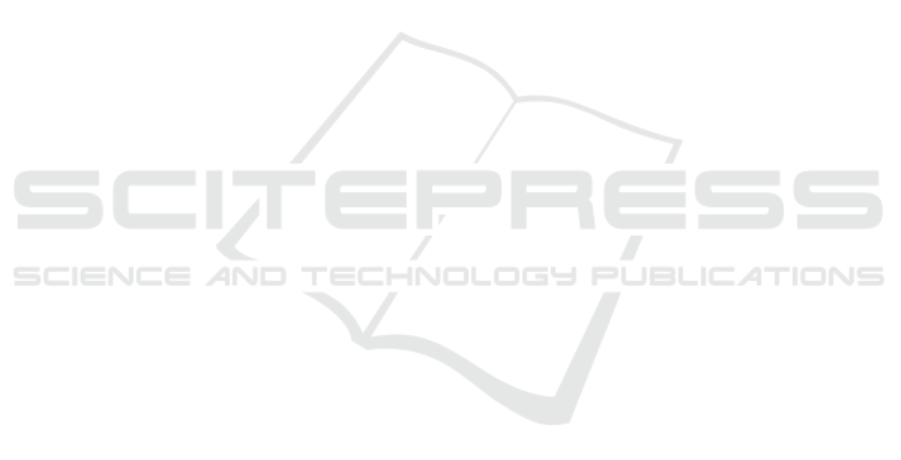
Ebner, M. and Hansen, J. (2013). Depth map color con-
stancy. Bio-Algorithms and Med-Systems, 9(4):167–
177.
Eigen, D. and Fergus, R. (2015). Predicting depth, surface
normals and semantic labels with a common multi-
scale convolutional architecture. In Int. Conf. Comput.
Vision, Santiago, Chile. IEEE.
Fan, R., Wang, H., Xue, B., Huang, H., Wang, Y., Liu, M.,
and Pitas, I. (2021). Three-filters-to-normal: An ac-
curate and ultrafast surface normal estimator. IEEE
Robot. Automat. Letters, 6(3):5405–5412.
Fouhey, D. F., Gupta, A., and Hebert, M. (2013). Data-
driven 3D primitives for single image understanding.
In Int. Conf. Comput. Vision, pages 3392–3399, Syd-
ney, NSW, Australia. IEEE.
Garces, E., Rodriguez-Pardo, C., Casas, D., and Lopez-
Moreno, J. (2022). A survey on intrinsic images: Delv-
ing deep into lambert and beyond. Int. J. Comput. Vi-
sion, 130:836–868.
Gouraud, H. (1971). Continuous shading of curved surfaces.
IEEE Trans. Computers, 100(6):623–629.
Grosse, R., Johnson, M. K., Adelson, E. H., and Free-
man, W. T. (2009). Ground truth dataset and base-
line evaluations for intrinsic image algorithms. In
Int. Conf. Comput. Vision, pages 2335–2342, Kyoto,
Japan. IEEE.
Harms, H., Beck, J., Ziegler, J., and Stiller, C. (2014). Ac-
curacy analysis of surface normal reconstruction in
stereo vision. In Intell. Vehicles Symp. Proc., pages
730–736, Dearborn, MI, USA. IEEE.
Hsu, H., Su, H.-T., Yeh, J.-F., Chung, C.-M., and Hsu, W. H.
(2022). SeqDNet: Improving missing value by se-
quential depth network. In Int. Conf. Image Process.,
pages 1826–1830, Bordeaux, France. IEEE.
Ioannou, Y., Taati, B., Harrap, R., and Greenspan, M.
(2012). Difference of normals as a multi-scale opera-
tor in unorganized point clouds. In Int. Conf. 3D Imag.
Model. Process. Visualization Transmiss., pages 501–
508, Zurich, Switzerland. IEEE.
Izadi, S., Kim, D., Hilliges, O., Molyneaux, D., Newcombe,
R., Kohli, P., Shotton, J., Hodges, S., Freeman, D.,
Davison, A., and Fitzgibbon, A. (2011). KinectFu-
sion: real-time 3D reconstruction and interaction us-
ing a moving depth camera. In Proc. Annu. ACM
Symp. User Interface Softw. Technol., pages 559–568,
Santa Barbara, CA, USA. ACM.
