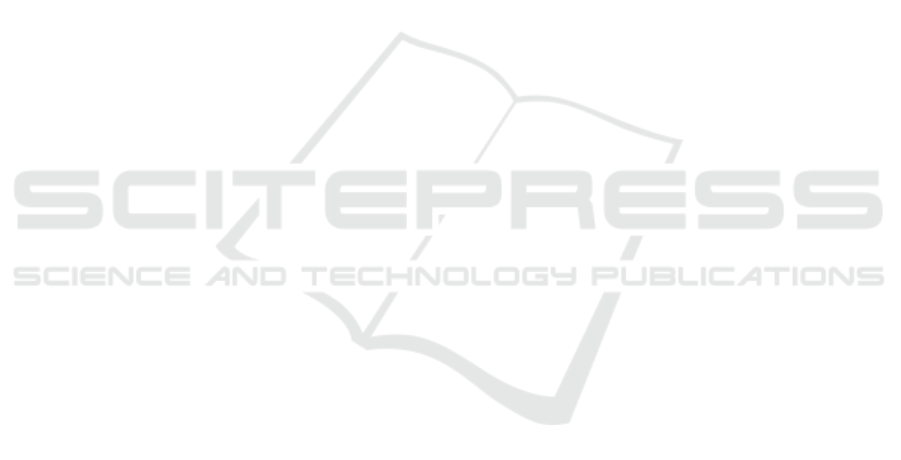
S., Chen, T., et al. (2021). Big self-supervised models
advance medical image classification. In Proceedings
of the IEEE/CVF International Conference on Com-
puter Vision, pages 3478–3488.
Baltruschat, I. M., Nickisch, H., Grass, M., Knopp, T., and
Saalbach, A. (2019). Comparison of deep learning
approaches for multi-label chest x-ray classification.
Scientific reports, 9(1):1–10.
Bustos, A., Pertusa, A., Salinas, J.-M., and de la Iglesia-
Vay
´
a, M. (2020). Padchest: A large chest x-ray image
dataset with multi-label annotated reports. Medical
image analysis, 66:101797.
Chen, T., Kornblith, S., Norouzi, M., and Hinton, G. (2020).
A simple framework for contrastive learning of visual
representations. In International conference on ma-
chine learning, pages 1597–1607. PMLR.
Cohen, J. P., Hashir, M., Brooks, R., and Bertrand, H.
(2020). On the limits of cross-domain generalization
in automated x-ray prediction. In Medical Imaging
with Deep Learning, pages 136–155. PMLR.
Diniz, J. O. B., Diniz, P. H. B., Valente, T. L. A., Silva,
A. C., de Paiva, A. C., and Gattass, M. (2018). De-
tection of mass regions in mammograms by bilateral
analysis adapted to breast density using similarity in-
dexes and convolutional neural networks. Computer
methods and programs in biomedicine, 156:191–207.
Dosovitskiy, A., Beyer, L., Kolesnikov, A., Weissenborn,
D., Zhai, X., Unterthiner, T., Dehghani, M., Minderer,
M., Heigold, G., Gelly, S., et al. (2020). An image is
worth 16x16 words: Transformers for image recogni-
tion at scale. arXiv preprint arXiv:2010.11929.
Elgendi, M., Nasir, M. U., Tang, Q., Smith, D., Grenier,
J.-P., Batte, C., Spieler, B., Leslie, W. D., Menon,
C., Fletcher, R. R., et al. (2021). The effectiveness
of image augmentation in deep learning networks for
detecting covid-19: A geometric transformation per-
spective. Frontiers in Medicine, 8:629134.
Goceri, E. (2023). Medical image data augmentation: tech-
niques, comparisons and interpretations. Artificial In-
telligence Review, pages 1–45.
Guan, Q., Huang, Y., Luo, Y., Liu, P., Xu, M., and Yang, Y.
(2021). Discriminative feature learning for thorax dis-
ease classification in chest x-ray images. IEEE Trans-
actions on Image Processing, 30:2476–2487.
