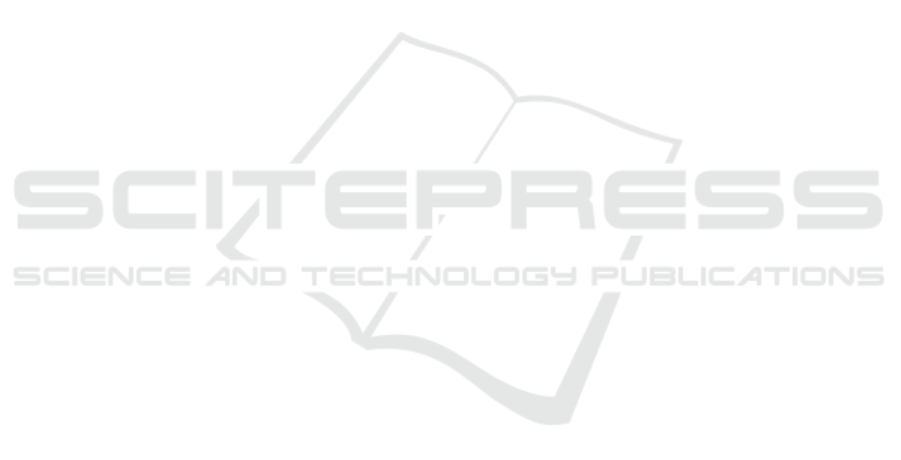
Dritsas, E. and Trigka, M. (2022b). Lung cancer risk pre-
diction with machine learning models. Big Data and
Cognitive Computing, 6(4):139.
Dritsas, E. and Trigka, M. (2022c). Machine learning meth-
ods for hypercholesterolemia long-term risk predic-
tion. Sensors, 22(14):5365.
Dritsas, E. and Trigka, M. (2022d). Machine learning tech-
niques for chronic kidney disease risk prediction. Big
Data and Cognitive Computing, 6(3):98.
Dritsas, E. and Trigka, M. (2022e). Stroke risk pre-
diction with machine learning techniques. Sensors,
22(13):4670.
Dritsas, E. and Trigka, M. (2022f). Supervised machine
learning models to identify early-stage symptoms of
sars-cov-2. Sensors, 23(1):40.
Dritsas, E. and Trigka, M. (2023a). Efficient data-driven
machine learning models for cardiovascular diseases
risk prediction. Sensors, 23(3):1161.
Dritsas, E. and Trigka, M. (2023b). Supervised machine
learning models for liver disease risk prediction. Com-
puters, 12(1):19.
Dritsas, E., Trigka, M., and Mylonas, P. (2023). Ensemble
machine learning models for breast cancer identifica-
tion. In IFIP International Conference on Artificial
Intelligence Applications and Innovations, pages 303–
311. Springer.
Fazakis, N., Dritsas, E., Kocsis, O., Fakotakis, N., and
Moustakas, K. (2021a). Long-term cholesterol risk
prediction using machine learning techniques in elsa
database. In IJCCI, pages 445–450.
Fazakis, N., Kocsis, O., Dritsas, E., Alexiou, S., Fakotakis,
N., and Moustakas, K. (2021b). Machine learning
tools for long-term type 2 diabetes risk prediction.
IEEE Access, 9:103737–103757.
Gandaglia, G., Leni, R., Bray, F., Fleshner, N., Freed-
land, S. J., Kibel, A., Stattin, P., Van Poppel, H., and
La Vecchia, C. (2021). Epidemiology and preven-
tion of prostate cancer. European urology oncology,
4(6):877–892.
Hossin, M. and Sulaiman, M. N. (2015). A review on eval-
uation metrics for data classification evaluations. In-
ternational journal of data mining & knowledge man-
agement process, 5(2):1.
Huljanah, M., Rustam, Z., Utama, S., and Siswantining, T.
(2019). Feature selection using random forest classi-
fier for predicting prostate cancer. In IOP Conference
Series: Materials Science and Engineering, volume
546, page 052031. IOP Publishing.
