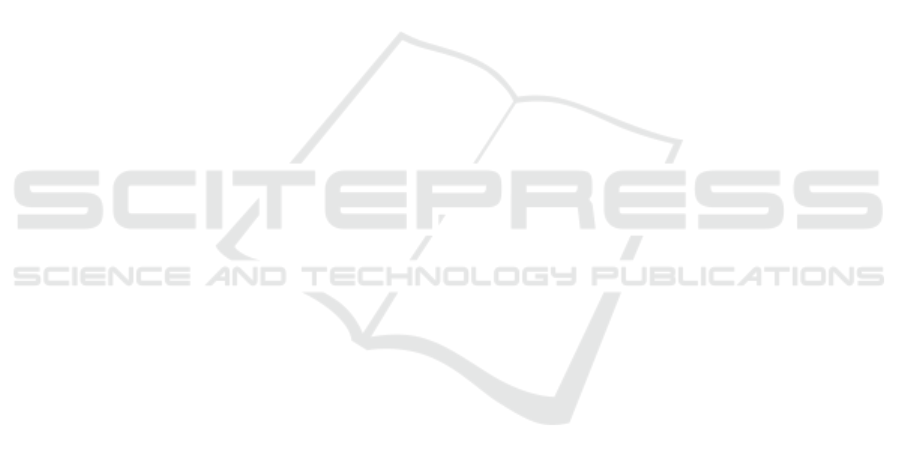
we studied statistics on the evolution of semantics
and geographical similarities and we then were able
to recommend semantic similarity threshold and geo-
graphical weighting values in order to improve both
geographical and semantic similarities. Moreover,
we conducted qualitative validation with a Ground
Truth Database which confirms the effectiveness of
our proposal with satisfactory performances. In fu-
ture work, we plan to address the problem related to
events that have too low a semantic similarity with
POI in their geographical neighborhood. We intend
to define the concept of user visits by grouping suc-
cessive and close events from the same user, which
will allow deducing the next most likely POI to at-
tach to an event. Another direction is to consider the
trajectories of a user composed of a sequence of suc-
cessively visited POI. A criterion for the consistency
of a trajectory could be specified. As a perspective,
we also plan to study event-POI matching in real-time
mobility data streams.
REFERENCES
Behera, R. K., Gunasekaran, A., Gupta, S., Kamboj, S.,
and Bala, P. K. (2020). Personalized digital market-
ing recommender engine. Journal of Retailing and
Consumer Services, 53:101799.
Beladev, M., Rokach, L., and Shapira, B. (2016). Recom-
mender systems for product bundling. Knowledge-
Based Systems, 111:193–206.
Cer, D., Yang, Y., Kong, S., Hua, N., Limtiaco, N., John,
R. S., Constant, N., Guajardo-Cespedes, M., Yuan, S.,
Tar, C., Strope, B., and Kurzweil, R. (2018). Univer-
sal sentence encoder for english. In Proceedings of
the 2018 Conference on Empirical Methods in Natu-
ral Language Processing, pages 169–174.
Chen, L., Cao, J., Tao, H., and Wu, J. (2023). Trip rein-
forcement recommendation with graph-based repre-
sentation learning. 17(4).
Deng, Y., Jiping, Liu, Y., and Luo, A. (2019). Detecting
urban polycentric structure from poi data. ISPRS In-
ternational Journal of Geo-Information, 8(6).
Esmaeili, L., Mardani, S., Golpayegani, S. A. H., and
Madar, Z. Z. (2020). A novel tourism recommender
system in the context of social commerce. Expert Sys-
tems with Applications, 149:113301.
Farazi, S. and Rafiei, D. (2022). Tracking where events take
place: Reverse spatial term queries on streaming data.
CoRR, abs/2201.07378.
Fayyaz, Z., Ebrahimian, M., Nawara, D., Ibrahim, A., and
Kashef, R. (2020). Recommendation systems: Algo-
rithms, challenges, metrics, and business opportuni-
ties. Applied Sciences, 10(21).
Geonames. The geonames dataset. "http://www.geonames.
org/export/". Online; Accessed: June 20, 2022.
Gottapu, R. D. and Sriram Monangi, L. V. (2017). Point-of-
interest recommender system for social groups. Pro-
cedia Computer Science, 114:159–164.
Gueye, I., Naacke, H., and Gançarski, S. (2020). Enrich-
ing geolocalized dataset with pois descriptions at large
scale. In Innovations and Interdisciplinary Solutions
for Underserved Areas (4th , InterSol), pages 264–
273.
Hassannia, R., Vatankhah Barenji, A., Li, Z., and Alipour,
H. (2019). Web-based recommendation system for
smart tourism: Multiagent technology. Sustainability,
11(2).
Hernández-Nieves, E., Hernández, G., Gil-González, A.-B.,
Rodríguez-González, S., and Corchado, J. M. (2020).
Fog computing architecture for personalized recom-
mendation of banking products. Expert Systems with
Applications, 140:112900.
Lim, K. H., Chan, J., Leckie, C., and Karunasekera, S.
(2015). Personalized tour recommendation based on
user interests and points of interest visit durations. In
International Conference on Artificial Intelligence, IJ-
CAI’15, pages 1778–1784.
Massimo, D. and Ricci, F. (2022). Building effective recom-
mender systems for tourists. AI Magazine, 43(2):209–
224.
Mishra, R. K., Urolagin, S., and Jothi, J. A. A. (2020).
Sentiment analysis for poi recommender systems. In
2020 Seventh International Conference on Informa-
tion Technology Trends (ITT), pages 174–179.
Pennington, J., Socher, R., and Manning, C. D. (2014).
Glove: Global vectors for word representation. In
Conference on Empirical Methods in Natural Lan-
guage Processing, EMNLP, pages 1532–1543.
Reimers, N. and Gurevych, I. (2019). Sentence-BERT: Sen-
tence embeddings using Siamese BERT-networks. In
Empirical Methods in Natural Language Processing
and International Joint Conference on Natural Lan-
guage Processing (EMNLP-IJCNLP), pages 3982–
3992.
Ricci, F. (2022). Recommender Systems in Tourism, pages
457–474. Springer.
Thomee, B., Shamma, D. A., Friedland, G., Elizalde, B.,
Ni, K., Poland, D., Borth, D., and Li, L.-J. (2016).
Yfcc100m: The new data in multimedia research.
Commun. ACM, 59(2):64–73.
Wang, H., Shen, H., Ouyang, W., and Cheng, X. (2018). Ex-
ploiting poi-specific geographical influence for point-
of-interest recommendation. In International Joint
Conference on Artificial Intelligence, IJCAI’18, pages
3877–3883.
Yannan, Z., Lu, Z., and Xinhuan, Z. (2020). The spatial dis-
tribution of retail outlets in urumqi: The application
of points of interest. Open Geosciences, 12(1):1541–
1556.
Zhou, C., Leng, M., Liu, Z., Cui, X., and Yu, J.
(2022). The impact of recommender systems and
pricing strategies on brand competition and consumer
search. Electronic Commerce Research and Applica-
tions, 53:101144.
Geo-Semantic Event-POI Matching of Large Mobility Datasets
503