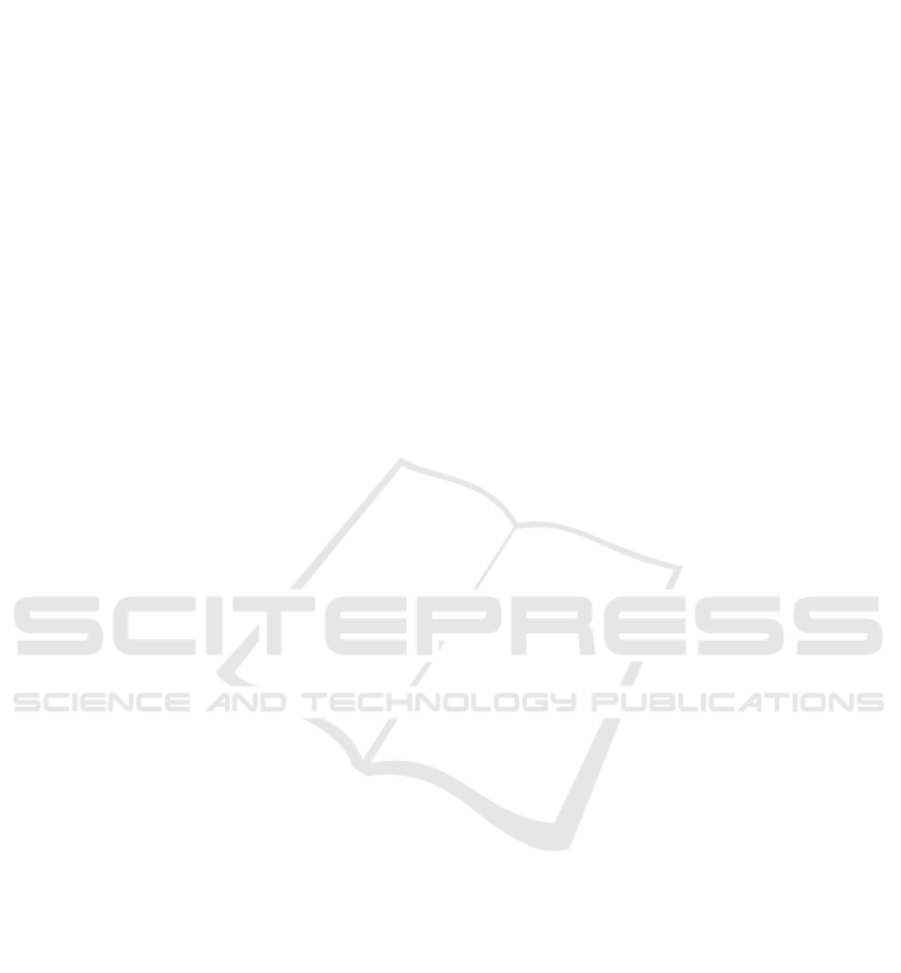
data set (Freitas, Silva, and Silva, 2021). The main
goal of pattern recognition is to accurately classify an
input pattern as one of the several output classes. The
two main steps of this approach are feature selection
and classification (Politikos, 2021). Since the
classifier won't be able to distinguish between badly
chosen features, feature selection is essential to the
entire process (Kikaki et al., 2022). This research
application can be used in classifying the Debris on
Ocean surfaces and shores. A few other applications
include with the help of this approach the amount of
garbage that is mixed with water bodies is determined
and also used to classify the debris in the ocean
(Vethaak, 2022).
The factors affecting the study include the
collection of datasets from various sources. Another
factor that affects the study is the time required to
classify the data for both classification and detection.
The limitation of this study, the debris present below
the surface cannot be determined due to the lack of
light below the surface. The future scope of this study
includes the way to introduce hybrid algorithms to
improve accuracy. The future scope of this study is
that the system should be expanded to include a larger
number of objects with lesser time consumption in
training the data set.
6 CONCLUSION
The Aquatic Debris Recognition Data set was used to
improve the performance of image classification. The
study employed the Novel AlexNet algorithm and
DenseNet for this purpose. The mean accuracy of the
Novel AlexNet algorithm was found to be 93.77%,
whereas that of DenseNet was 92.91%. It can be
inferred that the Novel AlexNet algorithm yields
higher accuracy than the DenseNet algorithm.
