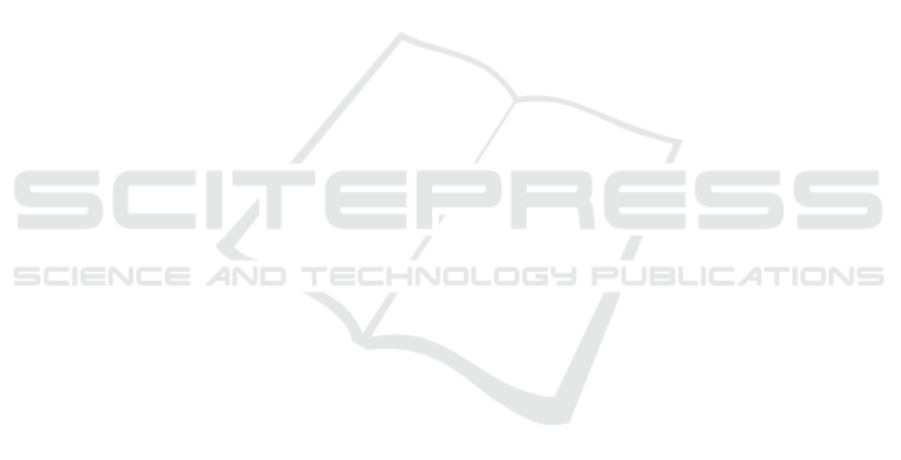
Ashley, E. A. and Niebauer, J. (2004). Conquering the
ECG. Remedica.
Bansal, A. and Joshi, R. (2018). Portable out-of-hospital
electrocardiography: A review of current technolo-
gies. Journal of Arrhythmia, 34(2):129–138.
Bayoumy, K., Gaber, M., Elshafeey, A., Mhaimeed, O.,
Dineen, E. H., Marvel, F. A., Martin, S. S., Muse,
E. D., Turakhia, M. P., Tarakji, K. G., and Elshazly,
M. B. (2021). Smart wearable devices in cardiovascu-
lar care: Where we are and how to move forward. Nat
Rev Cardiol, 18(8):581–599.
Behbahani, S., Dabanloo, N. J., Nasrabadi, A. M., Teixeira,
C. A., and Dourado, A. (2013). Pre-ictal heart rate
variability assessment of epileptic seizures by means
of linear and non-linear analyses. Anatolian Journal
of Cardiology, 13(8):797–803.
Butt, F. S., La Blunda, L., Wagner, M. F., Sch
¨
afer, J.,
Medina-Bulo, I., and G
´
omez-Ullate, D. (2021). Fall
Detection from Electrocardiogram (ECG) Signals and
Classification by Deep Transfer Learning. Informa-
tion, 12(2):63.
Carmo, A. S., Abreu, M., Fred, A. L. N., and da Silva, H. P.
(2022). EpiBOX: An Automated Platform for Long-
Term Biosignal Collection. Front. Neuroinform., 16.
Chatterjee, S., Thakur, R. S., Yadav, R. N., Gupta, L., and
Raghuvanshi, D. K. (2020). Review of noise removal
techniques in ECG signals. IET Signal Processing,
14(9):569–590.
Chen, L., Yu, H., Huang, Y., and Jin, H. (2021). ECG
Signal-Enabled Automatic Diagnosis Technology of
Heart Failure. J Healthc Eng, 2021:5802722.
Chiang, H.-T., Hsieh, Y.-Y., Fu, S.-W., Hung, K.-H., Tsao,
Y., and Chien, S.-Y. (2019). Noise Reduction in ECG
Signals Using Fully Convolutional Denoising Autoen-
coders. IEEE Access, 7:60806–60813.
Christov, I. I. (2004). Real time electrocardiogram QRS de-
tection using combined adaptive threshold. BioMedi-
cal Engineering OnLine, 3(1):28.
Clifford, G. D., Behar, J., Li, Q., and Rezek, I. (2012).
Signal quality indices and data fusion for determining
clinical acceptability of electrocardiograms. Physiol.
Meas., 33(9):1419–1433.
Goodfellow, I., Bengio, Y., and Courville, A. (2016). Deep
Learning. Adaptive Computation and Machine Learn-
ing. The MIT Press, Cambridge, Massachusetts.
Hamilton, P. (2002). Open source ECG analysis. In Com-
puters in Cardiology, pages 101–104.
Huerta, A., Martinez-Rodrigo, A., Rieta, J., Alcaraz, R.,
and IEEE (2021). ECG Quality Assessment via Deep
Learning and Data Augmentation. In 2021 CINC.
