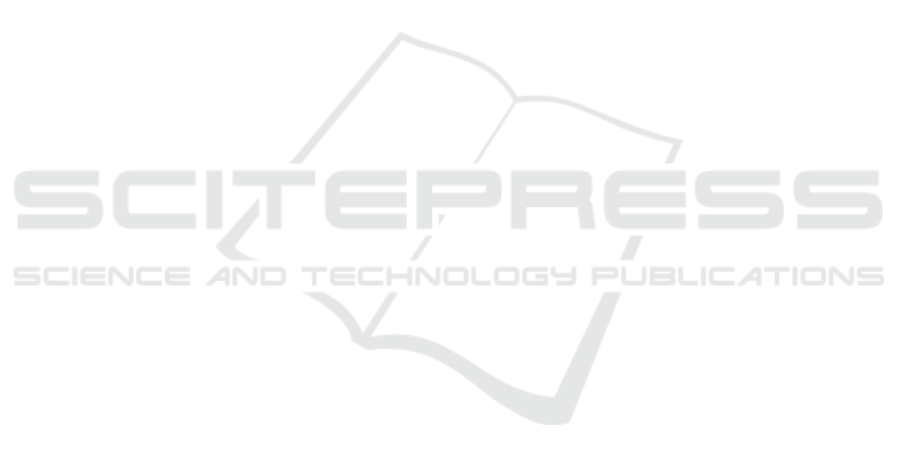
the classification of bipolar disorder episodes. Journal
of Applied Mathematics and Computer Science, 33(3).
Duarte, J. M. and Berton, L. (2023). A review of semi-
supervised learning for text classification. Artificial
Intelligence Review, pages 1–69.
Gonz
´
alez-Almagro, G., Peralta, D., De Poorter, E., Cano,
J.-R., and Garc
´
ıa, S. (2023). Semi-supervised con-
strained clustering: An in-depth overview, ranked tax-
onomy and future research directions. arXiv preprint
arXiv:2303.00522.
Hao, L. and Xu, Y. (2023). Semi-supervised learning based
occupancy estimation for real-time energy manage-
ment using ambient data. IEEE Internet of Things
Journal.
Kang, P., Kim, D., and Cho, S. (2016). Semi-supervised
support vector regression based on self-training with
label uncertainty: An application to virtual metrology
in semiconductor manufacturing. Expert Systems with
Applications, 51:85–106.
Kim, G., Choi, J. G., Ku, M., and Lim, S. (2023). De-
veloping a semi-supervised learning and ordinal clas-
sification framework for quality level prediction in
manufacturing. Computers & Industrial Engineering,
181:109286.
Kmita, K., Casalino, G., Castellano, G., Hryniewicz, O.,
and Kaczmarek-Majer, K. (2022). Confidence path
regularization for handling label uncertainty in semi-
supervised learning: use case in bipolar disorder mon-
itoring. In 2022 IEEE International Conference on
Fuzzy Systems (FUZZ-IEEE), pages 1–8. IEEE.
Kostopoulos, G., Karlos, S., Kotsiantis, S., and Ragos, O.
(2018). Semi-supervised regression: A recent review.
Journal of Intelligent & Fuzzy Systems, 35(2):1483–
1500.
Leite, D., Decker, L., Santana, M., and Souza, P. (2020).
Egfc: Evolving gaussian fuzzy classifier from never-
ending semi-supervised data streams – with applica-
tion to power quality disturbance detection and clas-
sification. In 2020 IEEE International Conference on
Fuzzy Systems (FUZZ-IEEE), pages 1–9.
Liu, Z., Kong, W., Peng, X., Yang, Z., Liu, S., Liu,
S., and Wen, C. (2023a). Dual-feature-embeddings-
based semi-supervised learning for cognitive en-
gagement classification in online course discussions.
Knowledge-Based Systems, 259:110053.
Liu, Z., Lai, Z., Ou, W., Zhang, K., and Huo, H.
(2023b). Discriminative sparse least square regression
for semi-supervised learning. Information Sciences,
636:118903.
Pedrycz, W. and Waletzky, J. (1997). Fuzzy clustering with
partial supervision. IEEE transactions on systems,
man, and cybernetics. Part B, Cybernetics, 27(5):787–
95.
Qayyum, A., Tahir, A., Butt, M. A., Luke, A., Abbas, H. T.,
Qadir, J., Arshad, K., Assaleh, K., Imran, M. A., and
Abbasi, Q. H. (2023). Dental caries detection using
a semi-supervised learning approach. Scientific Re-
ports, 13(1):749.
Qiu, L., Cheng, J., Gao, H., Xiong, W., and Ren, H. (2023).
Federated semi-supervised learning for medical image
segmentation via pseudo-label denoising. IEEE Jour-
nal of Biomedical and Health Informatics.
Wang, M., Hua, X.-S., Song, Y., Dai, L.-R., and Zhang,
H.-J. (2006). Semi-supervised kernel regression.
In Sixth International Conference on Data Mining
(ICDM’06), pages 1130–1135. IEEE.
Zhou, Z.-H., Li, M., et al. (2005). Semi-supervised regres-
sion with co-training. In IJCAI, volume 5, pages 908–
913.
Semi-Supervised Fuzzy C-Means for Regression
375