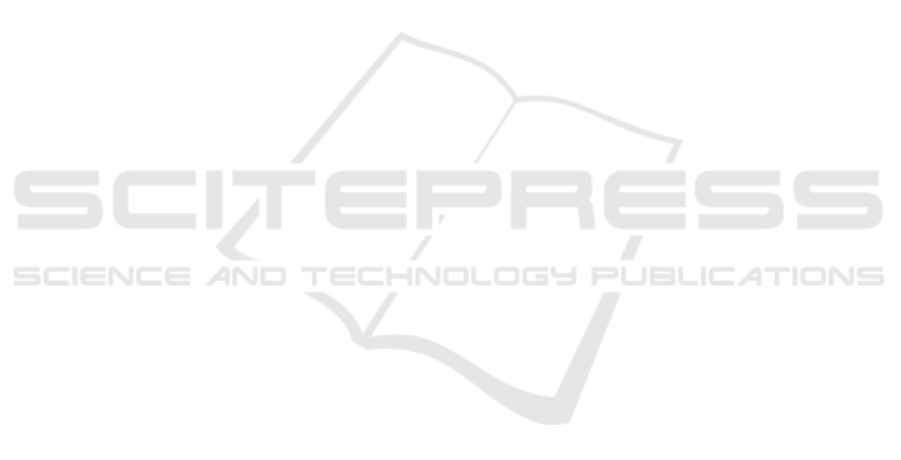
Abel, M., Gastal, E. S. L., Michelin, C. R. L., Maggi, L. G.,
Firnkes, B. E., Pachas, F. E. H., and dos Santos Al-
varenga, R. (2019). A knowledge organization system
for image classification and retrieval in petroleum ex-
ploration domain. In ONTOBRAS.
Abou Baker, N., Zengeler, N., and Handmann, U. (2022).
A transfer learning evaluation of deep neural net-
works for image classification. Machine Learning and
Knowledge Extraction, 4(1):22–41.
Anthimopoulos, M., Christodoulidis, S., Ebner, L., Christe,
A., and Mougiakakou, S. (2016). Lung pattern classi-
fication for interstitial lung diseases using a deep con-
volutional neural network. IEEE transactions on med-
ical imaging, 35(5):1207–1216.
Ara
´
ujo, T., Aresta, G., Castro, E., Rouco, J., Aguiar,
P., Eloy, C., Pol
´
onia, A., and Campilho, A. (2017).
Classification of breast cancer histology images us-
ing convolutional neural networks. PloS one,
12(6):e0177544.
Arruzzo, F. (2016). Mapeamento geol
´
ogico estrutural da
formac¸
˜
ao tiradentes, mesoproteroz
´
oico, s
˜
ao jo
˜
ao del
rei.
Barstugan, M., Ozkaya, U., and Ozturk, S. (2020).
Coronavirus (covid-19) classification using ct im-
ages by machine learning methods. arXiv preprint
arXiv:2003.09424.
Celik, Y., Talo, M., Yildirim, O., Karabatak, M., and
Acharya, U. R. (2020). Automated invasive ductal car-
cinoma detection based using deep transfer learning
with whole-slide images. Pattern Recognition Letters,
133:232–239.
Chen, C.-F. R., Fan, Q., and Panda, R. (2021). Crossvit:
Cross-attention multi-scale vision transformer for im-
age classification. In Proceedings of the IEEE/CVF
international conference on computer vision, pages
357–366.
Dosovitskiy, A., Beyer, L., Kolesnikov, A., Weissenborn,
D., Zhai, X., Unterthiner, T., Dehghani, M., Minderer,
M., Heigold, G., Gelly, S., et al. (2020). An image is
worth 16x16 words: Transformers for image recogni-
tion at scale. arXiv preprint arXiv:2010.11929.
Dung, C. V. et al. (2019). Autonomous concrete crack de-
tection using deep fully convolutional neural network.
Automation in Construction, 99:52–58.
Han, F., Yao, J., Zhu, H., and Wang, C. (2020). Underwater
image processing and object detection based on deep
cnn method. Journal of Sensors, 2020.
