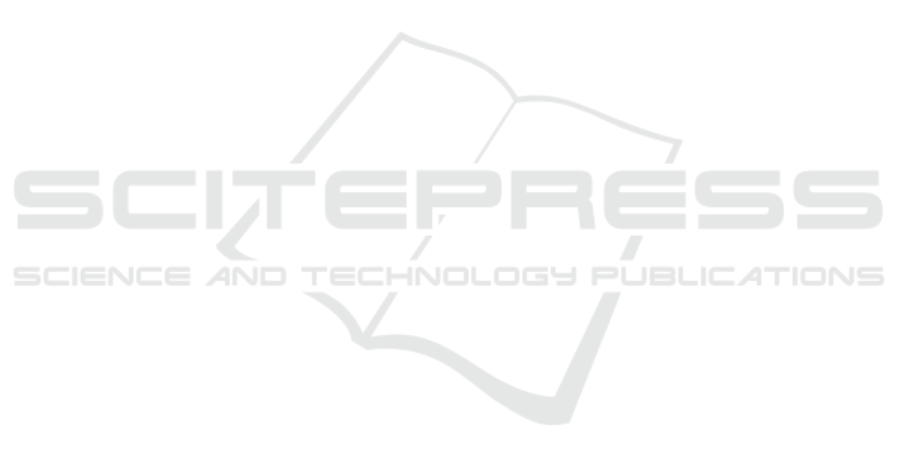
2021 Special Session and Competition on Single Ob-
jective Bound Constrained Numerical Optimization.
Technical report, Nanyang Technological University,
Singapore.
Bartz-Beielstein, T., Doerr, C., Berg, D. v. d., Bossek,
J., Chandrasekaran, S., Eftimov, T., Fischbach, A.,
Kerschke, P., La Cava, W., Lopez-Ibanez, M., et al.
(2020). Benchmarking in optimization: Best practice
and open issues. arXiv preprint arXiv:2007.03488.
Benavoli, A., Corani, G., Dem
ˇ
sar, J., and Zaffalon, M.
(2017). Time for a change: a tutorial for compar-
ing multiple classifiers through Bayesian analysis. The
Journal of Machine Learning Research, 18(1):2653–
2688.
Biedrzycki, R., Arabas, J., and Warchulski, E. (2022). A
version of nl-shade-rsp algorithm with midpoint for
cec 2022 single objective bound constrained prob-
lems. In 2022 IEEE Congress on Evolutionary Com-
putation (CEC), pages 1–8. IEEE.
Birattari, M. and Dorigo, M. (2007). How to assess and
report the performance of a stochastic algorithm on a
benchmark problem: mean or best result on a number
of runs? Optimization letters, 1:309–311.
Biswas, S., Saha, D., De, S., Cobb, A. D., Das, S., and
Jalaian, B. A. (2021). Improving Differential Evolu-
tion through Bayesian Hyperparameter Optimization.
In 2021 IEEE Congress on Evolutionary Computation
(CEC), pages 832–840. IEEE.
Brest, J., Mau
ˇ
cec, M. S., and Bo
ˇ
skovi
´
c, B. (2016). iL-
SHADE: Improved L-SHADE algorithm for single
objective real-parameter optimization. In 2016 IEEE
Congress on Evolutionary Computation (CEC), pages
1188–1195. IEEE.
Brest, J., Zamuda, A., Fister, I., and Boskovic, B. (2014).
Some improvements of the self-adaptive jde algo-
rithm. In 2014 IEEE Symposium on Differential Evo-
lution (SDE), pages 1–8. IEEE.
Bujok, P. and Kolenovsky, P. (2022). Eigen crossover in
cooperative model of evolutionary algorithms applied
to cec 2022 single objective numerical optimisation.
In 2022 IEEE Congress on Evolutionary Computation
(CEC), pages 1–8. IEEE.
Calvo, B., Shir, O. M., Ceberio, J., Doerr, C., Wang, H.,
B
¨
ack, T., and Lozano, J. A. (2019). Bayesian per-
formance analysis for black-box optimization bench-
marking. In Proceedings of the Genetic and Evolu-
tionary Computation Conference Companion, pages
1789–1797.
