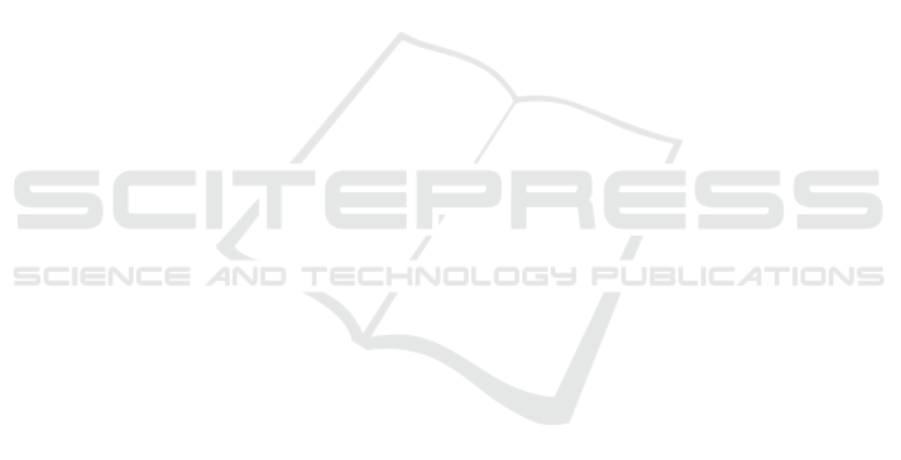
IEEE International Conference on Software Mainte-
nance and Evolution (ICSME), pages 439–443. IEEE.
Cascavilla, G., Catolino, G., and Sangiovanni, M. (2022b).
Illicit darkweb classification via natural-language pro-
cessing: Classifying illicit content of webpages based
on textual information. In Proceedings of the 19th In-
ternational Conference on Security and Cryptography
- Volume 1: SECRYPT,, pages 620–626. INSTICC,
SciTePress.
Chopra, S., Hadsell, R., and LeCun, Y. (2005). Learning
a similarity metric discriminatively, with application
to face verification. In 2005 IEEE Computer Society
Conference on Computer Vision and Pattern Recogni-
tion, volume 1, pages 539–546.
Choshen, L., Eldad, D., Hershcovich, D., Sulem, E., and
Abend, O. (2019). The language of legal and illegal
activity on the Darknet. In Proceedings of the 57th
Annual Meeting of the Association for Computational
Linguistics, pages 4271–4279.
Dalins, J., Tyshetskiy, Y., Wilson, C., Carman, M. J., and
Boudry, D. (2018). Laying foundations for effective
machine learning in law enforcement. majura – a la-
belling schema for child exploitation materials. Digi-
tal Investigation, 26:40 – 54.
Fei-Fei, L., Fergus, R., and Perona, P. (2006). One-shot
learning of object categories. IEEE transactions on
pattern analysis and machine intelligence, 28:594–
611.
Fidalgo, E., Alegre, E., Fern
´
andez-Robles, L., and
Gonz
´
alez-Castro, V. (2019). Classifying suspicious
content in tor darknet through semantic attention key-
point filtering. Digital Investigation, 30:12 – 22.
Fidalgo, E., Alegre, E., Gonz
´
alez-Castro, V., and
Fern
´
andez-Robles, L. (2018). Illegal activity categori-
sation in darknet based on image classification using
creic method. pages 600–609.
Garcia, V. and Bruna, J. (2017). Few-shot learning with
graph neural networks.
Ghosh, S., Das, A., Porras, P., Yegneswaran, V., and
Gehani, A. (2017). Automated categorization of onion
sites for analyzing the darkweb ecosystem. pages
1793–1802.
Godawatte, K., Raza, M., Murtaza, M., and Saeed, A.
(2019). Dark web along with the dark web marketing
and surveillance. In PDCAT, pages 483–485. IEEE.
Gojare, S., Joshi, R., and Gaigaware, D. (2015). Analy-
sis and design of selenium webdriver automation test-
ing framework. Procedia Computer Science, 50:341 –
346. Big Data, Cloud and Computing Challenges.
Hadsell, R., Chopra, S., and LeCun, Y. (2006). Dimen-
sionality reduction by learning an invariant mapping.
In 2006 IEEE Computer Society Conference on Com-
puter Vision and Pattern Recognition (CVPR’06), vol-
ume 2, pages 1735–1742. IEEE.
Hashemi, M. and Hall, M. (2019). Detecting and classify-
ing online dark visual propaganda. Image and Vision
Computing, 89:95 – 105.
Hilliard, N., Phillips, L., Howland, S., Yankov, A., Corley,
C. D., and Hodas, N. O. (2018). Few-shot learning
with metric-agnostic conditional embeddings.
Juan Sanchez, G. G. (2019). Who’s afraid of the dark? hype
versus reality on the dark web. https://www.recorded
future.com/dark-web-reality.
Koch, G., Zemel, R., and Salakhutdinov, R. (2015).
Siamese neural networks for one-shot image recogni-
tion. In ICML deep learning workshop, volume 2.
Lake, B. M., Salakhutdinov, R., Gross, J., and Tenenbaum,
J. B. (2011). One shot learning of simple visual con-
cepts. Cognitive Science, 33.
Li, Z., Zhou, F., Chen, F., and Li, H. (2017). Meta-sgd:
Learning to learn quickly for few shot learning. CoRR,
abs/1707.09835.
Lin, X., Wang, H., Li, Z., Zhang, Y., Yuille, A., and Lee,
T. S. (2017). Transfer of view-manifold learning to
similarity perception of novel objects.
Melekhov, I., Kannala, J., and Rahtu, E. (2016). Siamese
network features for image matching. In 2016
23rd International Conference on Pattern Recognition
(ICPR), pages 378–383.
Ochal, M. et al. (2021). Class imbalance in few-shot learn-
ing.
O’Mahony, N., Campbell, S., Carvalho, A., Krpalkova, L.,
Hernandez, G. V., Harapanahalli, S., Riordan, D., and
Walsh, J. (2019). One-shot learning for custom iden-
tification tasks; a review. Procedia Manufacturing,
38:186–193.
Qiao, S., Liu, C., et al. (2017). Few-shot image recognition
by predicting parameters from activations.
Raghavan, S. and Garc
´
ıa-Molina, H. (2001). Crawling the
hidden web. In Proceedings of the 27th International
Conference on Very Large Databases (VLDB 2001),
pages 129–138.
Ranade, P., Mittal, S., Joshi, A., and Joshi, K. (2018). Using
deep neural networks to translate multi-lingual threat
intelligence.
Replication-Package (2023). When the few outweigh the
many: Illicit content recognition with few-shot learn-
ing. https://doi.org/10.5281/zenodo.7657482.
Schroff, F., Kalenichenko, D., and Philbin, J. (2015).
Facenet: A unified embedding for face recognition
and clustering. 2015 IEEE Conference on Computer
Vision and Pattern Recognition (CVPR).
Shaban, A., Bansal, S., Liu, Z., Essa, I., and Boots, B.
(2017). One-shot learning for semantic segmentation.
Varior, R. R., Haloi, M., and Wang, G. (2016). Gated
siamese convolutional neural network architecture for
human re-identification.
Vinyals, O., Blundell, C., Lillicrap, T. P., Kavukcuoglu, K.,
and Wierstra, D. (2016). Matching networks for one
shot learning. In NIPS.
Wang, Y., Yao, Q., Kwok, J., and Ni, L. M. (2019). Gen-
eralizing from a few examples: A survey on few-shot
learning.
SECRYPT 2023 - 20th International Conference on Security and Cryptography
332