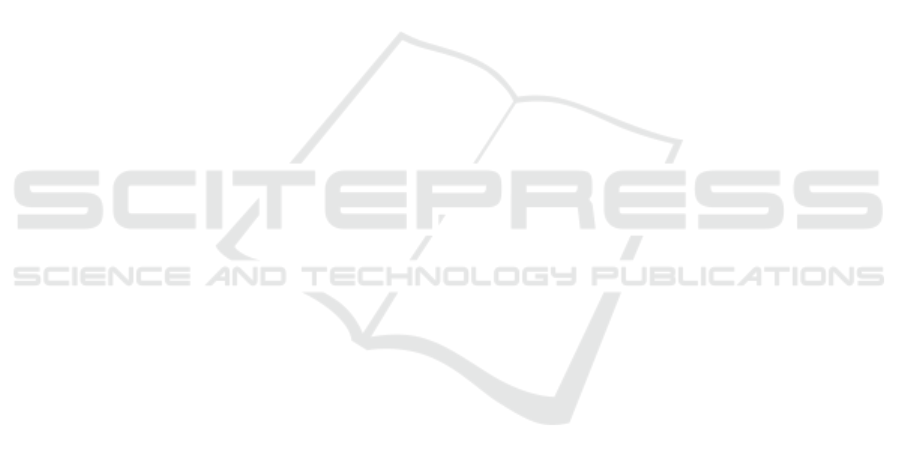
machine learning can outperform cox regression pre-
dictions and provide insights in breast cancer survival.
Scientific Reports, 11(1):6968.
Moore, C. (1990). Unpredictability and undecidability
in dynamical systems. Physical Review Letters,
64(20):2354.
Morris, M. D. (1991). Factorial sampling plans for pre-
liminary computational experiments. Technometrics,
33(2):161–174.
Nieuwenstein, M. R., Wierenga, T., Morey, R. D., Wicherts,
J. M., Blom, T. N., Wagenmakers, E.-J., and van Rijn,
H. (2015). On making the right choice: A meta-
analysis and large-scale replication attempt of the un-
conscious thought advantage. Judgment and Decision
Making, 10(1):1–17.
Nikolaev, A. R., Ehinger, B. V., Meghanathan, R. N., and
van Leeuwen, C. (2023). Planning to revisit: Neu-
ral activity in refixation precursors. Journal of Vision,
23(7):2–2.
Nikolaev, A. R., Jurica, P., Nakatani, C., Plomp, G., and
Van Leeuwen, C. (2013). Visual encoding and fixa-
tion target selection in free viewing: presaccadic brain
potentials. Frontiers in systems neuroscience, 7:26.
Planck, M. (1949). Scientific autobiography and other pa-
pers, trans. F. Gaynor (New York, 1949), pages 33–34.
Rabinovich, M., Huerta, R., and Laurent, G. (2008).
Transient dynamics for neural processing. Science,
321(5885):48–50.
Rao, R. P. and Ballard, D. H. (1999). Predictive coding in
the visual cortex: a functional interpretation of some
extra-classical receptive-field effects. Nature neuro-
science, 2(1):79–87.
Rao, S., Mehta, S., Kulkarni, S., Dalvi, H., Katre, N.,
and Narvekar, M. (2022). A study of lime and shap
model explainers for autonomous disease predictions.
In 2022 IEEE Bombay Section Signature Conference
(IBSSC), pages 1–6. IEEE.
Ribeiro, M. T., Singh, S., and Guestrin, C. (2016). ” why
should i trust you?” explaining the predictions of any
classifier. In Proceedings of the 22nd ACM SIGKDD
International Conference on Knowledge Discovery
and Data Mining, pages 1135–1144.
Schneider, W. and Shiffrin, R. M. (1977). Controlled and
automatic human information processing: I. detection,
search, and attention. Psychological review, 84(1):1.
Schwitzgebel, E. (2008). The unreliability of naive intro-
spection. Philosophical Review, 117(2):245–273.
