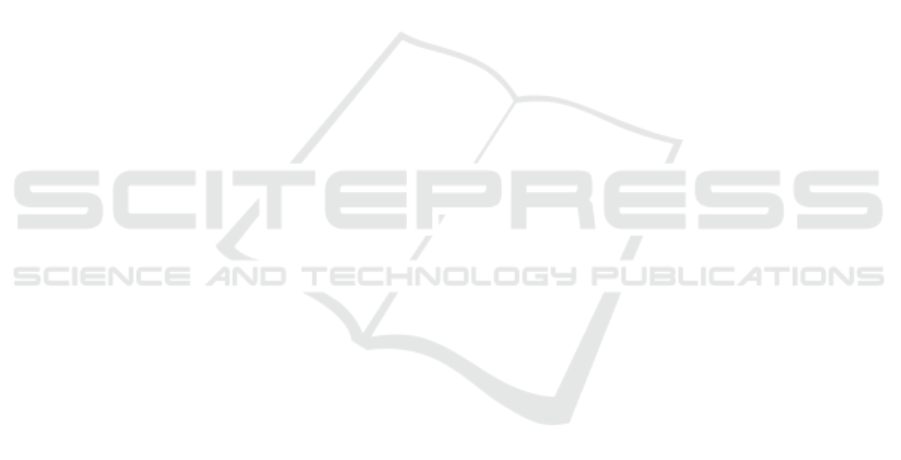
Analysis of Common Indicators and Unidentified Factors of Heart
Disease Based on Two Machine Learning Models
Jincheng Guo
School of Science, China University of Petroleum (East China), Qingdao, China
Keywords: Heart Disease, Unidentified Factors, Logistic Regression, Random Forest.
Abstract: In recent years, heart disease had caused great attention in the medical and health field. Many researchers
continuously care about common key indicators that directly related to heart disease. However, some
researchers have found that some unidentified non-direct indicators were also potential factors that affect
early heart disease. Therefore, the research theme in this paper is the impact of multiple direct and indirect
indicators on the prevalence of heart disease. And research method is downloading a large data set from
Kaggle website, which includes 18 variables and 320 thousand samples, before using logistic regression
model and random forest model to perform categorical prediction. It is found that the random forest model
performs very excellent in the training set, but the comprehensive classification effect on the logistic
regression model turns out to be better. Through analysis of these model results, it showed that in addition to
well-known indicators such as age and physical health, whether a person have diabetes, stroke, asthma or
some other indirect illnesses would also affect whether that person suffer from heart disease. Hence, the
prevention and treatment of heart disease patients should start from the early stage of other minor diseases
and potential latent factors, and patients should take their physical and psychological state seriously in a
comprehensive assessment.
1 INTRODUCTION
As early as the beginning of the last century, people
have taken emphasis on heart disease’s
seriousness. Surprisingly, about 17.5 million deaths
all around the world each year was caused by heart
disease and its complications, accounting for 1/3 of all
deaths (Liu and Qiao 2019). It is reported that there
were 300 million people in total suffering from heart
disease in China, and the number of hospitalizations
for heart disease has increased fourfold in the past 10
years (Tian et al 2019). Compared to Western
countries, where heart disease patients are people over
the age of 70, the patients in China whose age was 40
to 64 account for a large proportion. Firstly, this is due
to the fact that with the development of China's
economy, people are living more and more
prosperously, and their diet is biased towards high
cholesterol, heavy oil and salt. Secondly, the pressure
of work and life for modern people is numerous, but
their diet and rest are irregular (Qun et al 2016).
Thirdly, some diseases are asymptomatic or mild. If
latent patients cannot be screened and detected in time,
they may have further deterioration of the condition.
Additionally, some large gaps between China's
medical level and that of advanced countries are still
existing, as well as the problem of uneven economic
level between different areas. Therefore, the detection
and treatment of heart disease has become an urgent
issue on Chinese people and has become the focus of
attention in the medical field (Qun et al 2016).
At present, most medical institutions still perform
the detection of heart disease according to doctors'
personal experience and physical examination
results. It not only costs a lot on labor, but also delays
the optimal treatment time of patients. However, using
machine learning prediction methods as an auxiliary
diagnosis to provide effective guidance for clinical
diagnosis is a great way to improve the accuracy of
prediction and diagnosis (Yang et al 2016). Since the
technology convergence in this big data era is
commonplace nowadays, using machine learning to
contribute to the diagnosis and prediction of heart
disease will be a valuable and meaningful studying
(Liu and Qiao 2019). Machine learning takes
advantages of computers to build probabilistic
mathematical models on the basis of given data and
utilize these models to predict and analyze (Wang
2018).
Scholars all over the world have carried out
numerous research on the use of machine learning
Guo, J.
Analysis of Common Indicators and Unidentified Factors of Heart Disease Based on Two Machine Learning Models.
DOI: 10.5220/0012805200003885
Paper published under CC license (CC BY-NC-ND 4.0)
In Proceedings of the 1st International Conference on Data Analysis and Machine Learning (DAML 2023), pages 315-320
ISBN: 978-989-758-705-4
Proceedings Copyright © 2024 by SCITEPRESS – Science and Technology Publications, Lda.
315