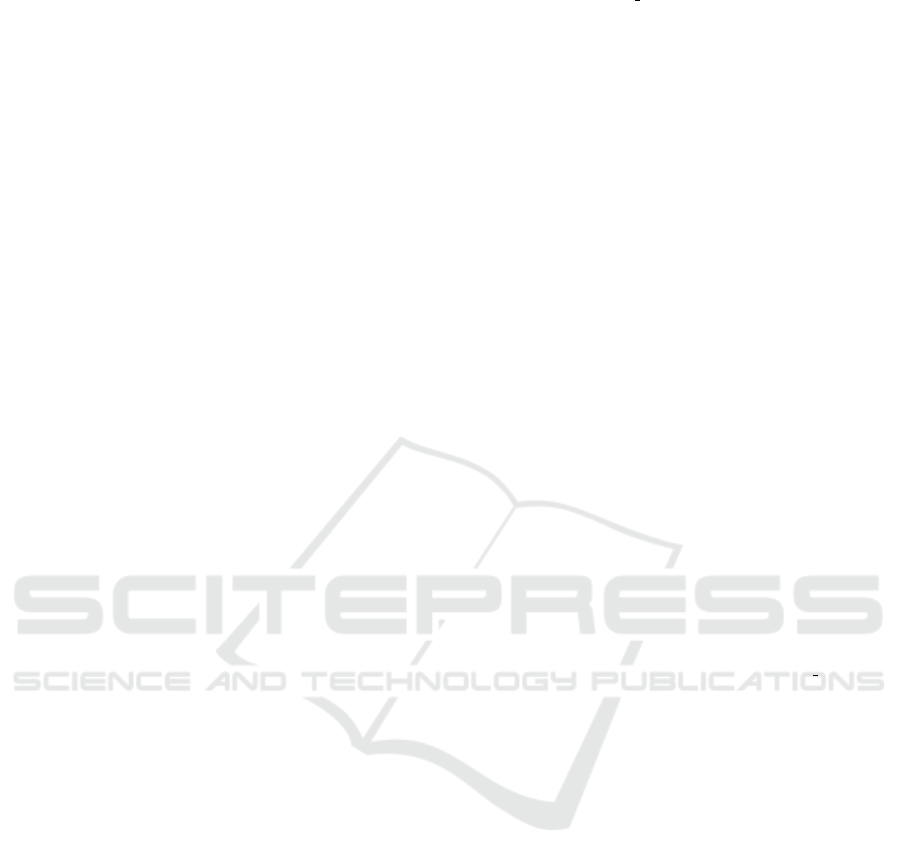
T. T. (2020). Species-level image classification with
convolutional neural network enables insect identifi-
cation from habitus images. Ecology and Evolution,
10(2):737–747.
He, K., Gkioxari, G., Doll
´
ar, P., and Girshick, R. (2017).
Mask r-cnn. In Proceedings of the IEEE international
conference on computer vision, pages 2961–2969.
Hebert, L., Ahamed, T., Costa, A. C., O’Shaughnessy,
L., and Stephens, G. J. (2021). Wormpose: Image
synthesis and convolutional networks for pose esti-
mation in c. elegans. PLoS computational biology,
17(4):e1008914.
Husson, S. J., Costa, W. S., Schmitt, C., and Gottschalk, A.
(2018). Keeping track of worm trackers. WormBook:
The Online Review of C. elegans Biology [Internet].
Jocher, G. (2020). YOLOv5 by Ultralytics.
Jocher, G., Chaurasia, A., and Qiu, J. (2023., {https://
github.com/ultralytics/ultralytics}). Yolo by ultralyt-
ics.
Kr
¨
oncke, N. and Benning, R. (2022). Self-selection of feed-
ing substrates by tenebrio molitor larvae of different
ages to determine optimal macronutrient intake and
the influence on larval growth and protein content. In-
sects, 13(7):657.
Labuguen, R., Matsumoto, J., Negrete, S. B., Nishimaru,
H., Nishijo, H., Takada, M., Go, Y., Inoue, K.-i., and
Shibata, T. (2021). Macaquepose: a novel “in the
wild” macaque monkey pose dataset for markerless
motion capture. Frontiers in behavioral neuroscience,
14:581154.
Lin, T.-Y., Maire, M., Belongie, S., Hays, J., Perona, P.,
Ramanan, D., Doll
´
ar, P., and Zitnick, C. L. (2014).
Microsoft coco: Common objects in context. In Com-
puter Vision–ECCV 2014: 13th European Confer-
ence, Zurich, Switzerland, September 6-12, 2014, Pro-
ceedings, Part V 13, pages 740–755. Springer.