Jeon, J., Cho, S., Tong, X., and Lee, S. (2014). Intrinsic im-
age decomposition using structure-texture separation
and surface normals. In Eur. Conf. Comput. Vision,
pages 218–233, Zurich, Switzerland. Springer.
Karakaya, D., Ulucan, O., and Turkan, M. (2022). Image de-
clipping: Saturation correction in single images. Digit.
Signal Process., 127:103537.
Klasing, K., Althoff, D., Wollherr, D., and Buss, M. (2009).
Comparison of surface normal estimation methods for
range sensing applications. In Int. Conf. Robot. Au-
tomat., pages 3206–3211, Kobe, Japan. IEEE.
Kwon, H., Tai, Y.-W., and Lin, S. (2015). Data-driven depth
map refinement via multi-scale sparse representation.
In Conf. Comput. Vision Pattern Recognit., pages 159–
167, Boston, MA, USA. IEEE.
Li, B., Shen, C., Dai, Y., Van Den Hengel, A., and He, M.
(2015). Depth and surface normal estimation from
monocular images using regression on deep features
and hierarchical CRFs. In Conf. Comput. Vision Pat-
tern Recognit., pages 1119–1127, Boston, MA, USA.
IEEE.
Li, K., Zhao, M., Wu, H., Yan, D.-M., Shen, Z., Wang,
F.-Y., and Xiong, G. (2022). GraphFit: Learn-
ing multi-scale graph-convolutional representation for
point cloud normal estimation. In Eur. Conf. Comput.
Vision, pages 651–667, Tel Aviv, Israel. Springer.
Martull, S., Peris, M., and Fukui, K. (2012). Realistic cg
stereo image dataset with ground truth disparity maps.
In ICPR Workshop TrakMark2012, volume 111, pages
117–118.
Mitra, N. J. and Nguyen, A. (2003). Estimating surface nor-
mals in noisy point cloud data. In Proc. Annu. Symp.
Comput. Geometry, pages 322–328, San Diego, CA,
USA. ACM.
Pizer, S. M. and Marron, J. (2017). Object statistics on
curved manifolds. In Statistical Shape Deformation
Anal., pages 137–164. Elsevier.
Saracchini, R. F. V., Stolfi, J., Leitão, H. C. G., Atkinson,
G. A., and Smith, M. L. (2012). A robust multi-
scale integration method to obtain the depth from gra-
dient maps. Comput. Vision Image Understanding,
116(8):882–895.
Silberman, N., Hoiem, D., Kohli, P., and Fergus, R.
(2012). Indoor segmentation and support inference
from RGBD images. In Eur. Conf. Comput. Vision,
pages 746–760, Florence, Italy. Springer.
Ulucan, D., Ulucan, O., and Ebner, M. (2022a). IID-
NORD: A comprehensive intrinsic image decomposi-
tion dataset. In Int. Conf. Image Process., pages 2831–
2835, Bordeaux, France. IEEE.
Ulucan, O., Ulucan, D., and Ebner, M. (2022b). BIO-CC:
Biologically inspired color constancy. In BMVC, Lon-
don, UK. BMVA Press.
Ulucan, O., Ulucan, D., and Ebner, M. (2022c). Color
constancy beyond standard illuminants. In Int. Conf.
Image Process., pages 2826–2830, Bordeaux, France.
IEEE.
Zeng, J., Tong, Y., Huang, Y., Yan, Q., Sun, W., Chen, J.,
and Wang, Y. (2019). Deep surface normal estima-
tion with hierarchical RGB-D fusion. In Conf. Com-
put. Vision Pattern Recognit., Long Beach, CA, USA.
IEEE/CVF.
Zhang, Y. and Funkhouser, T. (2018). Deep depth comple-
tion of a single rgb-d image. In Conf. Comput. Vision
Pattern Recognit., pages 175–185, Salt Lake City, UT,
USA. IEEE/CVF.
Zhou, J., Huang, H., Liu, B., and Liu, X. (2020). Nor-
mal estimation for 3d point clouds via local plane con-
straint and multi-scale selection. Comput. Aided Des.,
129:102916.
IMPROVE 2023 - 3rd International Conference on Image Processing and Vision Engineering
54