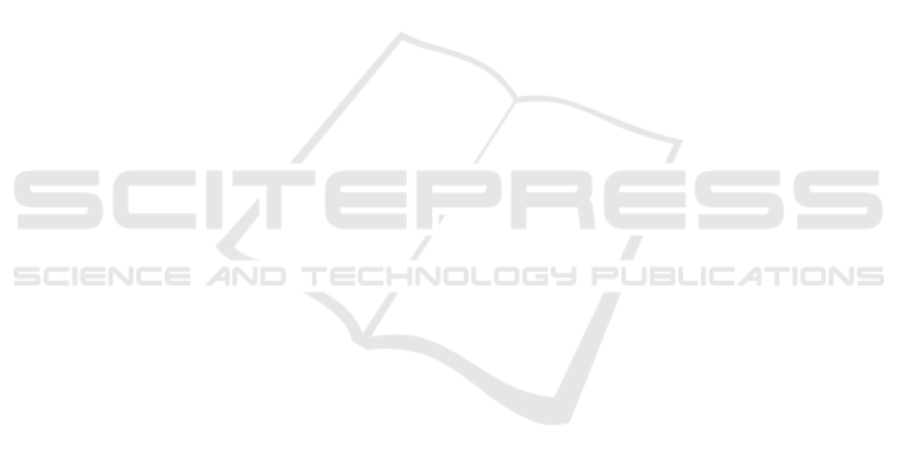
Additionally, the study highlights the importance
of avoiding a one-size-fits-all approach in
business strategies, emphasizing the need for
personalized approaches enabled by accurate
patron fractionation.
5. Overall Recommendations: Based on the
findings, it is recommended to utilize the novel K-
Means clustering method for patron fractionation
in online social networks due to its superior
accuracy compared to the EM clustering
technique. This recommendation is supported by
the statistical significance of the results and the
potential business benefits associated with more
precise patron segmentation. In conclusion, the
study provides valuable insights into the
effectiveness of different clustering methods for
patron fractionation, highlighting the importance
of accurate data analysis in optimizing business
strategies and resource allocation in cloud
infrastructure management.
REFERENCES
Tabianan et.al, Kayalvily et.al, Shubashini Velu et.al, and
Vinayakumar Ravi. 2022 et.al. “Novel K-Means
Clustering Approach for Intelligent patron fractionation
Using patron Purchase Behavior Data.” Sustainability.
https://doi.org/10.3390/su14127243.
Sivaguru, M. 2022 et.al. “Dynamic patron fractionation: A
Case Study Using the Modified Dynamic Fuzzy c-
Means Clustering Algorithm.” Granular Computing.
https://doi.org/10.1007/s41066-022-00335-0.
G. Ramkumar, R. Thandaiah Prabu, Ngangbam Phalguni
Singh, U. Maheswaran, Experimental analysis of brain
tumor detection system using Machine learning
approach, Materials Today: Proceedings, 2021, ISSN
2214-7853, https://doi.org/10.1016/j.matpr.2021.01.246.
Lefait et al, Guillem et al, and Tahar Kechadi. 2010 et al.
“Patron fractionation Architecture Based on Clustering
Techniques.” 2010 Fourth International Conference on
Digital Society. https://doi.org/10.1109/icds.2010.47.
Pradhan et al, Rahul. 2021 et al. “Patron fractionation Using
Clustering Approach Based on RFM Analysis.” 2021
5th (ISCON).
https://doi.org/10.1109/iscon52037.2021.9702482.
Liu et al, Chaohua. 2011 et al. “patron fractionation and
Evaluation Based on RFM, Cross-Selling and patron
Loyalty.” 2011 ICMSS.
https://doi.org/10.1109/icmss.2011.5998805.
Barga et.al, Roger et.al, Valentine Fontama et.al, and Wee
Hyong Tok. 2015 et.al. “Patron fractionation Models.”
Predictive Analytics with Microsoft Azure Machine
Learning. https://doi.org/10.1007/978-1-4842-1200-
4_10
Hossain et.al, A. S. M. Shahadat et.al, and A. S. Shahadat
Hossain. 2017 et.al. “patron fractionation Using
Centroid Based and Density Based Clustering
Algorithms.” 2017 3rd (EICT).
https://doi.org/10.1109/eict.2017.8275249.
Seybold et.al, Patricia. 2002 et.al. “Designing a patron
Flight Deck (SM) System - patron fractionation.”
https://doi.org/10.1571/fw1-31-02cc.
Santana et.al, Clodomir J. et.al, Pedro Aguiar et.al, and
Carmelo J. A et.al. Bastos-Filho et.al. 2018. “Patron
Fractionation in a Travel Agency Dataset Using
Clustering Algorithms.” 2018 IEEE(LA-CCI).
https://doi.org/10.1109/la-cci.2018.8625252.
Zakrzewska, D et al., and J. Murlewski 2005 et. al.
“Clustering Algorithms for Bank patron fractionation.”
(ISDA’05). https://doi.org/10.1109/isda.2005.33.
Sivakumar, V. L., Nallanathel, M., Ramalakshmi, M., &
Golla, V. (2022). Optimal route selection for the
transmission of natural gas through pipelines in
Tiruchengode Taluk using GIS–a preliminary study.
Materials Today: Proceedings, 50, 576-581..
Syaputra et al, Aldino et al, Zulkarnain et al, and Enrico
Laoh. 2020 et al. “patron fractionation on Returned
Product patrons Using Time Series Clustering
Analysis.” 2020(ICISS).
https://doi.org/10.1109/iciss50791.2020.9307575.
Garca, Kimberly, and Antonio Santos-Silva. 2022. “New
Species and New Records in Neoibidionini and
Hexoplonini (Coleoptera: Cerambycidae:
Cerambycinae).” Zootaxa 5134 (3): 399–414.
James, J., Lakshmi, S. V., & Pandian, P. K. (2017). A
preliminary investigation on the geotechnical properties
of blended solid wastes as synthetic fill material.
International Journal of Technology, 8(3), 466-476.
Hax, Arnoldo C. 2010. “Customer Segmentation and
Customer Value Proposition: The First Critical Task of
Strategy.” The Delta Model.
https://doi.org/10.1007/978-1-4419-1480-4_3.
Xue et.al, Mengfan et.al, Lu Han et.al, Yiran Song et.al, Fan
Rao et.al, and Dongliang Peng. 2022 et.al. “A Fissure-
Aided Registration Approach for Automatic Pulmonary
Lobe fractionation Using Deep Learning.” Sensors 22
(21). https://doi.org/10.3390/s22218560.
Pramono, Pradnya Paramita, Isti Surjandari, and Enrico
Laoh. 2019. “Estimating Customer Segmentation
Based on Customer Lifetime Value Using Two-Stage
Clustering Method.” 2019 16th International
Conference on Service Systems and Service
Management (ICSSSM).
https://doi.org/10.1109/icsssm.2019.8887704.
Resource Load Balancing on Cloud Infrastructure for Subscriber Management in Comparison with Raw Unbalanced Data for Calculation of
Energy Consumption
359