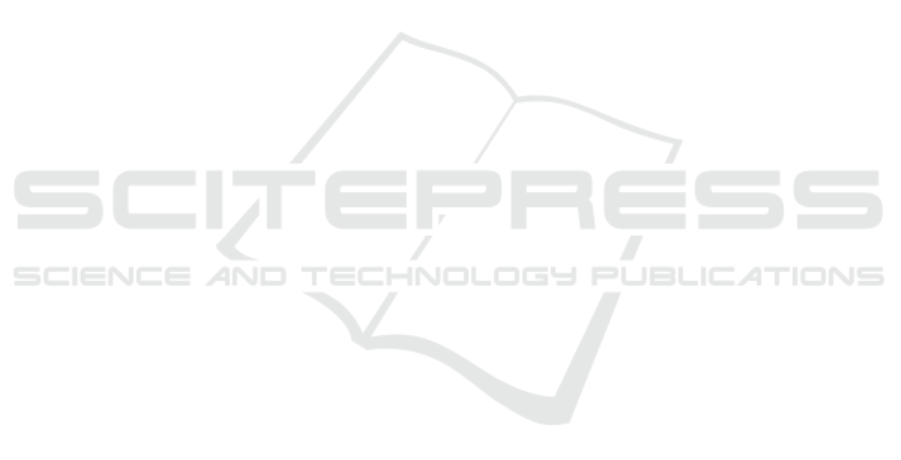
and management of its configurations to be stored in
a knowledge-base. The facts in the knowledge-base
are further exploited with the help of a Cyber Physi-
cal Recommendation System (CPRS). In this context,
the CPRS presents a model architecture that may pro-
vide necessary recommendations for building a CPS
according to the presented objective, details, and ap-
plication scenario. In the future work, we plan the
to improve the knowledge-base using the training and
testing phase with the help of natural language pro-
cessing algorithms and techniques. While this must
also provide means to explore the explainabilty as-
pects of such a supporting system.
ACKNOWLEDGEMENTS
The authors would like to thank the University of the
Littoral Cote d’Opale, and the Lebanese University
for the financial support.
REFERENCES
Banerjee, A., Gupta, S. K., Fainekos, G., and Varsamopou-
los, G. (2011). Towards modeling and analysis of
cyber-physical medical systems. In Proceedings of the
4th International Symposium on Applied Sciences in
Biomedical and Communication Technologies, pages
1–5.
Chung, D. S. (2017). STUDENT NUMBER: 2708062
COURSE NAME: CIS 601 DEPARTMENT: Depart-
ment of electrical engineering and computer science.
page 22.
Djouad, A., Atil, F., and Beddiar, C. (2018). Domain model
for cyber-physical systems. page 8.
Fallah, Y. P., Huang, C., Sengupta, R., and Krishnan, H.
(2010). Design of cooperative vehicle safety systems
based on tight coupling of communication, comput-
ing and physical vehicle dynamics. In Proceedings of
the 1st ACM/IEEE international conference on cyber-
physical systems, pages 159–167.
Garouani, M., Ahmad, A., Bouneffa, M., and Hamlich, M.
(2022a). AMLBID: An auto-explained automated ma-
chine learning tool for big industrial data. SoftwareX,
17:100919.
Garouani, M., Ahmad, A., Bouneffa, M., Hamlich, M.,
Bourguin, G., and Lewandowski, A. (2022b). Towards
big industrial data mining through explainable auto-
mated machine learning. The International Journal
of Advanced Manufacturing Technology, 120:1169–
1188.
Garouani, M., Ahmad, A., Bouneffa, M., Hamlich, M.,
Bourguin, G., and Lewandowski, A. (2022c). Using
meta-learning for automated algorithms selection and
configuration: an experimental framework for indus-
trial big data. Journal of Big Data, 9:1169–1188.
Garouani., M., Ahmad., A., Bouneffa., M., Lewandowski.,
A., Bourguin., G., and Hamlich., M. (2021). Towards
the automation of industrial data science: A meta-
learning based approach. In Proceedings of the 23rd
International Conference on Enterprise Information
Systems - Volume 1: ICEIS, pages 709–716.
Garouani, M., Ahmad, A., Bouneffa, M., Lewandowski,
A., Bourguin, G., and Hamlich, M. (2022d). Towards
meta-learning based data analytics to better assist the
domain experts in industry 4.0. In Artificial Intelli-
gence in Data and Big Data Processing, pages 59–66,
Cham. Springer International Publishing.
Ge, Y., Dong, Y., and Zhao, H. (2012). A cyber-physical en-
ergy system architecture for electric vehicles charging
application. In 2012 12th International Conference on
Quality Software, pages 246–250. IEEE.
Haque, S. A., Aziz, S. M., and Rahman, M. (2014). Review
of cyber-physical system in healthcare. 10(4):217415.
Publisher: SAGE Publications Sage UK: London,
England.
Jamwal, A., Agrawal, R., Manupati, V. K., Sharma, M.,
Varela, L., and Machado, J. (2020). Development
of cyber physical system based manufacturing system
design for process optimization. 997(1):012048.
Lee, J., Bagheri, B., and Kao, H.-A. (2015). A cyber-
physical systems architecture for industry 4.0-based
manufacturing systems. 3:18–23.
Lee, J., Jin, C., and Bagheri, B. (2017). Cyber physical
systems for predictive production systems. 11.
Oks, S. J., Jalowski, M., Fritzsche, A., and M
¨
oslein, K. M.
(2019). Cyber-physical modeling and simulation: A
reference architecture for designing demonstrators for
industrial cyber-physical systems. 84:257–264. Pub-
lisher: Elsevier.
Raisin, S. N., Jamaludin, J., Rahalim, F. M., Mohamad, F.
A. J., and Naeem, B. (2020). Cyber-physical system
(CPS) application- a REVIEW. 1(2):52–65. Number:
2.
Rajkumar, R. R., Lee, I., Sha, L., and Stankovic, J. (2010).
Cyber-physical systems: the next computing revolu-
tion. In Proceedings of the 47th Design Automation
Conference on - DAC ’10, page 731. ACM Press.
V
´
agner, A. (2018). Store and visualize EER in neo4j. In
Proceedings of the 2nd International Symposium on
Computer Science and Intelligent Control. ACM.
Wan, J., Yin, B., Li, D., Celesti, A., Tao, F., and Hua,
Q. (2018). An ontology-based resource reconfigu-
ration method for manufacturing cyber-physical sys-
tems. 23(6):2537–2546. Publisher: IEEE.
Yan, J., Zhang, M., and Fu, Z. (2019). An intralogistics-
oriented cyber-physical system for workshop in the
context of industry 4.0. 35:1178–1183.
Zheng, B., Lin, C.-W., Liang, H., Shiraishi, S., Li, W., and
Zhu, Q. (2017). Delay-aware design, analysis and ver-
ification of intelligent intersection management. In
2017 IEEE International Conference on Smart Com-
puting (SMARTCOMP), pages 1–8. IEEE.
Automated Decision Support Framework for IoT: Towards a Cyber Physical Recommendation System
373