Liu, W., Anguelov, D., Erhan, D., Szegedy, C., Reed, S.,
Fu, C.-Y., and Berg, A. C. (2016). Ssd: Single shot
multibox detector. In Computer Vision–ECCV 2016:
14th European Conference, Amsterdam, The Nether-
lands, October 11–14, 2016, Proceedings, Part I 14,
pages 21–37. Springer.
Majewski, P., Zapotoczny, P., Lampa, P., Burduk, R., and
Reiner, J. (2022). Multipurpose monitoring system
for edible insect breeding based on machine learning.
Scientific Reports, 12(1):1–15.
Ng, X. L., Ong, K. E., Zheng, Q., Ni, Y., Yeo, S. Y., and
Liu, J. (2022). Animal kingdom: A large and di-
verse dataset for animal behavior understanding. In
Proceedings of the IEEE/CVF Conference on Com-
puter Vision and Pattern Recognition (CVPR), pages
19023–19034.
Nguyen, M. (2020). Datatorch annotation tool. https:
//datatorch.io/.
Nuthalapati, S. V. and Tunga, A. (2021). Multi-domain few-
shot learning and dataset for agricultural applications.
In Proceedings of the IEEE/CVF International Con-
ference on Computer Vision, pages 1399–1408.
of the European Union, O. J. (2023). Commission im-
plementing regulation (eu) 2023/58. https://eur-lex.
europa.eu/eli/reg impl/2023/58/oj. Official Journal of
the European Union, 66.
Oonincx, D. G. and De Boer, I. J. (2012). Environmental
impact of the production of mealworms as a protein
source for humans–a life cycle assessment. PloS one,
7(12):e51145.
Pereira, T. D., Aldarondo, D. E., Willmore, L., Kislin,
M., Wang, S. S.-H., Murthy, M., and Shaevitz, J. W.
(2019). Fast animal pose estimation using deep neural
networks. Nature methods, 16(1):117–125.
Probst, A. (2023). larvae dataset. https://universe.
roboflow.com/andre-probst-egt9g/larvae-bbsvy. vis-
ited on 2023-02-23.
Redmon, J., Divvala, S., Girshick, R., and Farhadi, A.
(2016). You only look once: Unified, real-time object
detection. In Proceedings of the IEEE conference on
computer vision and pattern recognition, pages 779–
788.
Siemianowska, E., Kosewska, A., Aljewicz, M., Skib-
niewska, K. A., Polak-Juszczak, L., Jarocki, A., and
Jedras, M. (2013). Larvae of mealworm tenebrio moli-
tor l. as european novel food.
Stoops, J., Crauwels, S., Waud, M., Claes, J., Lievens, B.,
and Van Campenhout, L. (2016). Microbial commu-
nity assessment of mealworm larvae (tenebrio moli-
tor) and grasshoppers (locusta migratoria migratori-
oides) sold for human consumption. Food Microbiol-
ogy, 53:122–127.
Tan, M., Pang, R., and Le, Q. V. (2020). Efficientdet: Scal-
able and efficient object detection. In Proceedings
of the IEEE/CVF conference on computer vision and
pattern recognition, pages 10781–10790.
UI-3884LE (2021). Ids. ui-3884le-c-hq camera (ab00979).
https://en.ids-imaging.com/IDS/datasheet pdf.php?
sku=AB00979.
Van Horn, G., Mac Aodha, O., Song, Y., Cui, Y., Sun,
C., Shepard, A., Adam, H., Perona, P., and Belongie,
S. (2018). The inaturalist species classification and
detection dataset. In Proceedings of the IEEE con-
ference on computer vision and pattern recognition,
pages 8769–8778.
Van Huis, A., Van Itterbeeck, J., Klunder, H., Mertens, E.,
Halloran, A., Muir, G., and Vantomme, P. (2013). Ed-
ible insects: future prospects for food and feed secu-
rity. Number 171. Food and agriculture organization
of the United Nations.
Wah, C., Branson, S., Welinder, P., Perona, P., and Be-
longie, S. (2011). The caltech-ucsd birds-200-2011
dataset.
Wang, C.-Y., Bochkovskiy, A., and Liao, H.-Y. M. (2022).
Yolov7: Trainable bag-of-freebies sets new state-of-
the-art for real-time object detectors. arXiv preprint
arXiv:2207.02696.
Wu, X., Zhan, C., Lai, Y.-K., Cheng, M.-M., and Yang,
J. (2020). Ip102: A large-scale benchmark dataset
for insect pest recognition. In Proceedings of the
IEEE/CVF conference on computer vision and pattern
recognition, pages 8787–8796.
Wu, Y., Kirillov, A., Massa, F., Lo, W.-Y., and Gir-
shick, R. (2019). Detectron2. https://github.com/
facebookresearch/detectron2.
ICPRAM 2024 - 13th International Conference on Pattern Recognition Applications and Methods
196