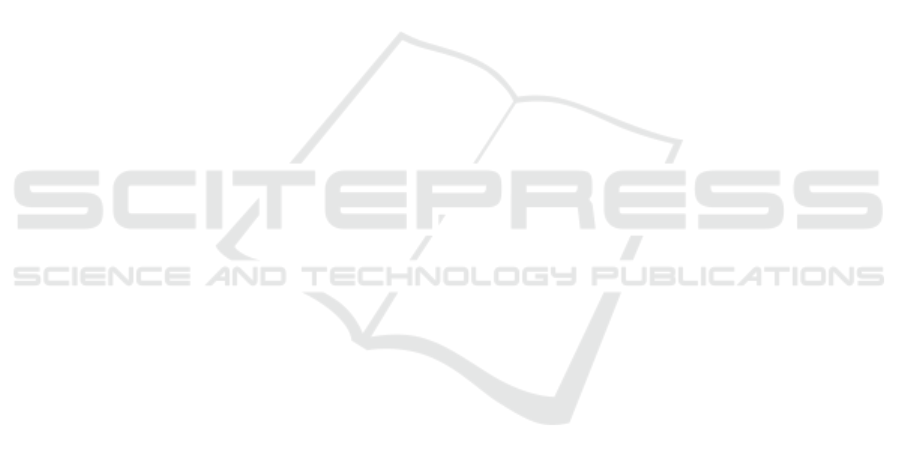
ture works will explore the use of other methods in
a hybrid way to the neo-fuzzy neuron to improve the
interpretability-accuracy trade-off.
ACKNOWLEDGEMENTS
Jorge S. S. J
´
unior is supported by Fundac¸
˜
ao para
a Ci
ˆ
encia e a Tecnologia (FCT) under the grant
ref. 2021.04917.BD. This research was supported
by the ERDF and national funds through the
project InGestAlgae (CENTRO-01-0247-FEDER-
046983), and by the FCT project UIDB/00048/2020.
REFERENCES
Angelov, P. P., Gu, X., and Pr
´
ıncipe, J. C. (2018). Au-
tonomous Learning Multimodel Systems From Data
Streams. IEEE Transactions on Fuzzy Systems,
26(4):2213–2224.
Angelov, P. P., Soares, E. A., Jiang, R., Arnold, N. I., and
Atkinson, P. M. (2021). Explainable artificial intelli-
gence: an analytical review. WIREs Data Mining and
Knowledge Discovery.
Curreri, F., Patan
`
e, L., and Xibilia, M. (2021). Soft Sen-
sor Transferability between Lines of a Sulfur Recov-
ery Unit. IFAC-PapersOnLine, 54(7):535–540.
Fortuna, L., Graziani, S., Rizzo, A., and Xibilia, M. G.
(2007). Soft Sensors for Monitoring and Control of
Industrial Processes. Springer Science & Business
Media.
Fortuna, L., Rizzo, A., Sinatra, M., and Xibilia, M. (2003).
Soft analyzers for a sulfur recovery unit. Control En-
gineering Practice, 11(12):1491–1500.
Gandin, I., Scagnetto, A., Romani, S., and Barbati, G.
(2021). Interpretability of time-series deep learning
models: A study in cardiovascular patients admitted
to Intensive care unit. Journal of Biomedical Infor-
matics, 121:103876.
Goodfellow, I., Bengio, Y., Courville, A., and Bengio, Y.
(2016). Deep learning. MIT press Cambridge.
Guo, R., Liu, H., Wang, W., Xie, G., and Zhang, Y.
(2021). A Hybrid-driven Soft Sensor with Com-
plex Process Data Based on DAE and Mechanism-
introduced GRU. In 2021 IEEE 10th Data Driven
Control and Learning Systems Conference (DDCLS),
pages 553–558.
Hochreiter, S. and Schmidhuber, J. (1997). Long Short-
Term Memory. Neural Computation, 9(8):1735–1780.
Jiang, Y., Yin, S., Dong, J., and Kaynak, O. (2021). A
Review on Soft Sensors for Monitoring, Control, and
Optimization of Industrial Processes. IEEE Sensors
Journal, 21(11):12868–12881.
J
´
unior, J. S. S., Mendes, J., Ara
´
ujo, R., Paulo, J. R., and
Premebida, C. (2021). Novelty Detection for Iterative
Learning of MIMO Fuzzy Systems. In 2021 IEEE
19th International Conference on Industrial Informat-
ics (INDIN), pages 1–7.
J
´
unior, J. S. S., Mendes, J., Souza, F., and Premebida, C.
(2023). Survey on Deep Fuzzy Systems in regression
applications: a view on interpretability. International
Journal of Fuzzy Systems, pages 1–22.
Kingma, D. P. and Ba, J. (2014). Adam: A
method for stochastic optimization. arXiv preprint
arXiv:1412.6980.
Lees, T., Reece, S., Kratzert, F., Klotz, D., Gauch, M.,
Bruijn, J. D., Sahu, R. K., Greve, P., Slater, L., and
Dadson, S. J. (2022). Hydrological concept formation
inside long short-term memory (LSTM) networks.
Hydrology and Earth System Sciences, 26(12):3079–
3101.
Liu, X., Shi, Q., Liu, Z., and Yuan, J. (2021). Using LSTM
Neural Network Based on Improved PSO and Atten-
tion Mechanism for Predicting the Effluent COD in a
Wastewater Treatment Plant. IEEE Access, 9:146082–
146096.
Mendes, J., Souza, F., Ara
´
ujo, R., and Rastegar, S.
(2019a). Neo-Fuzzy Neuron Learning Using Backfit-
ting Algorithm. Neural Computing and Applications,
31(8):3609–3618.
Mendes, J., Souza, F. A. A., Maia, R., and Ara
´
ujo, R.
(2019b). Iterative Learning of Multiple Univariate
Zero-Order T-S Fuzzy Systems. In Proc. of the
The IEEE 45th Annual Conference of the Industrial
Electronics Society (IECON 2019), pages 3657–3662.
IEEE.
Moral, J. M. A., Castiello, C., Magdalena, L., and Mencar,
C. (2021). Explainable Fuzzy Systems. Springer In-
ternational Publishing.
Silva, A. M., Caminhas, W., Lemos, A., and Gomide, F.
(2014). A fast learning algorithm for evolving neo-
fuzzy neuron. Applied Soft Computing, 14:194–209.
Souza, F., Offermans, T., Barendse, R., Postma, G., and
Jansen, J. (2022). Contextual Mixture of Experts: In-
tegrating Knowledge into Predictive Modeling. IEEE
Transactions on Industrial Informatics, pages 1–12.
Vapnik, V. (1999). The nature of statistical learning theory.
Springer science & business media.
Yamakawa, T. (1992). A Neo Fuzzy Neuron and Its Appli-
cations to System Identification and Prediction of the
System Behavior. Proc. of the 2nd Int. Conf. on Fuzzy
Logic & Neural Networks, pages 477–483.
Yuan, X., Li, L., and Wang, Y. (2020). Nonlinear Dynamic
Soft Sensor Modeling With Supervised Long Short-
Term Memory Network. IEEE Transactions on In-
dustrial Informatics, 16(5):3168–3176.
Zarzycki, K. and Ławry
´
nczuk, M. (2021). LSTM and GRU
Neural Networks as Models of Dynamical Processes
Used in Predictive Control: A Comparison of Mod-
els Developed for Two Chemical Reactors. Sensors,
21(16).
ICINCO 2023 - 20th International Conference on Informatics in Control, Automation and Robotics
288