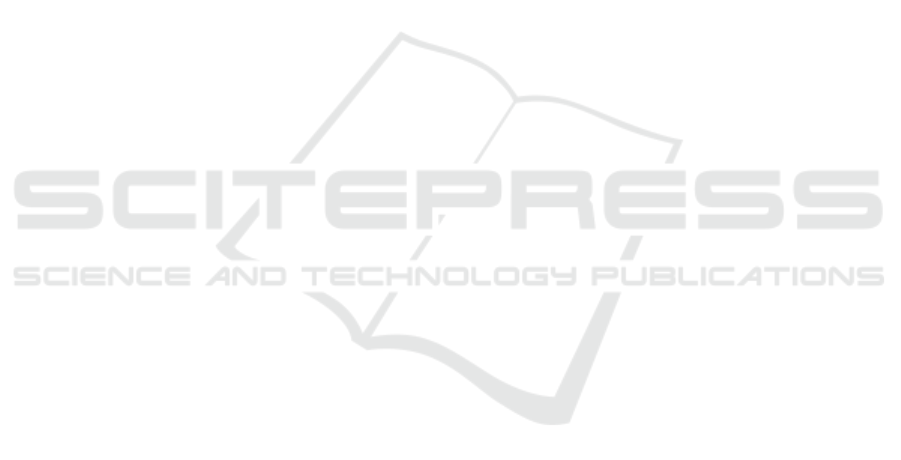
corporating the proposed steps has to be implemented
for each application separately. However, the pro-
posed techniques enable a further processing of data
in different use cases. Model training and other ap-
plications can be implemented after creating homo-
geneous data sets, which would not be possible or
well-performing in the case of raw and heterogeneous
machine data.
For future articles, a formalisation of the proposed
process is a necessary step. Additionally, by general-
ising the steps and implementing them in different ap-
plication scenarios, a comprehensive evaluation will
be an important next step.
REFERENCES
Bampoula, X., Siaterlis, G., Nikolakis, N., and Alexopou-
los, K. (2021). A Deep Learning Model for Predic-
tive Maintenance in Cyber-Physical Production Sys-
tems Using LSTM Autoencoders. Sensors, 21:972.
Bekar, E. T., Nyqvist, P., and Skoogh, A. (2020). An intelli-
gent approach for data pre-processing and analysis in
predictive maintenance with an industrial case study.
Advances in Mechanical Engineering, 12.
Bruneo, D. and De Vita, F. (2019). On the Use of LSTM
Networks for Predictive Maintenance in Smart In-
dustries. In 2019 IEEE International Conference on
Smart Computing (SMARTCOMP), pages 241–248.
Casagrande, V., Fenu, G., Pellegrino, F. A., Pin, G., Salvato,
E., and Zorzenon, D. (2021). Machine learning for
computationally efficient electrical loads estimation in
consumer washing machines. Neural Computing and
Applications, 33:15159–15170.
Cernuda, C. (2019). On the Relevance of Preprocessing
in Predictive Maintenance for Dynamic Systems. In
Lughofer, E. and Sayed-Mouchaweh, M., editors, Pre-
dictive Maintenance in Dynamic Systems: Advanced
Methods, Decision Support Tools and Real-World Ap-
plications, pages 53–93. Springer International Pub-
lishing, Cham.
Chen, L., Wei, L., Wang, Y., Wang, J., and Li, W. (2022).
Monitoring and Predictive Maintenance of Centrifu-
gal Pumps Based on Smart Sensors. Sensors, 22:2106.
Cofre-Martel, S., Lopez Droguett, E., and Modarres, M.
(2021). Big Machinery Data Preprocessing Method-
ology for Data-Driven Models in Prognostics and
Health Management. Sensors, 21:6841.
da Silva Arantes, J., da Silva Arantes, M., Fr
¨
ohlich, H. B.,
Siret, L., and Bonnard, R. (2021). A novel unsu-
pervised method for anomaly detection in time series
based on statistical features for industrial predictive
maintenance. International Journal of Data Science
and Analytics, 12:383–404.
Ding, H., Yang, L., and Yang, Z. (2019). A Predictive Main-
tenance Method for Shearer Key Parts Based on Qual-
itative and Quantitative Analysis of Monitoring Data.
IEEE Access, 7:108684–108702.
Kim, D., Lee, S., and Kim, D. (2021). An Applicable Pre-
dictive Maintenance Framework for the Absence of
Run-to-Failure Data. Applied Sciences, 11:5180.
Kulkarni, K., Devi, U., Sirighee, A., Hazra, J., and Rao,
P. (2018). Predictive Maintenance for Supermarket
Refrigeration Systems Using Only Case Temperature
Data. In 2018 Annual American Control Conference
(ACC), pages 4640–4645.
Luengo, J., Garc
´
ıa-Gil, D., Ram
´
ırez-Gallego, S., Garc
´
ıa, S.,
and Herrera, F. (2020). Big Data Preprocessing: En-
abling Smart Data. Springer International Publishing,
Cham.
Mobley, R. (2002). An Introduction to Predictive Mainte-
nance. Plant Engineering. Elsevier Science.
M
¨
uller, M. (2007). Dynamic Time Warping. In Informa-
tion Retrieval for Music and Motion, pages 69–84.
Springer, Berlin, Heidelberg.
Sagiroglu, S. and Sinanc, D. (2013). Big data: A review.
In 2013 International Conference on Collaboration
Technologies and Systems (CTS), pages 42–47.
Serradilla, O., Zugasti, E., Rodriguez, J., and Zurutuza,
U. (2022). Deep learning models for predictive
maintenance: a survey, comparison, challenges and
prospects. Applied Intelligence.
Sugumaran, V. and Ramachandran, K. I. (2011). Fault diag-
nosis of roller bearing using fuzzy classifier and his-
togram features with focus on automatic rule learning.
Expert Systems with Applications, 38:4901–4907.
Sun, C., Ma, M., Zhao, Z., Tian, S., Yan, R., and Chen,
X. (2019). Deep Transfer Learning Based on Sparse
Autoencoder for Remaining Useful Life Prediction of
Tool in Manufacturing. IEEE Transactions on Indus-
trial Informatics, 15:2416–2425. Conference Name:
IEEE Transactions on Industrial Informatics.
Wang, J., Liang, Y., Zheng, Y., Gao, R. X., and Zhang,
F. (2020). An integrated fault diagnosis and progno-
sis approach for predictive maintenance of wind tur-
bine bearing with limited samples. Renewable Energy,
145:642–650.
Yun, H., Kim, H., Jeong, Y. H., and Jun, M. B. G. (2021).
Autoencoder-based anomaly detection of industrial
robot arm using stethoscope based internal sound sen-
sor. Journal of Intelligent Manufacturing.
Z
¨
ufle, M., Moog, F., Lesch, V., Krupitzer, C., and Kounev,
S. (2022). A machine learning-based workflow for au-
tomatic detection of anomalies in machine tools. ISA
Transactions, 125:445–458.
DATA 2023 - 12th International Conference on Data Science, Technology and Applications
612