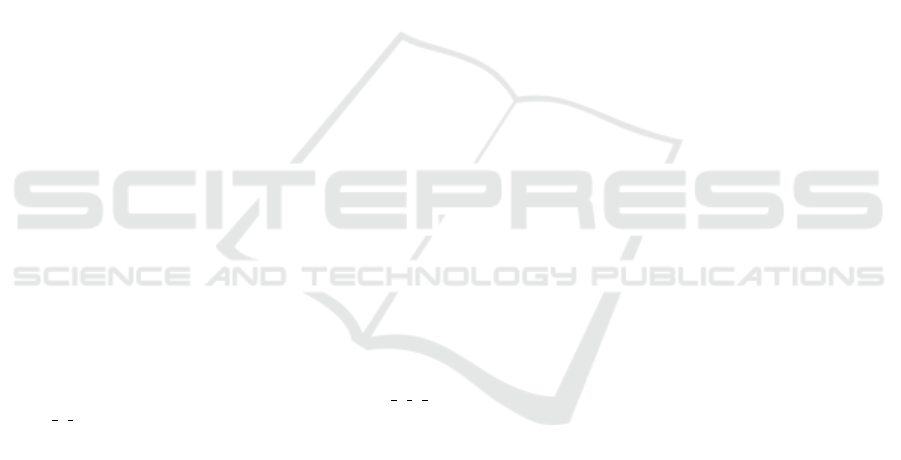
Hakimi Parizi, A., King, M., and Cook, P. (2019). UNBNLP
at SemEval-2019 task 5 and 6: Using language mod-
els to detect hate speech and offensive language. In
Proceedings of the 13th International Workshop on
Semantic Evaluation, pages 514–518, Minneapolis,
Minnesota, USA. Association for Computational Lin-
guistics.
Huang, L., Liu, F., and Zhang, Y. (2021). Overlapping
community discovery for identifying key research
themes. IEEE Transactions on Engineering Manage-
ment, 68(5):1321–1333.
Kansara, C., Gupta, R., Joshi, S. D., and Patil, S. (2016).
Crime mitigation at twitter using big data analytics
and risk modelling. In 2016 International Conference
on Recent Advances and Innovations in Engineering
(ICRAIE), pages 1–5.
Lee, W., Lee, S. S., Chung, S., and An, D. (2007). Harmful
contents classification using the harmful word filtering
and svm. In Shi, Y., van Albada, G. D., Dongarra, J.,
and Sloot, P. M. A., editors, Computational Science –
ICCS 2007, pages 18–25, Berlin, Heidelberg. Springer
Berlin Heidelberg.
Mihalcea, R. and Nastase, V. (2012). Word epoch disam-
biguation: Finding how words change over time. In
Proceedings of the 50th Annual Meeting of the Associ-
ation for Computational Linguistics (Volume 2: Short
Papers), pages 259–263, Jeju Island, Korea. Associa-
tion for Computational Linguistics.
Mikolov, T., Chen, K., Corrado, G., and Dean, J. (2013a).
Efficient estimation of word representations in vector
space. In Bengio, Y. and LeCun, Y., editors, 1st In-
ternational Conference on Learning Representations,
ICLR 2013, Scottsdale, Arizona, USA, May 2-4, 2013,
Workshop Track Proceedings.
Mikolov, T., Sutskever, I., Chen, K., Corrado, G., and
Dean, J. (2013b). Distributed representations of
words and phrases and their compositionality. CoRR,
abs/1310.4546.
MOJ, M. o. J. (2021). White paper on crime
2021. https://hakusyo1.moj.go.jp/jp/68/nfm/n68
2 4
2 2 3.html#h4-2-2-3.
NPA, N. P. A. (2018). Organized crime situation in 2018.
https://www.npa.go.jp/sosikihanzai/kikakubunseki/
sotaikikaku04/h30.sotaijousei.pdf(2020/11/25).
O’Day, D. and Calix, R. (2013). Text message corpus: Ap-
plying natural language processing to mobile device
forensics. pages 1–6.
Ohnishi, H. and Tajima, K. (2013). Discovering new jar-
gons based on skew of word appearance distribution.
DBSJ Journal, 12(1):49–54.
Satoshi ABIKO, Dai HASEGAWA, M. P. K. N. and
SAKUTA, H. (2018). Method for estimation of harm-
fulness of id-exchange bbs based on lexical jargoniza-
tions. Journal of Information Systems Society of
Japan, 13(2):41–58.
Shogo Matsuno, S. M. and Sakaki, T. (2019). Constructing
of the word embedding model by japanese large scale
sns + web corpus. The 33rd Annual Conference of
the Japanese Society for Artificial Intelligence, 2019,
JSAI2019:4Rin113–4Rin113.
Takaoka, K., Hisamoto, S., Kawahara, N., Sakamoto, M.,
Uchida, Y., and Matsumoto, Y. (2018). Sudachi: a
Japanese tokenizer for business. In Proceedings of
the Eleventh International Conference on Language
Resources and Evaluation (LREC 2018), Miyazaki,
Japan. European Language Resources Association
(ELRA).
Wiedemann, G., Ruppert, E., Jindal, R., and Biemann, C.
(2018). Transfer learning from LDA to bilstm-cnn
for offensive language detection in twitter. CoRR,
abs/1811.02906.
Wijaya, D. and Yeniterzi, R. (2011). Understanding seman-
tic change of words over centuries.
Wongcha-um, P. and Allard, T. (2020). Asia-Pacific
drug trade thrives amid the COVID-19 pandemic.
https://www.reuters.com/article/us-asia-crime-
drugs/asia-pacific-drug-trade-thrives-amid-the-
covid-19-pandemic-idUSKBN22R0E0.
Xiang, G., Fan, B., Wang, L., Hong, J., and Rose, C. (2012).
Detecting offensive tweets via topical feature discov-
ery over a large scale twitter corpus. pages 1980–
1984.
Yao, K., Wang, H., Li, Y., Rodrigues, J. J. P. C., and
de Albuquerque, V. H. C. (2021). A group discov-
ery method based on collaborative filtering and knowl-
edge graph for iot scenarios. IEEE Transactions on
Computational Social Systems, pages 1–12.
Yuan, K., Lu, H., Liao, X., and Wang, X. (2018). Reading
thieves’ cant: Automatically identifying and under-
standing dark jargons from cybercrime marketplaces.
In 27th USENIX Security Symposium (USENIX Secu-
rity 18). USENIX Association.
Zhao, K., Zhang, Y., Xing, C., Li, W., and Chen, H. (2016).
Chinese underground market jargon analysis based on
unsupervised learning. In 2016 IEEE Conference on
Intelligence and Security Informatics (ISI), pages 97–
102.
Detection of Compound-Type Dark Jargons Using Similar Words
437