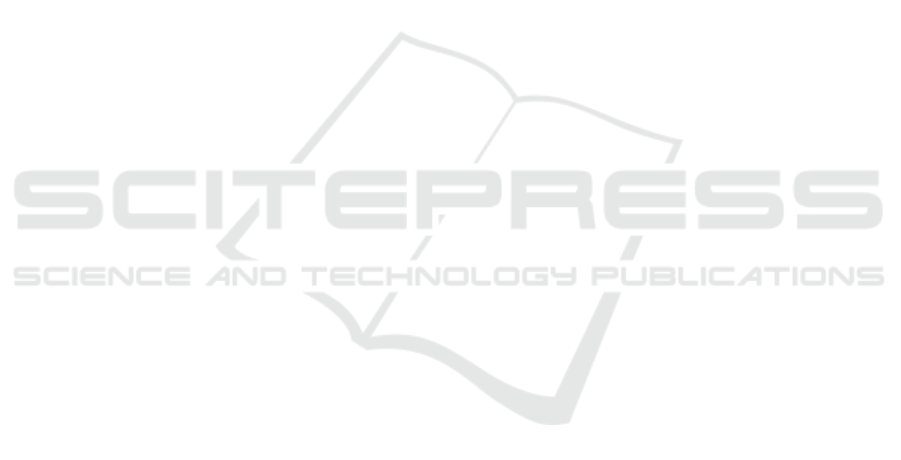
AI-based detection systems can defend against
cyber threats by analyzing network traffic, identifying
anomalies, detecting potential intrusions, and
predicting attack patterns through deep learning. This
proactive and adaptive mechanism empowers
governments to protect sensitive information with
enhanced efficiency. Still, challenges must be
addressed. The rapidly changing cyber threat
environment requires AI models to be continuously
updated and improved. It is also essential to safeguard
citizens' data privacy and security when using AI-
based detection. Achieving the correct balance
between security and privacy remains an ongoing
issue.
AI-based detection holds great promise for the
future. As AI systems continue to advance and gain
the capacity to learn from vast datasets, they will
acquire the capability to detect cyber threats that are
growing in complexity. Collaborative work between
governments, research institutions and the private
sector will be crucial to effectively utilise AI's full
potential in securing vital networks and infrastructure.
2.4 Company
In the business world, using AI to detect harmful
network activity is a significant development that
helps to maintain the smooth running of operations,
safeguard important data, and keep customers
confident in the company. All kinds of organisations,
from those in finance to healthcare, handle huge
quantities of valuable information, making them
potential targets for cyber criminals.
AI-driven detection programmes give businesses
the means to tighten their defences against these
threats and improve their overall cybersecurity. These
systems can quickly spot and address dangers,
reducing the risk of harm. Furthermore, AI can help to
evaluate and anticipate risks, allowing people to take
anticipatory measures to manage risks proficiently.
Nevertheless, establishments are grappling with
difficulties implementing AI-based answers, primarily
investing in AI tech, assimilating the solutions with
current cyber protection installations, and addressing
the lack of adept AI professionals. Overcoming these
challenges is crucial to reap the rewards of AI in
boosting network security.
In the future, AI will advance and provide even
more advanced detection techniques. This will enable
firms to outsmart cyber attackers. Collaborations
between companies and cybersecurity firms will also
fuel innovation and hasten the growth of AI-powered
solutions for network security.
2.5 Challenges and Future Prospects
Despite progress with AI-driven identification of
harmful network traffic, there are still challenges to be
tackled. Attackers are always altering their strategies,
creating difficulties in devising AI systems that can
precisely detect innovative and unknown attack
approaches. Moreover, an unequal distribution of
datasets (with few harmful occurrences compared to
harmless ones) presents a challenge in training AI
models effectively. Therefore, more advanced AI
models that have been widely applied in other
domains can be also considered in this field for further
improving the performance (Yu et al 2020 & Malhotra
et al 2022).
3 CONCLUSION
This work acknowledges that addressing future
challenges will necessitate collaboration between the
research community and industry. Techniques such as
transfer learning and continual learning will play a
crucial role in adapting AI models to effectively tackle
evolving threats. Furthermore, integrating AI with
human expertise to develop AI-powered collaborative
defense systems is likely to be the future approach.
Highlighting the importance of explainable AI in
network security will be paramount. AI models need
to be comprehensible and interpretable to establish
trust and enhance their effectiveness. Maintaining a
harmonious equilibrium between AI autonomy and
human supervision is vital for the development of
future AI-driven detection systems.
REFERENCES
Y. Li et al. 2021 Robust Online Learning against Malicious
Manipulation and Feedback Delay With Application
to Network Flow Classification.
N. Shone, T. N. Ngoc, V. D. Phai and Q. Shi, 2018. A Deep
Learning Approach to Network Intrusion Detection, in
IEEE Transactions on Emerging Topics in
Computational Intelligence, vol. 2, no. 1, pp.4150.
C. Radhika, and S. Shah. Detection of malicious network
traffic using convolutional neural networks. 2019 10th
International Conference on Computing,
Communication and Networking Technologies
(ICCCNT). IEEE (2019).
D. Kwon et al. A survey of deep learning-based network
anomaly detection. Cluster Comput 22 (Suppl 1), 949–
961 (2019).
P. Kaushik. Unleashing the Power of Multi-Agent Deep
Learning: Cyber-Attack Detection in IoT.
DAML 2023 - International Conference on Data Analysis and Machine Learning
324