Hollink, L., Schreiber, G., Wielemaker, J., Wielinga, B.,
et al. (2003). Semantic annotation of image collec-
tions. In Knowledge capture, volume 2.
Hong, D., Gao, L., Yokoya, N., Yao, J., Chanussot, J., Du,
Q., and Zhang, B. (2020). More diverse means bet-
ter: Multimodal deep learning meets remote-sensing
imagery classification. IEEE Transactions on Geo-
science and Remote Sensing, 59(5):4340–4354.
Horry, M. J., Chakraborty, S., Paul, M., Ulhaq, A., Prad-
han, B., Saha, M., and Shukla, N. (2020). Covid-19
detection through transfer learning using multimodal
imaging data. Ieee Access, 8:149808–149824.
Huang, G., Liu, Z., Van Der Maaten, L., and Weinberger,
K. Q. (2017). Densely connected convolutional net-
works. In Proceedings of the IEEE conference on
computer vision and pattern recognition, pages 4700–
4708.
Ivanoff, M. (2013). Sedimentac¸
˜
ao da lagoa de itapeva, rs
- brasil. Master’s thesis, Instituto de Geoci
ˆ
encias,
UFRGS.
Kieffer, B., Babaie, M., Kalra, S., and Tizhoosh,
H. R. (2017). Convolutional neural networks for
histopathology image classification: Training vs. us-
ing pre-trained networks. In 2017 Seventh Interna-
tional Conference on Image Processing Theory, Tools
and Applications (IPTA), pages 1–6. IEEE.
Kim, H. E., Cosa-Linan, A., Santhanam, N., Jannesari, M.,
Maros, M. E., and Ganslandt, T. (2022). Transfer
learning for medical image classification: a literature
review. BMC medical imaging, 22(1):1–13.
Krause, J., Stark, M., Deng, J., and Fei-Fei, L. (2013). 3d
object representations for fine-grained categorization.
In Proceedings of the IEEE international conference
on computer vision workshops, pages 554–561.
Krizhevsky, A., Hinton, G., et al. (2009). Learning multiple
layers of features from tiny images. Master’s thesis,
University of Tront.
Krizhevsky, A., Sutskever, I., and Hinton, G. E. (2012). Im-
agenet classification with deep convolutional neural
networks. Advances in neural information processing
systems, 25.
LeCun, Y., Bottou, L., Bengio, Y., and Haffner, P. (1998).
Gradient-based learning applied to document recogni-
tion. Proceedings of the IEEE, 86(11):2278–2324.
Li, Y., Zhao, H., Qi, X., Wang, L., Li, Z., Sun, J., and Jia,
J. (2021). Fully convolutional networks for panoptic
segmentation. In Proceedings of the IEEE/CVF con-
ference on computer vision and pattern recognition,
pages 214–223.
Liang, G. and Zheng, L. (2020). A transfer learning
method with deep residual network for pediatric pneu-
monia diagnosis. Computer methods and programs in
biomedicine, 187:104964.
Missio J
´
unior, V. (2014). Geoqu
´
ımica org
ˆ
anica das turfeiras
das praias de hermenegildo e maravilhas. Master’s
thesis, Instituto de Qu
´
ımica, UFRGS.
Mohan, A. and Venkatesan, M. (2020). Hybridcnn based
hyperspectral image classification using multiscale
spatiospectral features. Infrared Physics & Technol-
ogy, 108:103326.
Mormont, R., Geurts, P., and Mar
´
ee, R. (2018). Comparison
of deep transfer learning strategies for digital pathol-
ogy. In Proceedings of the IEEE conference on com-
puter vision and pattern recognition workshops, pages
2262–2271.
Paiva, R, G. (2018). Estratigrafia de sequ
ˆ
encias aplicada
`
a
formac¸
˜
ao poti, viseano da bacia do parna
´
ıba. Master’s
thesis, Instituto de Geoci
ˆ
encias, UnB.
Radford, A., Kim, J. W., Hallacy, C., Ramesh, A., Goh, G.,
Agarwal, S., Sastry, G., Askell, A., Mishkin, P., Clark,
J., et al. (2021). Learning transferable visual models
from natural language supervision. In International
Multiscale Context Features for Geological Image Classification
417