Carrasco, J., Garcia, S., Rueda, M., Das, S., and Herrera,
F. (2020). Recent trends in the use of statistical tests
for comparing swarm and evolutionary computing al-
gorithms: Practical guidelines and a critical review.
Swarm and Evolutionary Computation, 54:100665.
Cenikj, G., Lang, R. D., Engelbrecht, A. P., Doerr, C.,
Koro
ˇ
sec, P., and Eftimov, T. (2022). Selector: se-
lecting a representative benchmark suite for repro-
ducible statistical comparison. In Proceedings of the
Genetic and Evolutionary Computation Conference,
pages 620–629.
Hansen, N., Auger, A., Ros, R., Mersmann, O., Tu
ˇ
sar, T.,
and Brockhoff, D. (2021). COCO: A platform for
comparing continuous optimizers in a black-box set-
ting. Optimization Methods and Software, 36(1):114–
144.
Hansen, Nikolaus and Auger, Anne and Brockhoff, Dimo
and Tu
ˇ
sar, Tea (2022). Anytime performance as-
sessment in blackbox optimization benchmarking.
IEEE Transactions on Evolutionary Computation,
26(6):1293–1305.
Herzog, J., Brest, J., and Bo
ˇ
skovi
´
c, B. (2023). Analysis
based on statistical distributions: A practical approach
for stochastic solvers using discrete and continuous
problems. Information Sciences, 633:469–490.
Herzog, J., Brest, J., and Bo
ˇ
skovi
´
c, B. (2022). Performance
Analysis of Selected Evolutionary Algorithms on Dif-
ferent Benchmark Functions. In Bioinspired Opti-
mization Methods and Their Applications: 10th Inter-
national Conference, BIOMA 2022, Maribor, Slove-
nia, November 17–18, 2022, Proceedings, pages 170–
184. Springer.
Jansen, T. (2020). Analysing stochastic search heuristics
operating on a fixed budget. Theory of evolutionary
computation: recent developments in discrete opti-
mization, pages 249–270.
Kerschke, P., Hoos, H. H., Neumann, F., and Trautmann, H.
(2019). Automated algorithm selection: Survey and
perspectives. Evolutionary computation, 27(1):3–45.
Meng, Z., Zhong, Y., and Yang, C. (2021). Cs-de: Coop-
erative strategy based differential evolution with pop-
ulation diversity enhancement. Information Sciences,
577:663–696.
Nikolikj, A., Trajanov, R., Cenikj, G., Koro
ˇ
sec, P., and
Eftimov, T. (2022). Identifying minimal set of Ex-
ploratory Landscape Analysis features for reliable
algorithm performance prediction. In 2022 IEEE
Congress on Evolutionary Computation (CEC), pages
1–8. IEEE.
Piotrowski, A. P., Napiorkowski, J. J., and Piotrowska,
A. E. (2020). Population size in particle swarm op-
timization. Swarm and Evolutionary Computation,
58:100718.
Ravber, M., Moravec, M., and Mernik, M. (2022). Primer-
java evolucijskih algoritmov implementiranih v ra-
zli
ˇ
cnih programskih jezikih. Elektrotehniski Vestnik,
89(1/2):46–52. (In Slovene).
Stanovov, V., Akhmedova, S., and Semenkin, E. (2022).
NL-SHADE-LBC algorithm with linear parameter
adaptation bias change for CEC 2022 Numerical Op-
timization. In 2022 IEEE Congress on Evolutionary
Computation (CEC), pages 01–08. IEEE.
Tanabe, R. and Fukunaga, A. S. (2014). Improving the
search performance of SHADE using linear popula-
tion size reduction. In 2014 IEEE congress on evolu-
tionary computation (CEC), pages 1658–1665. IEEE.
Tu
ˇ
sar, T., Hansen, N., and Brockhoff, D. (2017). Anytime
benchmarking of budget-dependent algorithms with
the COCO platform. In IS 2017-International mul-
ticonference Information Society, pages 1–4.
Van Cuong, L., Bao, N. N., Phuong, N. K., and Binh, H.
T. T. (2022). Dynamic perturbation for population di-
versity management in differential evolution. In Pro-
ECTA 2023 - 15th International Conference on Evolutionary Computation Theory and Applications
228