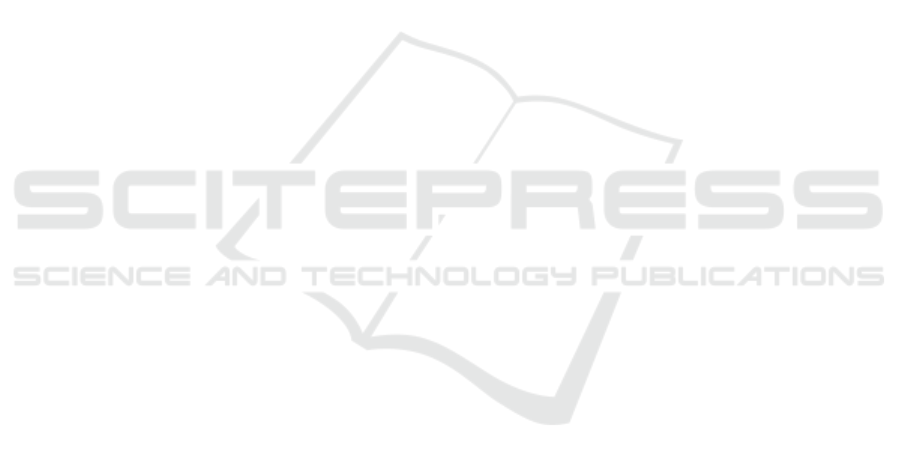
(2017). Poseidon: Face-from-depth for driver pose
estimation. In Proceedings of the IEEE Conference
on Computer Vision and Pattern Recognition (CVPR).
Hu, Z., Zhang, Y., Xing, Y., Li, Q., and Lv, C. (2022). An
integrated framework for multi-state driver monitor-
ing using heterogeneous loss and attention-based fea-
ture decoupling. Sensors, 22(19).
Jha, S. K., Marzban, M. F., Hu, T., Mahmoud, M. H., and
Busso, N. A.-D. C. (2020). The multimodal driver
monitoring database: A naturalistic corpus to study
driver attention. IEEE Transactions on Intelligent
Transportation Systems, 23:10736–10752.
Li, R., Wu, C., Li, L., Shen, Z., Xu, T., Wu, X.-J., Li, X.,
Lu, J., and Kittler, J. (2023). Action probability cal-
ibration for efficient naturalistic driving action local-
ization. In 2023 IEEE/CVF Conference on Computer
Vision and Pattern Recognition Workshops (CVPRW),
pages 5270–5277.
Liang, J., Zhu, H., Zhang, E., and Zhang, J. (2022).
Stargazer: A transformer-based driver action detec-
tion system for intelligent transportation. In Proceed-
ings of the IEEE/CVF Conference on Computer Vision
and Pattern Recognition, pages 3160–3167.
Muhrer, E. and Vollrath, M. (2011). The effect of visual and
cognitive distraction on driver’s anticipation in a simu-
lated car following scenario. Transportation Research
Part F: Traffic Psychology and Behaviour, 14(6):555–
566. Special Issue: Driving Simulation in Traffic Psy-
chology.
Naphade, M., Wang, S., Anastasiu, D. C., Tang, Z., Chang,
M., Yao, Y., Zheng, L., Rahman, M. S., Venkat-
achalapathy, A., Sharma, A., Feng, Q., Ablavsky, V.,
Sclaroff, S., Chakraborty, P., Li, A., Li, S., and Chel-
lappa, R. (2022). The 6th ai city challenge. In 2022
IEEE/CVF Conference on Computer Vision and Pat-
tern Recognition Workshops (CVPRW), pages 3346–
3355. IEEE Computer Society.
Naphade, M., Wang, S., Anastasiu, D. C., Tang, Z., Chang,
M.-C., Yao, Y., Zheng, L., Rahman, M. S., Arya,
M. S., Sharma, A., Feng, Q., Ablavsky, V., Sclaroff,
S., Chakraborty, P., Prajapati, S., Li, A., Li, S., Ku-
nadharaju, K., Jiang, S., and Chellappa, R. (2023).
The 7th ai city challenge. In The IEEE Conference
on Computer Vision and Pattern Recognition (CVPR)
Workshops.
Ortega, J. D., Kose, N., Ca
˜
nas, P., Chao, M.-A., Unnervik,
A., Nieto, M., Otaegui, O., and Salgado, L. (2020).
Dmd: A large-scale multi-modal driver monitoring
dataset for attention and alertness analysis. In Bar-
toli, A. and Fusiello, A., editors, Computer Vision –
ECCV 2020 Workshops, pages 387–405. Springer In-
ternational Publishing.
Rong, Y., Kassautzki, N.-R., Fuhl, W., and Kasneci, E.
(2022). Where and what: Driver attention-based ob-
ject detection. Proc. ACM Hum.-Comput. Interact.,
6(ETRA).
Vats, A. and Anastasiu, D. C. (2022). Key point-based
driver activity recognition. In 2022 IEEE/CVF Con-
ference on Computer Vision and Pattern Recognition
Workshops (CVPRW), pages 3273–3280.
Wang, C.-Y., Bochkovskiy, A., and Liao, H.-Y. M. (2022).
Yolov7: Trainable bag-of-freebies sets new state-of-
the-art for real-time object detectors.
Wang, Y., Zhao, T., Ding, X., Bian, J., and Fu, X. (2017).
Head pose-free eye gaze prediction for driver attention
study. In 2017 IEEE International Conference on Big
Data and Smart Computing (BigComp), pages 42–46.
Zhou, W., Qian, Y., Jie, Z., and Ma, L. (2023). Multi view
action recognition for distracted driver behavior local-
ization. In 2023 IEEE/CVF Conference on Computer
Vision and Pattern Recognition Workshops (CVPRW),
pages 5375–5380.
Driver Attention Estimation Based on Temporal Sequence Classification of Distracting Contexts
585