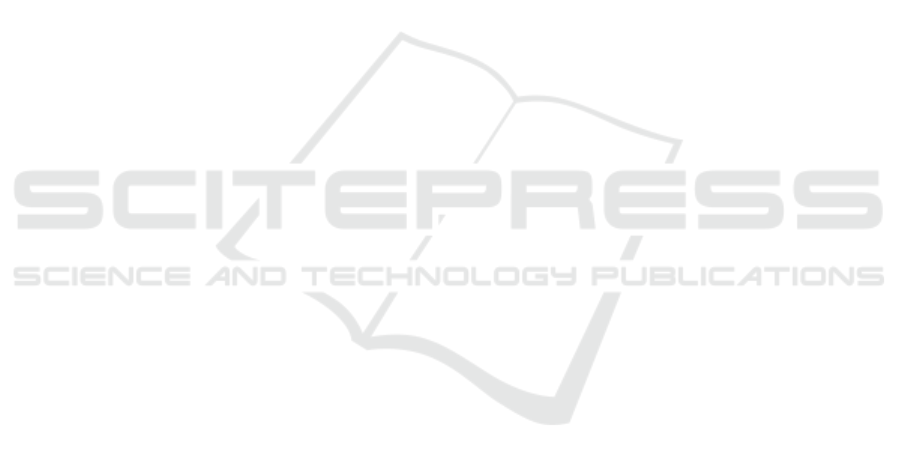
used as a reference in observing decisions made based
on the category of damage that occurs and is tested
by the system. Implementation of the best method
for the accuracy of asphalt damage identification re-
sults is made into a web-based application that can be
run offline or online. Based on the overall accuracy
test the results of the decision tree method show an
accuracy value of 98.6% and the KNN method gets
an overall accuracy value of 74.07%. This result is
obtained from the category of heavy damage to as-
phalt. The types of asphalt damage carried out in the
tests were carried out using images of groove dam-
age, distortion, fatness, wear and tear, curl, holes, and
cracks. The comparison of classifiers results that the
decision tree classifier gives the best accuracy value
from KNN.
ACKNOWLEDGEMENTS
The researcher would like to thank all parties involved
in this research, this research can be a solution and
simplify the field survey process for damaged road
conditions so as to simplify and speed up calculations
in predicting the need for asphalt repair materials in
the field.
REFERENCES
Aparna, Y., Rai, R., Gupta, V., Aggarwal, N., and Akula,
A. (2022). Convolutional neural networks based pot-
holes detection using thermal imaging. Journal of
King Saud University - Computer and Information
Sciences, 34:578–588.
Arfani, M. (2021). Implementasi computer vision pada de-
teksi dini kebakaran area spbu berbasis segmentasi.
Azhar, K., Murtaza, F., Yousaf, M., and Habib, H. (2016).
Computer vision based detection and localization of
potholes in asphalt pavement images. Institute of
Electrical and Electronics Engineers Inc.
Chitale, P., Kekre, K., Shenai, H., Karani, R., and Gala, J.
(2020). Pothole detection and dimension estimation
system using deep learning (yolo) and image process-
ing. IEEE Computer Society.
Coulibaly, S., Kamsu-Foguem, B., Kamissoko, D., and
Traore, D. (2022). Deep convolution neural network
sharing for the multi-label images classification. Ma-
chine Learning with Applications, 10:100422.
Dompeipen, T., Sompie, S., and Najoan, M. (2021). Com-
puter vision implementation for detection and count-
ing the number of humans,”jurnal. Teknik Informatika,
16:65–67.
Feng, L., Zhang, L., Gao, Z., Zhou, R., and Li, L. (2023).
Gabor-yolonet: A lightweight and efficient detection
network for low-voltage power lines from unmanned
aerial vehicle images. Frontiers in Energy Research,
10.
Hendrawan, Y., Widyaningtyas, S., and Sucipto, S.
(2019). Computer vision for purity, phenol, and
ph detection of luwak coffee green bean,”telkomnika.
Telecommunication Computing Electronics and Con-
trol, 17:3073–3085.
Kurniawan, Y. and Barokah, T. (2020). Klasifikasi penen-
tuan pengajuan kartu kredit menggunakan k-nearest
neighbor. Jurnal Ilmiah Matrik, 22:73–82.
Leonardo, L. (2020). Penerapan metode filter gabor untuk
analisis fitur tekstur citra pada kain songket. Jurnal
Sistem Komputer dan Informatika (JSON, 1:120.
Li, Y., Bi, Y., Zhang, W., Ren, J., and Chen, J. (2022). Color
image edge detection using multi-scale and multi-
directional gabor filter.
Ma, X., Shakoor, M., Vasiukov, D., Lomov, S., and Park, C.
(2021). Numerical artifacts of fast fourier transform
solvers for elastic problems of multi-phase materials:
their causes and reduction methods. Computational
Mechanics, 67:1661–1683.
Oppong, S., Twum, F., Hayfron-Acquah, J., and Missah,
Y. (2022). A novel computer vision model for medici-
nal plant identification using log-gabor filters and deep
learning algorithms. Computational Intelligence and
Neuroscience.
Ouma, Y. and Hahn, M. (2017). Pothole detection on as-
phalt pavements from 2d-colour pothole images using
fuzzy c-means clustering and morphological recon-
struction. Automation in Construction, 83:196–211.
Permadi, D., Widianto, W., and Hudayat, Y. (2021). Anali-
sis kondisi permukaan perkerasan jalan dengan meng-
gunakan metode survey sdi dan rci serta penanganan-
nya.
Qudsi, N., Asmara, R., and Syulistyo, A. (2020). Identi-
fikasi citra tulisan tangan digital menggunakan con-
volutional neural network (cnn. Seminar Informatika
Aplikatif Polinema, page 48–53.
Riana, D., Rahayu, S., Hadianti, S., Frieyadie, F., Hasan,
M., Karimah, I., and Pratama, R. (2022). Identifikasi
citra pap smear repomedunm dengan menggunakan k-
means clustering dan glcm. Jurnal RESTI (Rekayasa
Sistem dan Teknologi Informasi, 6:1–8.
Singh, G. and Gupta, P. (2019). Performance analysis of
various machine learning-based approaches for detec-
tion and classification of lung cancer in humans. Neu-
ral Computing and Applications, 31:6863–6877.
Vijayarajeswari, R., Parthasarathy, P., Vivekanandan, S.,
and Basha, A. (2019). Classification of mammo-
gram for early detection of breast cancer using svm
classifier and hough transform. Measurement: Jour-
nal of the International Measurement Confederation,
146:800–805.
Waluyo, W., Isnanto, R., and Rochim, A. (2023). Compar-
ison of mycobacterium tuberculosis image detection
accuracy using cnn and combination cnn-knn. Jur-
nal RESTI (Rekayasa Sistem dan Teknologi Informasi,
7:80–87.
ICAISD 2023 - International Conference on Advanced Information Scientific Development
106