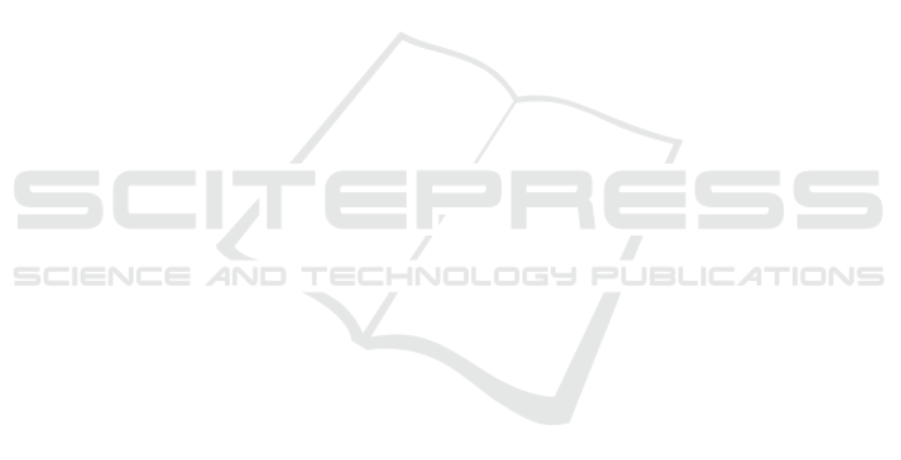
while a significant proportion of Emergency Act vis-
its come from married patients. Notably, the clus-
ter exhibits a clear preference for visits during the
winter season, particularly in November and Decem-
ber. Considering these characteristics, Cluster 3 can
be named the ”Mixed Acuity with Seasonal Prefer-
ence Cluster,” as it encompasses a mix of pediatric
and emergency visits, demonstrates distinct age and
seasonal patterns, and showcases variations in marital
status.
Regarding external factors like precipitation and
temperature, no discernible patterns were discovered
that had an impact on the utilization of the emergency
room.
In conclusion, this analysis contributes to the iden-
tification of distinct groups with unique needs, facil-
itating the development of tailored approaches to op-
timize resource allocation, improve patient care, and
enhance the overall efficiency of the emergency de-
partment. The comprehensive characterization of the
clusters, including their underlying variables and the
impact of emergency consultations, enhances the un-
derstanding of the diverse patient profiles within the
emergency department.
This information serves as a powerful tool for im-
proving patient care, enhancing resource allocation,
and addressing challenges such as overcrowding and
sub-optimal patient flow.
Future work in this context could explore alter-
native clustering algorithms or techniques to validate
and compare the results obtained using the k-means
algorithm. Such as hierarchical clustering, density-
based clustering, or model-based clustering to assess
their effectiveness in capturing the underlying pat-
terns in the data.
ACKNOWLEDGEMENTS
This work has been supported by national funds
through FCT – Fundac¸
˜
ao para a Ci
ˆ
encia e Tecnolo-
gia through project UIDB/04728/2020. The authors
thank the hospital for providing the real data used in
this study.
REFERENCES
Albarakati, N. and Obradovic, Z. (2019). Multi-domain
and multi-view networks model for clustering hospital
admissions from the emergency department. Interna-
tional Journal of Data Science and Analytics, 8:385–
403.
Charrad, M., Ghazzali, N., Boiteau, V., and Niknafs, A.
(2014). Nbclust: an r package for determining the
relevant number of clusters in a data set. Journal of
statistical software, 61:1–36.
Feretzakis, G., Sakagianni, A., Kalles, D., Loupelis, E.,
Tzelves, L., Panteris, V., Chatzikyriakou, R., Trakas,
N., Kolokytha, S., Batiani, P., Rakopoulou, Z., Tika,
A., Petropoulou, S., Dalainas, I., and Kaldis, V.
(2022). Exploratory Clustering for Emergency De-
partment Patients. IOS Press.
Guivarc’h, M., Saliba-Serre, B., Le Coz, P., and Bukiet, F.
(2020). A cross-sectional analysis of patient care path-
ways and profiles in a dental emergency department.
International Dental Journal, 70(1):21–28.
Hartigan, J. A. and Wong, M. A. (1979). Algorithm as
136: A k-means clustering algorithm. Journal of the
royal statistical society. series c (applied statistics),
28(1):100–108.
Ihaka, R. and Gentleman, R. (1996). R: a language for data
analysis and graphics. Journal of computational and
graphical statistics, 5(3):299–314.
R Core Team (2023). R: A Language and Environment for
Statistical Computing. R Foundation for Statistical
Computing, Vienna, Austria.
Valipoor, S., Hatami, M., Hakimjavadi, H., Akc¸alı, E.,
Swan, W. A., and De Portu, G. (2021). Data-driven
design strategies to address crowding and boarding in
an emergency department: A discrete-event simula-
tion study. HERD: Health Environments Research &
Design Journal, 14(2):161–177.
Wardrop, R., Ranse, J., Chaboyer, W., and Crilly, J. (2021).
Profile and outcomes of emergency department pre-
sentations based on mode of arrival: A statewide retro-
spective cohort study. Emergency Medicine Australa-
sia, 34(4):519–527.
Wartelle, A., Mourad-Chehade, F., Yalaoui, F., Questiaux,
H., Monneret, T., Soliveau, G., Chrusciel, J., Duclos,
A., Laplanche, D., and Sanchez, S. (2022). Multimor-
bidity clustering of the emergency department patient
flow: Impact analysis of new unscheduled care clinics.
Plos one, 17(1):e0262914.
Yatoo, G. H., Mufti, S., Jabeen, U., Malik, A., et al. (2021).
To study the profile of the patients attending the emer-
gency medicine department of a tertiary care teaching
hospital of north india. Biomedical Journal of Scien-
tific & Technical Research, 34(3):26758–26762.
Yeniocak, S. and Topacoglu, H. (2018). A profile of individ-
uals accompanying patients in the emergency depart-
ment: An analysis of 5046 cases. Nigerian Journal of
Clinical Practice, 21(10):1260–1264.
KDIR 2023 - 15th International Conference on Knowledge Discovery and Information Retrieval
264