Hallifax, R., Talwar, A., Wrightson, J., Edey, A., and Glee-
son, F. (2017). State-of-the-art: Radiological inves-
tigation of pleural disease. Respiratory medicine,
124:88–99.
Han, Y., Chen, C., Tewfik, A., Ding, Y., and Peng, Y.
(2021). Pneumonia detection on chest x-ray using
radiomic features and contrastive learning. In 2021
IEEE 18th International Symposium on Biomedical
Imaging (ISBI), pages 247–251. IEEE.
Ibrahim, R. F., Yhiea, N., Mohammed, A. M., and Mo-
hamed, A. M. (2022). Pleural effusion detection us-
ing machine learning and deep learning based on com-
puter vision. In Proceedings of the 8th International
Conference on Advanced Intelligent Systems and In-
formatics 2022, pages 199–210. Springer.
Jany, B. and Welte, T. (2019). Pleural effusion in
adults—etiology, diagnosis, and treatment. Deutsches
¨
Arzteblatt International, 116(21):377.
Liz, H., Huertas-Tato, J., S
´
anchez-Monta
˜
n
´
es, M., Del Ser,
J., and Camacho, D. (2023). Deep learning for under-
standing multilabel imbalanced chest x-ray datasets.
Future Generation Computer Systems.
Pooch, E. H. P., Alva, T. A. P., and Becker, C. D. L. (2020).
A deep learning approach for pulmonary lesion identi-
fication in chest radiographs. In Brazilian Conference
on Intelligent Systems, pages 197–211. Springer.
Radford, A., Kim, J. W., Hallacy, C., Ramesh, A., Goh, G.,
Agarwal, S., Sastry, G., Askell, A., Mishkin, P., Clark,
J., et al. (2021). Learning transferable visual models
from natural language supervision. In International
conference on machine learning, pages 8748–8763.
PMLR.
Rajpurkar, P., Irvin, J., Zhu, K., Yang, B., Mehta, H., Duan,
T., Ding, D., Bagul, A., Langlotz, C., Shpanskaya, K.,
et al. (2017). Chexnet: Radiologist-level pneumonia
detection on chest x-rays with deep learning. arXiv
preprint arXiv:1711.05225.
Serte, S. and Serener, A. (2021). Classification of covid-19
and pleural effusion on chest radiographs using cnn
fusion. In 2021 International Conference on INno-
vations in Intelligent SysTems and Applications (IN-
ISTA), pages 1–6. IEEE.
Wang, X., Peng, Y., Lu, L., Lu, Z., Bagheri, M., and Sum-
mers, R. M. (2017). Chestx-ray8: Hospital-scale chest
x-ray database and benchmarks on weakly-supervised
classification and localization of common thorax dis-
eases. In Proceedings of the IEEE conference on
computer vision and pattern recognition, pages 2097–
2106.
Zaidi, S. Z. Y., Akram, M. U., Jameel, A., and Al-
ghamdi, N. S. (2021). Lung segmentation-based pul-
monary disease classification using deep neural net-
works. IEEE Access, 9:125202–125214.
Zeiser, F. A., Costa, C. A. d., Ramos, G. d. O., Bohn, H.,
Santos, I., and Righi, R. d. R. (2021). Evaluation of
convolutional neural networks for covid-19 classifi-
cation on chest x-rays. In Intelligent Systems: 10th
Brazilian Conference, BRACIS 2021, Virtual Event,
November 29–December 3, 2021, Proceedings, Part
II 10, pages 121–132. Springer.
Zhang, Y., Jiang, H., Miura, Y., Manning, C. D., and Lan-
glotz, C. P. (2022). Contrastive learning of medical
visual representations from paired images and text. In
Machine Learning for Healthcare Conference, pages
2–25. PMLR.
Zuiderveld, K. (1994). Graphics gems iv. In Heckbert,
P. S., editor, Graphics Gems, chapter Contrast Lim-
ited Adaptive Histogram Equalization, pages 474–
485. Academic Press Professional, Inc., San Diego,
CA, USA.
Pleural Effusion Classification on Chest X-Ray Images with Contrastive Learning
405