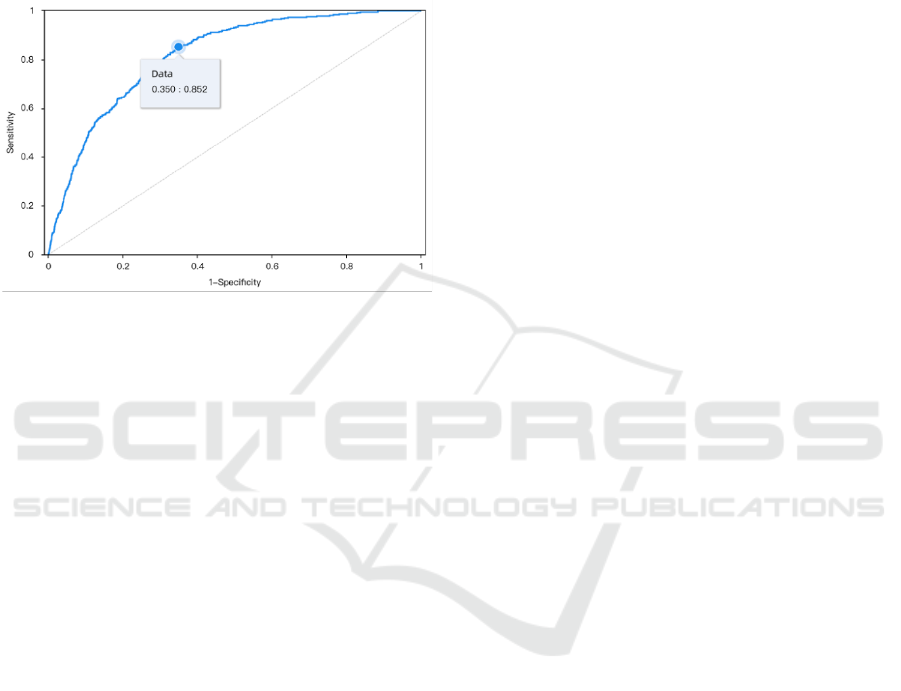
the proportion of subjects with heart disease and
subjects without it in the dataset used. When there are
too few subjects with heart disease in the dataset, it is
easy to obtain high accuracy by classifying more
subjects possible under the no-heart-disease category.
Therefore, accuracy is not a reasonable indicator to
evaluate the quality of the logit model if the purpose is
to identify heart disease carriers.
Figure 7: ROC Curve of Logit Regression Model (Picture
credit: Original).
Sensitivity is the accuracy when the true value is 1,
and Specificity is the accuracy when the true value is
0. When the goal is to identify as many heart disease
carriers as possible, the Sensitivity should be high with
the Specificity at an acceptable level. According to
Fig.7, when the Sensitivity is over 0.85, Specificity is
0.65. The classification threshold is calculated to be
0.0628. It means when the threshold value is set to
0.0628, the model can identify over 85% of heart
disease carriers while misclassifying 35% of non-
carriers as heart disease carriers.
4 CONCLUSION
This study selected 4855 observations and 10 variables
from the dataset, focusing on possible influencing
factors related to the development of heart disease. A
random forest model and a binary logit model are
constructed respectively trying to predict whether the
subjects have heart diseases.
The random forest model has a total accuracy of
86% and an AUC value of 0.88, indicating it can be
considered an effective model. However, the
prediction accuracy of heart disease carriers is
significantly lower than that of non-carriers, which
might be resulted from the proportion difference
between carriers and non-carriers in the dataset.
Increasing the sample size may also help to improve
accuracy.
The logit model has a total accuracy of 92% and an
AUC value of 0.82, proving its effectiveness.
However, the prediction accuracy of heart disease
carriers and non-carriers also have a huge difference
because of the proportion difference in the dataset.
Therefore, the ROC curve is introduced to adjust the
accuracy. By choosing an appropriate threshold value,
the accuracy of identifying heart disease carriers can
be improved at the cost of sacrificing the prediction
accuracy of non-carriers.
By comparison, the random forest model and the
logit regression model both have the ability to identify
heart disease carriers and non-carriers. However, the
logit regression model can better interpret the
relationship between variables and the risk of having a
heart disease. Adjusting threshold value can also help
the logit model to fit different needs.
It is undeniable that due to the limited amount of
data collected, the models may have errors. The
samples only cover a certain population, which may
cause some differences and affect the accuracy of the
results. Limited by the knowledge level of the authors,
this paper cannot better interpret and improve the
model. However, this paper proposes possible methods
to predict and screen potential heart disease carriers,
hoping to provide inspiration and ideas for clinicians
and future studies.
4.1 Authors Contribution
All the authors contributed equally, and their names
were listed in alphabetical order.
REFERENCES
C. C. Qin, “Research on Heart Disease Prediction Based on
Catboost Model,” Qufu Normal University, 2023.
F. Zhang, et al, “Analysis of Heart Disease Mortality Trends
among Residents of Death Cause Monitoring Points in
Hebei Province from 2014 to 2019,” China Public
Health Journal, vol. 38(03), pp. 351-355, 2022.
Q. Wei, et al, “Joinpoint regression analysis of heart disease
mortality trends in urban and rural China from 2004 to
2019,” Chinese Journal of Cardiology, vol. 27(04), pp.
371-376, 2022.
S. S. Hu and Z. W. Wang, “Overview of China
Cardiovascular Health and Disease Report 2022,” China
Cardiovascular Disease Research, vol. 21(07), pp. 577-
600, 2023.
D. M. Li, “A natural vegetable and fruit diet may offset the
impact of genetic factors on heart disease,” Knowledge
of Cardiovascular Disease Prevention and Control
(Science Popularization Edition), vol. 05, p. 66, 2012.
DAML 2023 - International Conference on Data Analysis and Machine Learning
96