Selvaraju, R. R., Cogswell, M., Das, A., Vedantam, R.,
Parikh, D., and Batra, D. (2017). Grad-cam: Visual
explanations from deep networks via gradient-based
localization. In Proceedings of the IEEE International
Conference on Computer Vision, pages 618–626.
Simon, H. (1978). Rationality as process and as product of
thought. American Economic Review, 68(2):1–16.
Simon, H. A. (1956). Rational choice and the structure of
the environment. Psychological Review, 63(2):129.
Simon, H. A. (1982). Models of bounded rationality, vols.
1 and 2. Economic Analysis and Public Policy, MIT
Press, Cambridge, Mass.
Simon, H. A. and Ericsson, K. A. (1984). Protocol analysis:
Verbal reports as data. (No Title).
Simonyan, K., Vedaldi, A., and Zisserman, A. (2013).
Deep inside convolutional networks: Visualising im-
age classification models and saliency maps. arXiv
preprint arXiv:1312.6034.
Skinner, D. J. and Dunkel, J. (2021). Improved bounds
on entropy production in living systems. Pro-
ceedings of the National Academy of Sciences,
118(18):e2024300118.
Sobol, I. M. (2001). Global sensitivity indices for nonlin-
ear mathematical models and their monte carlo esti-
mates. Mathematics and Computers in Simulation,
55(1-3):271–280.
Stanovich, K. E. and West, R. F. (2000). Individual differ-
ences in reasoning: Implications for the rationality de-
bate? Behavioral and Brain Sciences, 23(5):645–665.
Thomson, S. L., Adair, J., Brownlee, A. E., and van den
Berg, D. (2023). From fitness landscapes to explain-
able ai and back. In Proceedings of the Companion
Conference on Genetic and Evolutionary Computa-
tion, pages 1663–1667.
Trajanov, R., Dimeski, S., Popovski, M., Koro
ˇ
sec, P., and
Eftimov, T. (2022). Explainable landscape analysis
in automated algorithm performance prediction. In
International Conference on the Applications of Evo-
lutionary Computation (Part of EvoStar), pages 207–
222. Springer.
Tsuda, I. (2001). Toward an interpretation of dynamic neu-
ral activity in terms of chaotic dynamical systems. Be-
havioral and Brain Sciences, 24(5):793–810.
Tversky, A. and Kahneman, D. (1974). Judgment under un-
certainty: Heuristics and biases: Biases in judgments
reveal some heuristics of thinking under uncertainty.
Science, 185(4157):1124–1131.
Van Leeuwen, C. (1990). Perceptual-learning systems as
conservative structures: is economy an attractor? Psy-
chological Research, 52(2-3):145–152.
Van Stein, B., Raponi, E., Sadeghi, Z., Bouman, N.,
Van Ham, R. C., and B
¨
ack, T. (2022). A comparison
of global sensitivity analysis methods for explainable
ai with an application in genomic prediction. IEEE
Access, 10:103364–103381.
Varela, F. J., Thompson, L., and Rosch, E. (1992). The
embodied mind: Cognitive science and human expe-
rience.
Vaswani, A., Shazeer, N., Parmar, N., Uszkoreit, J., Jones,
L., Gomez, A. N., Kaiser, Ł., and Polosukhin, I.
(2017). Attention is all you need. Advances in Neural
Information Processing Systems, 30.
Verstijnen, I., Van Leeuwen, C., Hamel, R., and Hennessey,
J. (2000). What imagery can’t do and why sketching
might help. Empirical Studies of the Arts, 18(2):167–
182.
Wong, B. Y. and Jones, W. (1982). Increasing metacompre-
hension in learning disabled and normally achieving
students through self-questioning training. Learning
Disability Quarterly, 5(3):228–240.
Yang, J. (2021). Fast treeshap: Accelerating shap
The Opaque Nature of Intelligence and the Pursuit of Explainable AI
563