 2023-09-07.
Ning, H., Wang, H., Lin, Y., Wang, W., Dhelim, S., Farha,
F., Ding, J., and Daneshmand, M. (2023). A survey
on the metaverse: The state-of-the-art, technologies,
applications, and challenges. IEEE Internet Things J.
Roesner, F., Kohno, T., and Molnar, D. (2014). Security
and privacy for augmented reality systems. Commun.
ACM, 57(4):88–96.
Sanchez, J. (2007). Second life: An interactive qualitative
analysis. In Proc. of SITE, pages 1240–1243.
Skorupska, K., Grzeszczuk, M., Jaskulska, A., Kornacka,
M., Pochwatko, G., and Kope
´
c, W. (2022). A case
for vr briefings: Comparing communication in daily
audio and vr mission control in a simulated lunar mis-
sion. In Proc. of MIDI, pages 287–297.
Stahl, J. D., Sead, N., Murrell, T., Germe, G. D. L.,
and Kish, S. (2020). Suggestion of content
within augmented-reality environments. US Patent
10,719,989.
ˇ
Starcho
ˇ
n, P. and Pikul
´
ık, T. (2019). GDPR principles in data
protection encourage pseudonymization through most
popular and full-personalized devices-mobile phones.
Procedia Comput. Sci., 151:303–312.
Teller, E. (2023). Measurement method and system. US
Patent 11,579,442.
Voigt, P. and Von dem Bussche, A. (2017). The EU Gen-
eral Data Protection Regulation (GDPR): A Practical
Guide, 1st Ed. Springer.
Waldman, A. E. (2020). Cognitive biases, dark patterns, and
the ‘privacy paradox’. Curr. Opin. Psychol., 31:105–
109.
Wang, Y., Su, Z., Zhang, N., Xing, R., Liu, D., Luan, T. H.,
and Shen, X. (2022). A survey on metaverse: Funda-
mentals, security, and privacy. IEEE Commun. Surv.
Tutor.
Warin, C. and Reinhardt, D. (2022). Vision: Usable pri-
vacy for xr in the era of the metaverse. In Proc. of
EuroUSEC, pages 111–116.
Weingarden, G. and Artzt, M. (2022). International associ-
ation of privacy professionals: Metaverse and privacy.
https://edps.europa.eu/press-publications/publicatio
ns/techsonar/metaverse\ en. Accessed: 2023-09-07.
World Economic Forum (2023). Metaverse privacy and
safety. https://www.weforum.org/reports/privacy-and
-safety-in-the-metaverse/. Accessed: 2023-09-07.
XR Safety Initiative (2020). The XRSI Privacy Framework.
https://xrsi.org/publication/the-xrsi-privacy-framewo
rk. Accessed: 2023-09-07.
Xu, M., Guo, Y., Hu, Q., Xiong, Z., Yu, D., and Cheng, X.
(2023). A trustless architecture of blockchain-enabled
metaverse. High-Confid., 3(1):100088.
Zolezzi, D., Vercelli, G. V., et al. (2023). Teaching in the
Metaverse: Recreating an Italian Level A1 Course in
Meta Horizon Woorkrooms. In Proc. of The Future of
Education, pages 1–5.
Zuboff, S. (2019). The age of surveillance capitalism: The
fight for a human future at the new frontier of power.
PublicAffairs.
Ethical Design for Data Privacy and User Privacy Awareness in the Metaverse
341