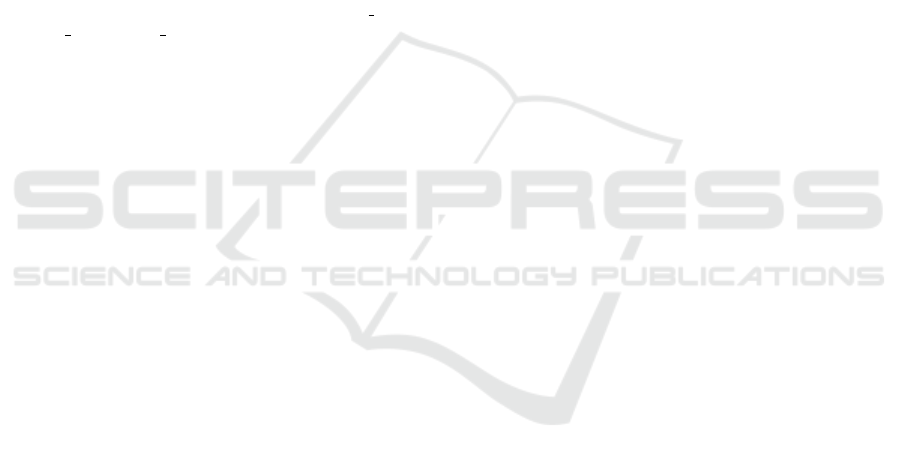
dataset. Availabe at : https://www.unb.ca/cic/dataset
s/dns-2021.html.
Google (2012). Corpus-n gram viewer. Available at: http:
//storage.googleapis.com/books/ngrams/books/datase
tsv2.html.
Grill, M., Nikolaev, I., Valeros, V., and Rehak, M. (2015).
Detecting dga malware using netflow. In 2015
IFIP/IEEE International Symposium on Integrated
Network Management (IM), pages 1304–1309. IEEE.
Hwang, C., Kim, H., Lee, H., and Lee, T. (2020). Effective
dga-domain detection and classification with textcnn
and additional features. Electronics, 9(7):1070.
Internet, A. (2018). Alexa dataset. Available at https://ww
w.alexa.com/topsites.
Kaggle (2021). Kaggle:domain generation algorithm
dataset. Available at:https://www.kaggle.com/dat
asets/gtkcyber/dga-dataset.
Labs, K. (2017). Shadowpad: Popular server management
software hit in supply chain attack. Available at https:
//media.kasperskycontenthub.com/wp-content/uploa
ds/sites/43/2017/08/07172148/ShadowPad\ technic
al\ description\ PDF.pdf.
Liu, Z., Zhang, Y., Chen, Y., Fan, X., and Dong, C. (2020).
Detection of algorithmically generated domain names
using the recurrent convolutional neural network with
spatial pyramid pooling. Entropy, 22(9):1058.
Netlab360 (Accessed: May 19, 2023). Network security
research lab at 360. https://data.netlab.360.com/dga/.
Norvig, P. (2009). Natural Language Corpus
Data:Beautiful Data, pages 219–242. O’Reilly
Media.
Oppenheim, M., Zuk, K., Meir, M., and Kessem, L. (2017).
Qakbot banking trojan causes massive active directory
lockouts. Available at https://securityintelligence.com
/qakbot-banking-trojan-causes-massive-active-direc
tory-lockouts/.
Pereira, M., Coleman, S., Yu, B., DeCock, M., and Nasci-
mento, A. (2018). Dictionary extraction and detection
of algorithmically generated domain names in passive
dns traffic. In International Symposium on Research
in Attacks, Intrusions, and Defenses, pages 295–314.
Springer.
Ren, F., Jiang, Z., Wang, X., and Liu, J. (2020). A dga
domain names detection modeling method based on
integrating an attention mechanism and deep neural
network. Cybersecurity, 3(1):1–13.
Shahzad, H., Sattar, A. R., and Skandaraniyam, J. (2021).
Dga domain detection using deep learning. In 2021
IEEE 5th International Conference on Cryptography,
Security and Privacy (CSP), pages 139–143. IEEE.
Skansi, S. (2018). Autoencoders. In Introduction to Deep
Learning, pages 153–163. Springer.
Technologies, C. P. S. (2020). July‘s most wanted malware:
Emotet strikes again after five-month absence. Avail-
able at https://blog.checkpoint.com/2020/08/07/julys
-most-wanted-malware-emotet-strikes-again-after-f
ive-month-absence/.
Tian, S., Fang, C., Liu, J., and Lei, Z. (2016). Detecting ma-
licious domains by massive dns traffic data analysis.
In 2016 8th International Conference on Intelligent
Human-Machine Systems and Cybernetics (IHMSC),
volume 1, pages 130–133. IEEE.
Vinayakumar, R., Alazab, M., Srinivasan, S., Pham, Q.-
V., Padannayil, S. B., and Simran, K. (2020). A
visualized botnet detection system based deep learn-
ing for the internet of things networks of smart
cities. IEEE Transactions on Industry Applications,
56:4436–4456.
Vormayr, G., Zseby, T., and Fabini, J. (2017). Botnet com-
munication patterns. IEEE Communications Surveys
Tutorials, 19(4):2768–2796.
Wang, T., Lin, H., Cheng, W., and Chen, C. (2017). Dbod:
Clustering and detecting dga-based botnets using dns
traffic analysis. Computers and Security, 64:1–15.
Wang, Z., Jia, Z., and Zhang, B. (2018). A detection scheme
for dga domain names based on svm. In Proceedings
of the 2018 international conference on Mathematics,
Modelling, Simulation and Algorithms (MMSA 2018).
Atlantis Press.
Xu, C., Shen, J., and Du, X. (2019). Detection method of
domain names generated by dgas based on semantic
representation and deep neural network. Computers&
Security, 85:77–88. https://www.sciencedirect.com/
science/article/pii/S0167404818312938.
Yan, F., Liu, J., Gu, L., and Chen, Z. (2020). A semi-
supervised learning scheme to detect unknown dga
domain names based on graph analysis. In 2020 IEEE
19th International Conference on Trust, Security and
Privacy in Computing and Communications (Trust-
Com), pages 1578–1583. IEEE.
Yan, X., Xiong, X., Cheng, X., Huang, Y., Zhu, H., and
Hu, F. (2021). Hmm-bimm: Hidden markov model-
based word segmentation via improved bi-directional
maximal matching algorithm. Computers & Electrical
Engineering, 94:107354.
Zhao, H., Chang, Z., Bao, G., and Zeng, X. (2019). Mali-
cious domain names detection algorithm based on n-
gram. Journal of Computer Networks and Communi-
cations, 2019.
CNN-HMM Model for Real Time DGA Categorization
829