Kavanagh, J. J. and Menz, H. B. (2008). Accelerometry: A
technique for quantifying movement patterns during
walking. Gait & Posture, 28(1):1–15.
Kingma, D. P. and Ba, J. (2014). Adam: A Method for
Stochastic Optimization.
Kutz, M. (2010). Biomedical Engineering and Design
Handbook, Volumes I and II (2nd Edition). McGraw-
Hill Professional Publishing, New York, USA.
Li, Q., Mark, R. G., and Clifford, G. D. (2007). Robust heart
rate estimation from multiple asynchronous noisy
sources using signal quality indices and a Kalman fil-
ter. Physiol. Meas., 29(1):15–32.
Li, Q., Rajagopalan, C., and Clifford, G. D. (2014). A
machine learning approach to multi-level ECG signal
quality classification. Comp. Methods Prog. Biomed.,
117(3):435–447.
Mohaddes, F., da Silva, R. L., Akbulut, F. P., Zhou, Y., Tan-
neeru, A., Lobaton, E., Lee, B., and Misra, V. (2020).
A Pipeline for Adaptive Filtering and Transformation
of Noisy Left-Arm ECG to Its Surrogate Chest Signal.
Electronics, 9(5):866.
Mohd Apandi, Z. F., Ikeura, R., Hayakawa, S., and Tsut-
sumi, S. (2020). An Analysis of the Effects of Noisy
Electrocardiogram Signal on Heartbeat Detection Per-
formance. Bioengineering (Basel), 7(2):53.
Munoz-Minjares, J. U., Lopez-Ramirez, M., Vazquez-
Olguin, M., Lastre-Dominguez, C., and Shmaliy, Y. S.
(2021). Outliers detection for accurate HRV-seizure
baseline estimation using modern numerical. Biomed-
ical Signal Processing and Control, 67.
Nonaka, N. and Seita, J. (2022). RandECG: Data Augmen-
tation for Deep Neural Network Based ECG Classi-
fication. In Takama, Y., Matsumura, N., Yada, K.,
Matsushita, M., Katagami, D., Abe, A., Kashima, H.,
Hiraoka, T., Uchiya, T., and Rzepka, R., editors, Ad-
vances in Art. Intel., volume 1423, pages 178–189.
Nurmaini, S., Darmawahyuni, A., Sakti Mukti, A. N., Rach-
matullah, M. N., Firdaus, F., and Tutuko, B. (2020).
Deep Learning-Based Stacked Denoising and Autoen-
coder for ECG Heartbeat Classification. Electronics,
9(1):135.
Pan, Q., Li, X., and Fang, L. (2020). Data Augmentation
for Deep Learning-Based ECG Analysis. In Feature
Engineering and Computational Intelligence in ECG
Monitoring, pages 91–111. Springer, Singapore.
Prieto-Avalos, G., Cruz-Ramos, N. A., Alor-Hern
´
andez,
G., S
´
anchez-Cervantes, J. L., Rodr
´
ıguez-Mazahua, L.,
and Guarneros-Nolasco, L. R. (2022). Wearable De-
vices for Physical Monitoring of Heart: A Review.
Biosensors, 12(5):292.
Raya, M. and Sison, L. (2002). Adaptive noise cancelling of
motion artifact in stress ECG signals using accelerom-
eter. In Proc. of the 2nd Joint 24th Ann. Conf. and
the Ann. Fall Meeting of the Biomedical Eng. Soc.
(IEMBS), volume 2, pages 1756–1757 vol.2.
Reljin, N., Lazaro, J., Hossain, M. B., Noh, Y. S., Cho,
C. H., and Chon, K. H. (2020). Using the Re-
dundant Convolutional Encoder–Decoder to Denoise
QRS Complexes in ECG Signals Recorded with an
Armband Wearable Device. Sensors, 20(16):4611.
Rodrigues, J., Belo, D., and Gamboa, H. (2017). Noise
detection on ECG based on agglomerative clustering
of morphological features. Computers in Biology and
Medicine, 87:322–334.
Saraiva, J. (nov-2022a). Deep Residual Learning for
Epileptic Seizure Prediction and Tools to Expedite
Biosignal Research. MSc. Thesis, Department of
Computer Science and Engineering, Instituto Superior
T
´
ecnico, Universidade de Lisboa, Lisbon.
BIOSIGNALS 2023 - 16th International Conference on Bio-inspired Systems and Signal Processing
144