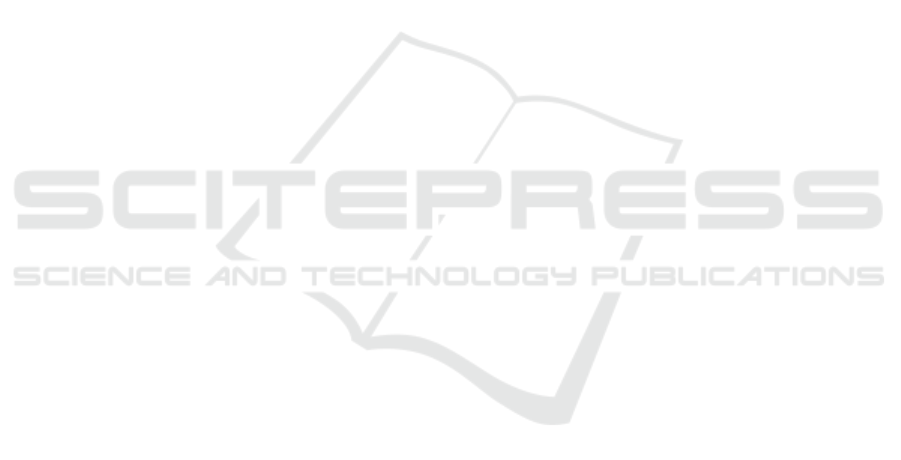
the temperature data to achieve more accurate
predictions.
However, some limitations remain. First of all, the
forecast in this paper is based on historical data, and
possible unexpected events or contingencies in the
future cannot be fully taken into account. In addition,
the paper solely relies on temperature data as a
forecasting variable, while other important factors like
precipitation and wind speed may be necessary to
consider in real-world scenarios.
Although this study has certain limitations, it offers
valuable insights into the field of meteorology and
climate research in Stockholm. Moreover, its findings
hold practical application potential in various contexts.
Future studies can further improve the model and
introduce more external variables to improve the
accuracy and reliability of temperature predictions.
In further research, other time series models or
hybrid models, such as VARIMA, can be considered
to seek more accurate temperature prediction methods.
These models are able to combine seasonal, trend and
cyclical factors to further improve the accuracy of
temperature forecasts.
In addition, the predictive performance of different
models can be compared to assess their strengths and
weaknesses in the Stockholm temperature prediction.
By comparing it with other prediction models, the
model that is most suitable for the region can be
selected and more reliable predictions can be made for
relevant decisions.
In summary, the ARIMA model is used to predict
the temperature in Stockholm, and the results are more
accurate and robust. However, further improvement
and exploration are still needed to obtain more accurate
and reliable temperature prediction results and apply
them to practical meteorological and climate studies.
4 CONCLUSION
In conclusion, the developed seasonal ARIMA model,
utilizing the meteorological data from Stockholm
City's monthly average temperature spanning from
1980 to 2020, has demonstrated high forecasting
accuracy. The improved predictive accuracy of this
model will assist relevant departments in formulating
effective strategies and measures to address the
consequences of fluctuating temperature changes. By
taking proactive measures beforehand, potential
impacts can be mitigated.
However, there is scope for further improvement in
order to enhance the accuracy and stability of the
model's predictions. Different techniques or
approaches can be explored to address these areas and
achieve better results. Future research should explore
additional influencing factors when predicting time
series, which would offer new insights into time series
forecasting. Incorporating these factors into the model
will likely enhance its overall performance and provide
a more robust understanding of temperature
fluctuations.
In summary, while the present study successfully
developed a seasonal ARIMA model with impressive
forecasting accuracy for monthly average temperatures
in Stockholm City, further enhancements are
necessary. Integrating additional influencing factors
and expanding the dataset will enable more reliable
predictions and support informed decision-making in
response to temperature changes.
REFERENCES
S. Qasmi and A. Ribes, “Reducing uncertainty in local
temperature projections,” Science Advances, Oct. 2022,
vol. 8, no. 41.
T. Dimri, S. Ahmad, and M. Sharif, “Time series analysis of
climate variables using seasonal ARIMA approach,”
Journal of Earth System Science, Jun. 2020, vol. 129, no.
1.
H. Wu, J. Wang, W. Wu, H. Zhao, and P. Zhang, “Prediction
and Analysis of Global Gas Gauge Temperature Based
on ARIMA Model,” Modern Information Technology,
Aug. 2023, vol. 16, no. 7, pp. 145–150.
S. Peng, X. Zhang, M. Li, and Y. Xiang, “Drought
Prediction Based on ARIMA Model and VSWI index: a
Case Study of Northwest Guangxi,” Geomatics &
Spatial Information Technology, Dec. 2022, vol. 12, no.
45, pp. 38–41, 44.
M. Amjad, A. Khan, K. Fatima et al, “Analysis of
Temperature Variability, Trends and Prediction in the
Karachi Region of Pakistan Using ARIMA Models,”
Atmospher, Dec. 2022e, vol. 14, no. 1, p. 88.
F. Liu and R. Ge, “Prediction and Analysis of Winter Daily
Minimum Temperature Based on ARIMA,” Electronic
Technology & Software Engineering, Jun. 2022, vol. 12,
pp. 184–188.
Z. Wu, “Construction and Analysis of Temperature Change
Prediction Model in Z.Z City,” Science & Technology
Ecnony Market, Apr. 2018, vol. 3, pp. 33–34, 146.
R. Kesavan, M. Muthian, K. Sudalaimuthu, S. Sundarsingh,
and S. Krishnan, “ARIMA modeling for forecasting
land surface temperature and determination of urban
heat island using remote sensing techniques for Chennai
city, India,” Arabian Journal of Geosciences, May 2021,
vol. 14, no. 11.
H. S. Hippert, C. E. Pedreira, and R. C. Souza, “Combining
neural networks and ARIMA models for hourly
temperature forecast,” Proceedings of the IEEE-INNS-
ENNS International Joint Conference on Neural
Networks. IJCNN 2000. Neural Computing: New
Challenges and Perspectives for the New Millennium,
2000, vol. 4.
Weather Forecast Analysis Based on ARIMA Model: A Case Study of Stockholm
169