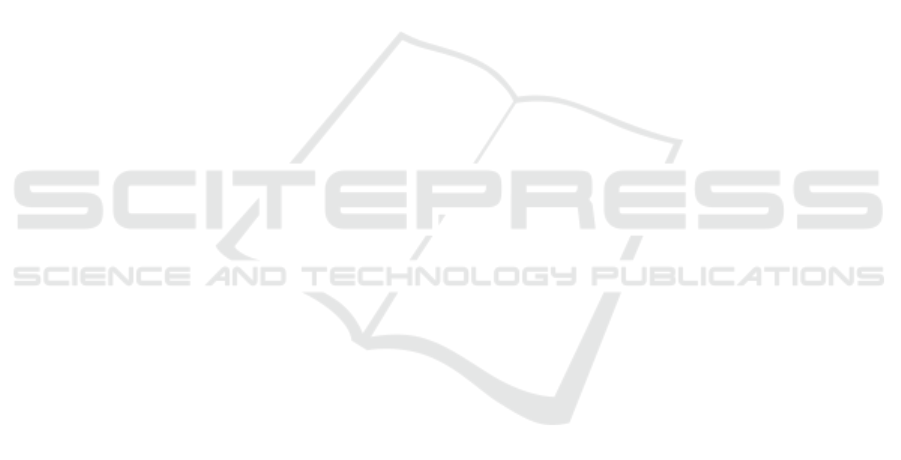
6 CONCLUSION
The proposed process-aware recommender system is
a promising method for suggesting optimal steps to-
wards process automation. By leveraging process
models discovered from sub-logs created based on ac-
tivity projections and assigned connectors in labeled
event logs, the system uses user behavior to make rec-
ommendations. The results from applying this system
to real event logs, previously labeled by an expert,
demonstrate that for most connectors, the system is
able to recommend the expected automation steps ac-
curately.
To further improve the system’s effectiveness, fu-
ture research will consider additional data attributes,
such as the resources that execute activities and time
dimensions, and explore other techniques such as as-
sociation rule mining. Finally, classical data mining
approaches will also be considered to compare the
system’s performance with alternative approaches.
Overall, these efforts will enhance the system’s rec-
ommendation capabilities and further validate its use-
fulness in process automation.
REFERENCES
Adriansyah, A. and Buijs, J. C. A. M. (2012). Mining
process performance from event logs. In Business
Process Management Workshops - BPM 2012 Inter-
national Workshops, Tallinn, Estonia, September 3,
2012. Revised Papers, pages 217–218.
Berti, A., Van Zelst, S. J., and van der Aalst, W. (2019).
Process mining for python (pm4py): bridging the gap
between process-and data science.
Chinosi, M. and Trombetta, A. (2012). BPMN: an intro-
duction to the standard. Comput. Stand. Interfaces,
34(1):124–134.
Choi, D., R’bigui, H., and Cho, C. (2022). Enabling the
gab between RPA and process mining: User interface
interactions recorder. IEEE Access, 10:39604–39612.
Eili, M. Y., Rezaeenour, J., and Sani, M. F. (2021). A
systematic literature review on process-aware recom-
mender systems. CoRR, abs/2103.16654.
Geyer-Klingeberg, J., Nakladal, J., Baldauf, F., and Veit,
F. (2018). Process mining and robotic process au-
tomation: A perfect match. In Proceedings of the
Dissertation Award, Demonstration, and Industrial
Track at BPM 2018 co-located with 16th International
Conference on Business Process Management (BPM
2018), Sydney, Australia, September 9-14, 2018, vol-
ume 2196 of CEUR Workshop Proceedings, pages
124–131. CEUR-WS.org.
Hayes, T. R. and Henderson, J. M. (2021). Looking for
semantic similarity: what a vector-space model of
semantics can tell us about attention in real-world
scenes. Psychological Science, 32(8).
Jr., S. B., Ceravolo, P., Damiani, E., and Tavares, G. M.
(2021). Using meta-learning to recommend process
discovery methods. CoRR, abs/2103.12874.
Leemans, S. J. J., Fahland, D., and van der Aalst, W. M. P.
(2013). Discovering block-structured process models
from event logs containing infrequent behaviour. In
Business Process Management Workshops - BPM Bei-
jing, China, August 26, 2013, Revised Papers, volume
171 of Lecture Notes in Business Information Process-
ing, pages 66–78. Springer.
Leno, V., Augusto, A., Dumas, M., Rosa, M. L., Maggi,
F. M., and Polyvyanyy, A. (2020). Identifying candi-
date routines for robotic process automation from un-
segmented UI logs. In 2nd International Conference
on Process Mining, ICPM 2020, Padua, Italy, October
4-9, 2020, pages 153–160. IEEE.
Mayr, A., Herm, L., Wanner, J., and Janiesch, C. (2022).
Applications and challenges of task mining: A liter-
ature review. In 30th European Conference on Infor-
mation Systems - New Horizons in Digitally United
Societies, ECIS 2022, Timisoara, Romania, June 18-
24, 2022.
Petri, C. A. and Reisig, W. (2008). Petri net. Scholarpedia,
3(4):6477.
Qiu, X., Sun, T., Xu, Y., Shao, Y., Dai, N., and Huang,
X. (2020). Pre-trained models for natural language
processing: A survey. CoRR, abs/2003.08271.
Ribeiro, J., Carmona, J., Misir, M., and Sebag, M. (2014). A
recommender system for process discovery. In Busi-
ness Process Management - 12th International Con-
ference, BPM 2014, Haifa, Israel, September 7-11,
2014. Proceedings, volume 8659 of Lecture Notes in
Computer Science, pages 67–83. Springer.
Rozinat, A. and van der Aalst, W. M. P. (2008). Confor-
mance checking of processes based on monitoring real
behavior. Inf. Syst., 33(1):64–95.
Schonenberg, H., Weber, B., Dongen, B. v., and Aalst, W.
v. d. (2008). Supporting flexible processes through
recommendations based on history. In International
Conference on Business Process Management, pages
51–66. Springer.
Seeliger, A., Nolle, T., and Mühlhäuser, M. (2018). Pro-
cess explorer: an interactive visual recommendation
system for process mining. In KDD Workshop on In-
teractive Data Exploration and Analytics.
Sroka, M. and Fani Sani, M. (2022). 50 business assign-
ments log.
Syed, R., Suriadi, S., Adams, M., Bandara, W., Leemans,
S. J., Ouyang, C., ter Hofstede, A. H., van de Weerd,
I., Wynn, M. T., and Reijers, H. A. (2020). Robotic
process automation: contemporary themes and chal-
lenges. Computers in Industry, 115:103162.
Terragni, A. and Hassani, M. (2018). Analyzing customer
journey with process mining: From discovery to rec-
ommendations. In 2018 IEEE 6th International Con-
ference on Future Internet of Things and Cloud (Fi-
Cloud), pages 224–229. IEEE.
van der Aalst, W. M. P. (2016). Process Mining - Data
Science in Action, Second Edition. Springer Berlin
Heidelberg.
Behavioral Recommender System for Process Automation Steps
261