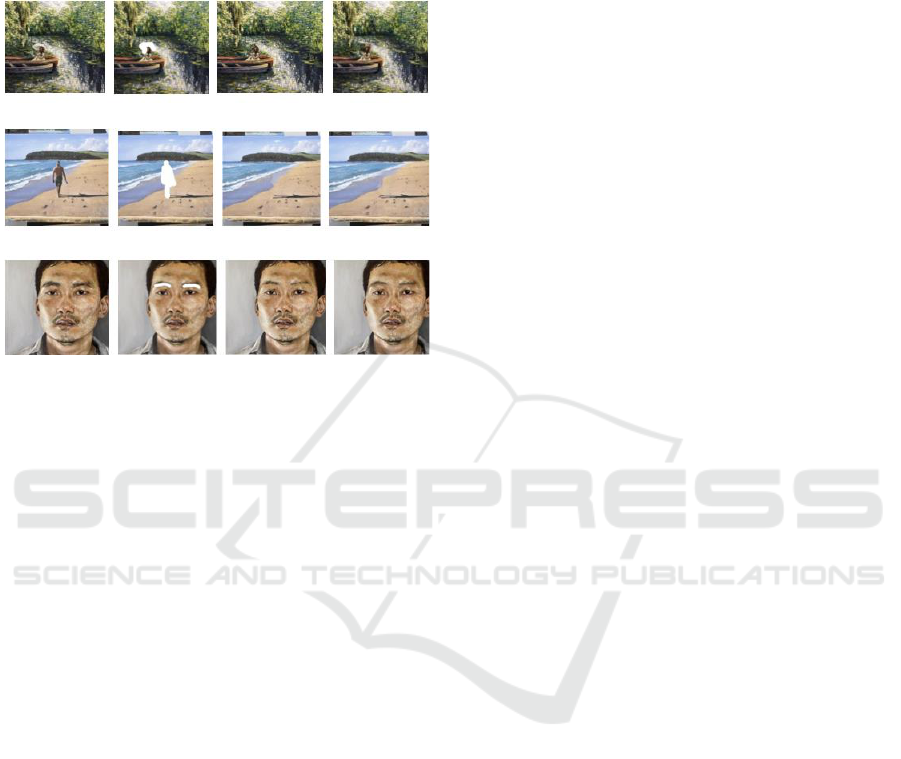
et al 2018 & Liu et al 2018). Figure 4 displays the
results of automatic restoration on several
representative images. Displayed from left to right: the
raw image, the masked image, and the result of the
model using spatial convolution and gated convolution.
Figure 4: Comparison of the outcome of spatial and gated
convolution approaches (Picture credit: Original).
By comparing these two approaches, it is
noticeable that partial convolution produces better
results but still exhibits observable color discrepancies.
However, the approach based on gated convolutions
achieves more visually pleasing results without
significant color inconsistencies.
To summarize, by quantitatively comparing several
commonly used image restoration methods with the
approach introduced in this paper, it can be concluded
that the model using gated convolution has
significantly lower loss rates than other methods.
Regarding the image restoration results, partial
convolution, unlike vanilla convolution, does not
exhibit obvious visual artifacts and edge responses
within holes or around holes, but it still shows
noticeable color discrepancies. In contrast, the gated
convolution-based approach largely overcomes this
issue, producing more realistic output results.
4 CONCLUSION
This study presents a groundbreaking approach to the
restoration of oil painting images by integrating gated
convolutions and the SN-PatchGAN discriminator.
Traditional inpainting methods have long struggled
with limitations when dealing with diverse hole shapes
and multi-channel inputs, often yielding unrealistic or
subpar results. However, this innovative technique
offers a solution to these challenges, enabling the
restoration of oil paintings with remarkable realism
and high quality.
Gated convolutions are at the core of this approach,
introducing dynamic feature selection mechanisms for
each channel and spatial position. This significantly
enhances color uniformity and inpainting performance,
ensuring that the restored images are both faithful to
the original artwork and aesthetically pleasing. This is
a crucial advancement as it addresses a critical issue in
image restoration, particularly when dealing with free-
form masks that are common in the world of art
conservation.
The SN-PatchGAN discriminator complements the
process by streamlining the training phase, making it
more efficient and robust. It simplifies the loss function,
resulting in a more straightforward yet effective
approach. The combination of gated convolutions and
SN-PatchGAN is a novel technique in the field of
image restoration. It not only significantly improves
inpainting quality but also opens up new possibilities
for various oil painting restoration tasks.
This research plays a vital role in preserving
cultural heritage, revitalizing the art market, advancing
educational and research endeavors, and safeguarding
personal memories. Furthermore, it fosters artistic
innovation by providing artists and restorers with
powerful tools to breathe new life into old artworks.
Looking ahead, this research can serve as a
foundation for further exploration within the realm of
image restoration, inspiring new approaches and
innovations to meet the evolving needs of art
conservation and digital image processing.
REFERENCES
J. Yu, Z. Lin, J. Yang, et al. “Free-form image inpainting
with gated convolution,” Proceedings of the IEEE/CVF
international conference on computer vision, 2019, pp.
4471-4480.
J. H. Dewan, S. D. Thepade, “Image retrieval using low
level and local features contents: a comprehensive
review,” Applied Computational Intelligence and Soft
Computing, 2020, pp. 1-20.
S. Iizuka, E. Simo-Serra, H. Ishikawa, “Globally and locally
consistent image completion,” ACM Transactions on
Graphics (ToG), vol. 36, 2017, pp. 1-14.
Y. Song, C. Yang, Z. Lin, et al. “Contextual-based image
inpainting: Infer, match, and translate,” Proceedings of
the European conference on computer vision (ECCV),
2018, pp. 3-19.
C. Li, M. Wand. “Precomputed real-time texture synthesis
with Markovian generative adversarial networks,”
Computer Vision–ECCV 2016: 14th European
Conference, Amsterdam, 2016, pp. 702-716.
DAML 2023 - International Conference on Data Analysis and Machine Learning
496