Konstantoulas, I., Dritsas, E., and Moustakas, K. (2022).
Sleep quality evaluation in rich information data. In
2022 13th International Conference on Information,
Intelligence, Systems & Applications (IISA), pages 1–
4. IEEE.
Konstantoulas, I., Kocsis, O., Dritsas, E., Fakotakis, N., and
Moustakas, K. (2021). Sleep quality monitoring with
human assisted corrections. In IJCCI, pages 435–444.
Laabidi, A. and Aissaoui, M. (2020). Performance analysis
of machine learning classifiers for predicting diabetes
and prostate cancer. In 2020 1st international confer-
ence on innovative research in applied science, engi-
neering and technology (IRASET), pages 1–6. IEEE.
Leitzmann, M. F. and Rohrmann, S. (2012). Risk factors
for the onset of prostatic cancer: age, location, and
behavioral correlates. Clinical epidemiology, pages
1–11.
Leung, K. M. et al. (2007). Naive bayesian classifier.
Polytechnic University Department of Computer Sci-
ence/Finance and Risk Engineering, 2007:123–156.
Liu, Y., Mu, Y., Chen, K., Li, Y., and Guo, J. (2020).
Daily activity feature selection in smart homes based
on pearson correlation coefficient. Neural Processing
Letters, 51:1771–1787.
Maalouf, M. (2011). Logistic regression in data analysis:
an overview. International Journal of Data Analysis
Techniques and Strategies, 3(3):281–299.
Matsushita, M., Fujita, K., and Nonomura, N. (2020). In-
fluence of diet and nutrition on prostate cancer. Inter-
national journal of molecular sciences, 21(4):1447.
Mottet, N., Bellmunt, J., Briers, E., Van den Bergh, R.,
Bolla, M., Van Casteren, N., Cornford, P., Culine,
S., Joniau, S., Lam, T., et al. (2015). Guidelines on
prostate cancer. European Association of Urology,
56:e137.
Mushtaq, Z., Ramzan, M. F., Ali, S., Baseer, S., Samad,
A., and Husnain, M. (2022). Voting classification-
based diabetes mellitus prediction using hypertuned
machine-learning techniques. Mobile Information
Systems, 2022:1–16.
Ngo, G., Beard, R., and Chandra, R. (2022). Evolution-
ary bagging for ensemble learning. Neurocomputing,
510:1–14.
Palimkar, P., Shaw, R. N., and Ghosh, A. (2022). Machine
learning technique to prognosis diabetes disease: Ran-
dom forest classifier approach. In Advanced Com-
puting and Intelligent Technologies: Proceedings of
ICACIT 2021, pages 219–244. Springer.
Pavlyshenko, B. (2018). Using stacking approaches for ma-
chine learning models. In 2018 IEEE Second Interna-
tional Conference on Data Stream Mining & Process-
ing (DSMP), pages 255–258. IEEE.
Perdana, N. R., Mochtar, C. A., Umbas, R., and Hamid,
A. R. A. (2017). The risk factors of prostate cancer
and its prevention: a literature review. Acta medica
indonesiana, 48(3):228–238.
Pernar, C. H., Ebot, E. M., Wilson, K. M., and Mucci,
L. A. (2018). The epidemiology of prostate cancer.
Cold Spring Harbor perspectives in medicine, page
a030361.
Rawla, P. (2019). Epidemiology of prostate cancer. World
journal of oncology, 10(2):63.
Rodriguez, J. J., Kuncheva, L. I., and Alonso, C. J. (2006).
Rotation forest: A new classifier ensemble method.
IEEE transactions on pattern analysis and machine
intelligence, 28(10):1619–1630.
Sagi, O. and Rokach, L. (2018). Ensemble learning: A sur-
vey. Wiley Interdisciplinary Reviews: Data Mining
and Knowledge Discovery, 8(4):e1249.
Machine Learning Models for Prostate Cancer Identification
427