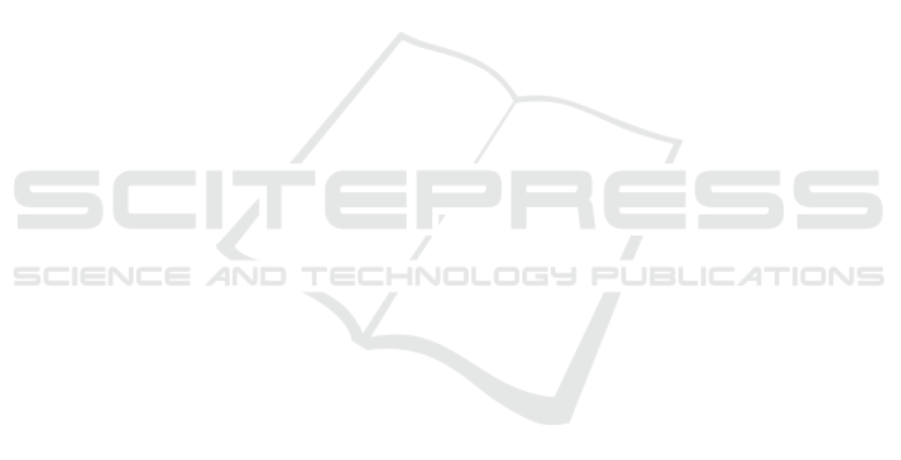
Fed Avg, Moreover, produces poor convergence
and introduces gradient biases into model
aggregation, according to Yao et al. (Yao et al 2019).
Fed Avg requires 1400 epochs (280 synchronization
cycles) to accomplish 80% sorting precision on the
dataset, whereas SGD only needs 36 epochs [2]. Fed
Avg-based algorithms are not recommended for the
domain under the FL in that case we may able to
communicate with any interrupt under the key feature
of bottleneck, due to the disadvantage.
The huge rate of recurrence could be coordinated
with SSGD that is based on the algorithms chosen by
the domain within the range of FL due to their higher
conjunction and lack of communication bottleneck.
However, the main constraint is the significant
computational heterogeneity. There is collection in
the collective information Centre since the computers
donated by dissimilar gatherings have computation
strategies with varying authority and it is expensive
and difficult to exchange all the outdated
technologies. Because straggler machines will block
powerful technologies in every single organization up
till the barricade is grasped, heterogeneity results in
significant inefficiency.
Asynchronous and synchronous approaches can
be used to solve the straggler problem. The
coordinated gathering shave a tendency to be
standardized. since synchronous approaches choose
participants with comparable processing capabilities.
Models supplied within a predetermined time
frame were accepted by Bonawitz et al. (Zinkevich et
al 2010), but timeout models from lagging parties
were rejected. Chai et al.'s (Zinkevich et al 2010)
division of parties into many tiers with uniform
processing power allowed them to choose one tier for
synchronization based on chance. These techniques
impair the generalization of the global model and
make it harder for lone parties to contribute their
models.
Asynchronous and synchronous approaches can
be used to solve the straggler problem. Coordinated
gatherings have a habit of to be standardized since
synchronous approaches choose participants with
comparable processing capabilities.
This study provides an effective synchronization
technique which could able to evade the obstructive
brought on by dawdlers in order to lecture the
dawdlers in the very assorted domain in the range of
FL while retaining accurateness without any loss of
data.
The fundamental concept is to encourage
powerful parties to meet the required that has to be
trained as per many repetitions as they can previously
lagging gatherings finish an repetition, allowing
authoritative gatherings to discover advanced
excellence copies through the obstructive time.
Number of local iterations for each party must be
adaptively coordinated via an online scheduling
method in order to realism this concept. The
following is a summary of this paper's contributions:
• In our new FL proposal, called the domain
within the range on FL, the gatherings work together
to set the ML models in a collective information
Centre with significant computational heterogeneity.
We compare the proposed intra-domain.
• To synchronize the speed of all gatherings, we
suggest a novel scheduler State Server. By State
Server, which may also update scheduling choices in
response to changing circumstances.
• For strongly heterogeneous situations, we
suggest the effective synchronization technique
Essynce. Essynce, which is coordinated by State
Server, enables gatherings to train numerous
repetitions nearby depending on their possessions,
resolving the dawdler issue & quickening the working
out procedure.
2 RELATED WORKS
Stragglers occur in both FL and conventional
machine learning algorithm which is not in the
information on only in the present FL because of the
information separation. We summarize the many
approaches that have been suggested to deal with the
problems provided by straggler.
2.1 Cross-Device and Cross-Silo FL
The most popular federated optimization approach,
called synchronous Fed Avg, requires that all parties
grasp the limited representations for synchronizing
their limited representations. The assortment of
calculating hardware, encourages the appearance of
dawdlers, that results in lengthy obstructive period,
severe training inefficiency, and resource waste.
Some techniques use deadlines and time limitations
to weed out stragglers. First M models were approved
by Bonawitz et al. (Krichevsky et al 2009) but
timeout models from stragglers (Fed Drop) were
refused.
Parties were able to provide numerous repetitions
in the vicinity throughout the predetermined period
space. Parties have until the deadline to upload their
local models, according to Rafizadeh et al. (Coates et
al 2013).
Non-I.I.D.
Accelerating Federated Learning Within a Domain with Heterogeneous Data Centers
345