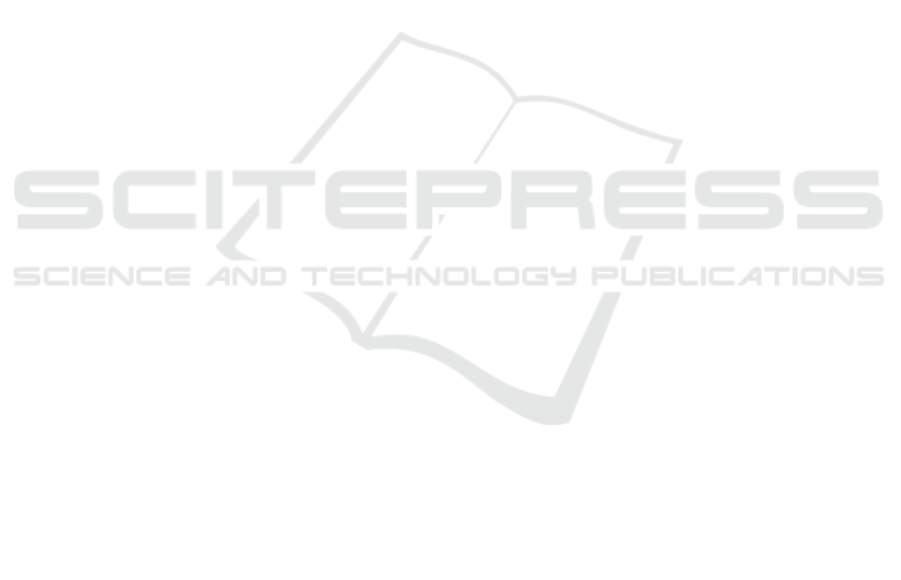
figure 7 if the change is from Barren to Vegetation,
values will only occur in a pixel before Barren, then
Transition potential maps are generated.
In 1991, Barren Land accounted for around 75 %
of the overall Bengaluru District Boundary, while
Vegetation accounted for 9.2 %, and built-up area
accounted for 15 % of the total Bengaluru District
Boundary. Then, in 2001, we can see that bare land
decreased to 30.7 %, while built-up has expanded 9.2
% since 1991 and Vegetation rose exponentially to
29.2 %. Between 2001 and 2011, barren land was
reduced by 8%, and Vegetation was decreased by 4%,
resulting in an 8.2% increase in an urban area in 2011
as barren plot was transformed into the urbanized
area. From 2011 to 2021, barren land was reduced by
14.81%, and Vegetation has been increased by 2%,
resulting in a 13.9 % increase in an urban area in 2021
(figure 5-7).
Because the Accuracy of the Land Change
Modeler is 79.02 percent, we can claim it will predict
about 80 percent of the time. As shown, Bengaluru’s
future urban growth and the direction in which the
city is expanding are visible.
Between 2021 and 2031, bare land will be reduced
by 5.78%, and Vegetation will be reduced by 4%,
resulting in a 7.88% increase in urban areas in 2031.
The total built-up area will increase by 54.6 %.
Between 2031 and 2041, bare land will be reduced by
4 %, and Vegetation will be reduced by 2%, resulting
in a 6.4 % increase in urban areas in 2041. The total
built-up area will increase by 61 %.
6 CONCLUSIONS
Using Shannon Entropy, we can see that the most
substantial change from 1991 to 2021 happened in the
Southeast direction, where the Built-up region has
increased. This study concludes the challenges and
issues of urbanization in Bengaluru (Gupta J, 2022).
The solutions to these concerns are GIS data and
raster data are employed. Raster data are collected
from the google earth engine & GIS Data are gathered
from different web portals & studied various research
literature in the journal about the problem. To bring
this study to a close, qualitative, and quantitative tools
were examined. This paper explains the logical
method, which must be associated with the CA
Markov Model and the Shannon Entropy Study.
This report requires Future research of
Bengaluru’s changing spatial patterns of urban
growth. It is challenging to identify significant
differences between agriculture and parks because of
the low spatial resolution of Landsat 5 & 7 (Gupta et
al, 2015). The CA Markov model has a drawback in
that it cannot be employed for short time intervals.
While calibration is the most crucial procedure for
determining which parameters are appropriate for the
model, this model has been run more than 15 times.
Each time the parameters change, the results vary.
REFERENCES
Bhat, V., Aithal, B. H., & Ramachandra, T. V. (2015).
Spatial patterns of urban growth with globalisation in
India’s Silicon Valley. Organized By Department of
Civil Engineering, Indian Institute of Technology
(Banaras Hindu University), Varanasi-221005 Uttar
Pradesh, India, 98.
Baqa, M. F., Chen, F., Lu, L., Qureshi, S., Tariq, A., Wang,
S., ... & Li, Q. (2021). Monitoring and modeling the
patterns and trends of urban growth using urban sprawl
matrix and CA-Markov model: A case study of
Karachi, Pakistan. Land, 10(7), 700.
Mishra, V. N., Rai, P. K., & Mohan, K. (2014). Prediction
of land use changes based on land change modeler
(LCM) using remote sensing: A case study of
Muzaffarpur (Bihar), India. Journal of the
Geographical Institute" Jovan Cvijic", SASA, 64(1),
111-127.
Jain, S., Siddiqui, A., Tiwari, P. S., & Shashi, M. (2016).
Urban growth assessment using CA Markov model: A
case study of Dehradun City. 9th International
Geographic Union.
Jadawala, S., Shukla, S. H., & Tiwari, P. S. (2021). Cellular
automata and markov chain based urban growth
prediction. International Journal of Environment and
Geoinformatics, 8(3), 337-343.
Yadav, V., & Ghosh, S. K. (2021). Assessment and
prediction of urban growth for a mega-city using CA-
Markov model. Geocarto International, 36(17), 1960-
1992.
Gupta, J. (2022, November). Statistical Assessment of
Spatial Autocorrelation on Air Quality in Bengaluru,
India. In International Conference on Intelligent Vision
and Computing (pp. 254-265). Cham: Springer Nature
Switzerland.
Gupta, A. J., & Subrahmanian, R. R. Design Challenges of
Project Deliverables in Construction Industry in
Bangalore.
Urban Growth Modelling Based on CA-Markov Approach on Bengaluru India
391