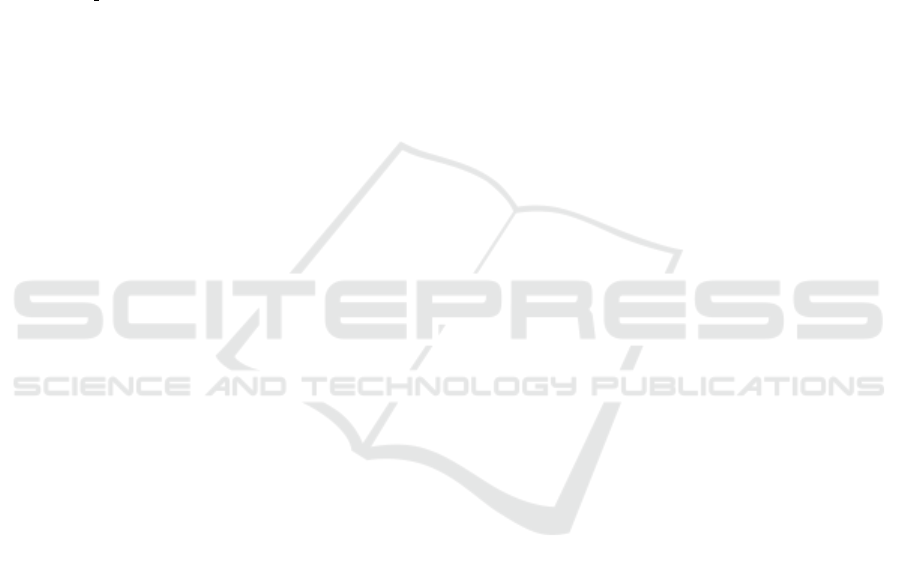
Daudt, R. C., Le Saux, B., Boulch, A., and Gousseau, Y.
(2018). Urban change detection for multispectral earth
observation using convolutional neural networks. In
2018 IEEE IGARSS.
Fan, T., Wang, G., Li, Y., and Wang, H. (2020). Ma-net:
A multi-scale attention network for liver and tumor
segmentation. IEEE Access, 8:179656–179665.
Hao Chen, Z. Q. and Shi, Z. (2021). Remote sensing image
change detection with transformers. IEEE TGRS.
Ji, S., Wei, S., and Lu, M. (2019). Fully convolutional
networks for multisource building extraction from an
open aerial and satellite imagery data set. IEEE TGRS.
Kaiyu Li, Fulin Sun, X. L. (2021). Change de-
tection pytorch. URL: https://github.com/likyoo/
change detection.pytorch.
Kumar, R., Dabral, R., and Sivakumar, G. (2021).
Learning unsupervised cross-domain image-to-image
translation using a shared discriminator. CoRR,
abs/2102.04699.
Li, B., Liu, Y., and Wang, X. (2019). Gradient harmonized
single-stage detector. In AAAI Conference on Artifi-
cial Intelligence.
Li, H., Xiong, P., An, J., and Wang, L. (2018). Pyramid
attention network for semantic segmentation. arXiv
preprint arXiv:1805.10180.
Li, X., Yang, J., and et. al (2020). Generalized Focal Loss:
Learning Qualified and Distributed Bounding Boxes
for Dense Object Detection. arXiv:2006.04388.
Lin, T.-Y., Maire, M., Belongie, S., Bourdev, L., Girshick,
R., Hays, J., Perona, P., Ramanan, D., Zitnick, C. L.,
and Doll
´
ar, P. (2014). Microsoft coco: Common ob-
jects in context.
Liu, M., Chai, Z., Deng, H., and Liu, R. (2022). A
cnn-transformer network with multi-scale context ag-
gregation for fine-grained cropland change detection.
IEEE Journal of Selected Topics in Applied Earth Ob-
servations and Remote Sensing.
Lu, X., Li, B., Yue, Y., Li, Q., and Yan, J. (2019). Grid
r-cnn. In Proceedings of the IEEE Conference on
CVPR.
Maharashtra Remote Sensing Application Centre
(MRSAC) (2015). Jalyukt shivar , water con-
servation department, mantralaya, mumbai.
Source:http://mrsac.maharashtra.gov.in/jalyukt/.
Online; Accessed: 2019-09-15.
Prasad, P., Damani, O. P., and Sohoni, M. (2022). How can
resource-level thresholds guide sustainable intensifi-
cation of agriculture at farm level? a system dynamics
study of farm-pond based intensification. Agricultural
Water Management.
Redmon, J. and Farhadi, A. (2018). Yolov3: An incremental
improvement. CoRR, abs/1804.02767.
Ren, S., He, K., Girshick, R., and Sun, J. (2017). Faster
r-cnn: Towards real-time object detection with region
proposal networks. In IEEE TPAMI, number 6.
Ronneberger, O., Fischer, P., and Brox, T. (2015). U-net:
Convolutional networks for biomedical image seg-
mentation. In MICCAI.
Russell, B. C., Torralba, A., Murphy, K. P., and Freeman,
W. T. (2008). Labelme: a database and web-based
tool for image annotation. IJCV.
Shao, R., Du, C., Chen, H., and Li, J. (2021). Sunet: Change
detection for heterogeneous remote sensing images
from satellite and uav using a dual-channel fully con-
volution network. Remote Sensing.
Shen, L., Lu, Y., Chen, H., Wei, H., Xie, D., Yue, J., Chen,
R., Lv, S., and Jiang, B. (2021). S2looking: A satel-
lite side-looking dataset for building change detection.
Remote Sensing, 13(24).
Shi, Q., Liu, M., Li, S., Liu, X., Wang, F., and Zhang, L.
(2022). A deeply supervised attention metric-based
network and an open aerial image dataset for remote
sensing change detection. IEEE TGRS.
Tian, S., Zheng, Z., Ma, A., and Zhong, Y. (2020).
Hi-ucd: A large-scale dataset for urban semantic
change detection in remote sensing imagery. CoRR,
abs/2011.03247.
Tundia., C., Kumar., R., Damani., O., and Sivakumar., G.
(2022). The mis check-dam dataset for object detec-
tion and instance segmentation tasks. In VISAPP.
Tundia, C., Tank, P., and Damani, O. (2020). Aiding Irri-
gation Census in Developing Countries by Detecting
Minor Irrigation Structures from Satellite Imagery. In
GISTAM.
Van Etten, A., