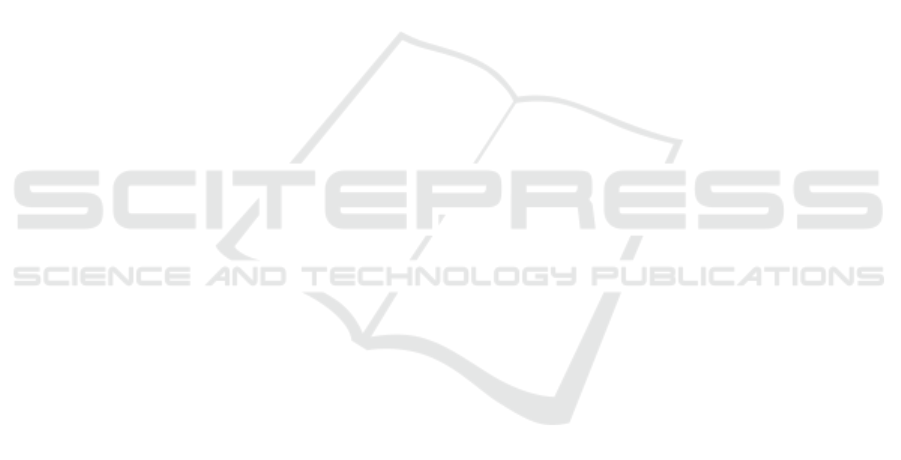
98.95%, Linear Discriminant Analysis 91.01%, K-
Nearest Neighbors 98.73%, Classification and Re-
gression Tree 99.15% and Na
¨
ıve Bayes 98.53%. The
test results show that the Random Forest algorithm
produces the highest accuracy value compared to sev-
eral other methods.
As noted, the research does not aim to replace the
plant disease diagnosis process carried out by experts
but only seeks to complement it. The existence of
machine learning methods can only predict with the
level of accuracy by the accuracy obtained; still, test-
ing through the laboratory is the most reliable way to
diagnose plant diseases. However, the model imple-
mentation is used to help smallholders who need help
to get a quick response from experts.
For further research, other feature extraction can
be applied, which is not just one type; a feature extrac-
tion combination sees the difference in performance
results in the extraction process. If there are larger
data, it can be continued using deep learning and im-
plemented in the system.
ACKNOWLEDGMENTS
The author would like to thank all parties who
have supported this research process. The author
also thanks Siddharth Singh Chouhan, Uday Pratap
Singh, Ajay Kaul, and Sanjeev Jain for publishing the
Pongamia Pinnata Leaf Image Data Repository
REFERENCES
A. Aminah, Supriyanto, I. Z. and A. Suryani, P. J. S. S.
B. B. B. C. M. P. L. (2017). Pierre from java is-
land as biodiesel raw material. J. Penelit. Has. Hutan,
35(4):255-262.
Agustiani, S., Arifin, Y., Junaidi, A., Wildah, S., and
Mustopa, A. (2022). Klasifikasi penyakit daun padi
menggunakan random forest dan color histogram.
Balyan, A. (2022). A hybrid intrusion detection model us-
ing ega-pso and improved random forest method. Sen-
sors, 22(16):1-20.
Beauty, C. and Sari, Y. (2019). Temu kembali citra makanan
menggunakan color histogram dan local binary pat-
tern. J. Pengemb. Teknol. Inf. dan Ilmu Komput,
3(6):5514-5520.
Chen, H. (2022). Establishment and analysis of a dis-
ease risk prediction model for the systemic lupus
erythematosus with random forest. Front. Immunol,
13(November): 1-13.
Chouhan, S., Singh, U., Kaul, A., and Jain, S. (2019). A
data repository of leaf images: Practice towards plant
conservation with plant pathology. Conf. Inf. Syst.
Comput. Networks, ISCON, pages 700-707.
Chouhan, S., Singh, U., Sharma, U., and Jain, S. (2021).
Leaf disease segmentation and classification of jat-
ropha curcas l. and pongamia pinnata l. biofuel plants
using computer vision based approaches. Meas. J. Int.
Meas. Confed, 171:108796.
Dendang, B. and Suhaendah, E. (2017). Uji efektivitas in-
sektisida terhadap hama maruca testulalis pada bibit
malapari (pongamia pinnata (l.) pierre. J. Pemuliaan
Tanam. Hutan, 11(2):123-130.
Evans, M. (2021). Pongamia: Manfaat-manfaat potensial
untuk restorasi dan bioenergi di indonesia. accessed
Jan. 15, 2023).
Gandhi, F. and Lina, L. (2021). Pengenalan jenis maker
dengan metode color histogram dan euclidean dis-
tance. J. Ilmu Komput. dan Sist. Inf, 9(2):18.
Khasanah, F. (2020). Pemanfaatan media sosial dan ecom-
merce sebagai media pemasaran dalam mendukung
peluang usaha mandiri pada masa pandemi covid 19.
J. Sains Teknol. dalam Pemberdaya. Masy, 1(1):51-
62.
Kusnandar, V. (2022). Indonesia impor minyak 30 juta ton
pada januari-september 2022. accessed Jan. 15, 2023).
Li, X., Chen, W., Zhang, Q., and Wu, L. (2020). Build-
ing auto-encoder intrusion detection system based
on random forest feature selection. Comput. Secur,
95:101851.
Maniyath, S. (2018). Plant disease detection using machine
learning. Conf. Des. Innov. 3Cs Comput. Commun.
Control, (April):41-45.
Maretta, A. T. D., Devy, L., and Sulastri (2016). Pro-
siding seminar nasional dan kongres perhimpunan
agronomi Indonesia 2016. Mult. Tunas Vitr. Satoimo
(Colocasia esculenta Scott Var Antiq. pada Media MS
dengan Penambahan 2iP, Glutamin, GA3, BAP, dan
NAA,(May):173-178.
Mitra, S. (2021). A review on environmental and socioeco-
nomic perspectives of three promising biofuel plants
jatropha curcas, pongamia pinnata and mesua ferrea.
Biomass and Bioenergy, 151:106173.
More, P., Agarwal, P., and Agarwal, P. (2019). Gem-
iniviruses: Molecular biodiversity and global dis-
tribution in jatropha. Physiol. Mol. Plant Pathol,
108(May):101439,.
Murugan, A., Nair, S., and Kumar, K. (2019). Detection of
skin cancer using svm, random forest and knn classi-
fiers. J. Med. Syst, 43(8).
Orte, F., Mira, J., S
´
anchez, M., and Solana, P. (2023-01). A
random forest-based model for crypto asset forecasts
in futures markets with out-of-sample prediction. Res.
Int. Bus. Financ, 64:101829.
Primajaya, A. and Sari, B. (2018). Random forest algorithm
for prediction of precipitation. Indones. J. Artif. Intell.
Data Min, 1(1):27-31.
Purwanto, A., Wikantika, K., Deliar, A., and Darmawan, S.
(2023). Decision tree and random forest classification
algorithms for mangrove forest mapping in sembilang
national park, Indonesia. Remote Sens, 15(1).
Radhiana (2023). Strategi keberlanjutan pembangunan
energi terbarukan jangka panjang indonesia: Kasus
Leaf Disease Detection Using Color Histogram and Random Forest on Pongamia Pinnata (L.) Pierre
147