REFERENCES
Alom, Md Zahangir, Tarek M. Taha, Christopher Yakopcic,
Stefan Westberg, Paheding Sidike, Mst Shamima
Nasrin, Brian C. Van Esesn, Abdul A. S. Awwal, and
Vijayan K. Asari, 2021. “The History Began from
AlexNet: A Comprehensive Survey on Deep Learning
Approaches.” http://arxiv.org/abs/1803.01164.
Chen, Ge., 2022. “Deep-Sea Debris Identification Using
Deep Convolutional Neural Networks.” 2022.
https://doi.org/10.1109/JSTARS.2021.3107853.
Freitas, Sara, Hugo Silva, and Eduardo Silva, 2021.
“Remote Hyperspectral Imaging Acquisition and
Characterization for Marine Litter Detection.” Remote
Sensing 13 (13): 2536.
G. Ramkumar, R. Thandaiah Prabu, Ngangbam Phalguni
Singh, U. Maheswaran, 2021, Experimental analysis of
brain tumor detection system using Machine learning
approach, Materials Today: Proceedings, ISSN 2214-
7853, https://doi.org/10.1016/j.matpr.2021.01.246.
Huang, Baoxiang. 2021. “An Efficient Deep-Sea Debris
Detection Method Using Deep Neural Networks”,
2021. https://doi.org/10.1109/JSTARS.2021.3130238.
James, J., Lakshmi, S. V., & Pandian, P. K., 2017. A
preliminary investigation on the geotechnical properties
of blended solid wastes as synthetic fill material.
International Journal of Technology, 8(3), 466-476.
Kang, Kai, Hongsheng Li, Junjie Yan, Xingyu Zeng, Bin
Yang, Tong Xiao, Cong Zhang, et al., 2022. “T-CNN:
Tubelets with Convolutional Neural Networks for
Object Detection from Videos.” IEEE Transactions on
Circuits and Systems for Video Technology 28 (10):
2896–2907.
Kishore Kumar, M. Aeri, A. Grover, J. Agarwal, P. Kumar,
and T. Raghu, “Secured supply chain management
system for fisheries through IoT,” Meas. Sensors, vol.
25, no. August 2022, p. 100632, 2023, doi:
10.1016/j.measen.2022.100632.
Kikaki, Katerina, Ioannis Kakogeorgiou, Paraskevi Mikeli,
Dionysios E. Raitsos, and Konstantinos Karantzalos,
2022. “MARIDA: A Benchmark for Marine Debris
Detection from Sentinel-2 Remote Sensing Data.” PloS
One 17 (1): e0262247.
Li, Ya-Li, and Shengjin Wang, 2022. “BooDet: Gradient
Boosting Object Detection with Additive Learning-
Based Prediction Aggregation.” IEEE Transactions on
Image Processing: A Publication of the IEEE Signal
Processing Society 31 (March): 2620–32.
Marin, Ivana, Saša Mladenović, Sven Gotovac, and Goran
Zaharija. 2021. “Deep-Feature-Based Approach to
Marine Debris Classification.” NATO Advanced
Science Institutes Series E: Applied Sciences 11 (12):
5644.
Politikos, Dimitris V., 2021. “Automatic Detection of
Seafloor Marine Litter Using Towed Camera Images
and Deep Learning.” Marine Pollution Bulletin 164
(March): 111974.
Sivakumar, V. L., Nallanathel, M., Ramalakshmi, M., &
Golla, V., 2022. Optimal route selection for the
transmission of natural gas through pipelines in
Tiruchengode Taluk using GIS–a preliminary study.
Materials Today: Proceedings, 50, 576-581.
Vethaak, Dick, 2022. “Marine Microplastic Debris: An
Emerging Issue for Food Security, Food Safety and
Human Health.” Marine Pollution Bulletin 133
(August): 336–48.
V. P. Parandhaman, "An Automated Efficient and Robust
Scheme in Payment Protocol Using the Internet of
Things," (2023) Eighth International Conference on
Science Technology Engineering and Mathematics
(ICONSTEM), Chennai, India, 2023, pp. 1-5, doi:
10.1109/ICONSTEM56934.2023.10142797.
Wang, Gaihua, Zhao Guo, Xizhou Wan, and Xu Zheng.
2021. “Study on Image Classification Algorithm Based
Comparison of AlexNet Algorithm with DenseNet Algorithm for Aquatic Debris Detection on Ocean Surfaces
371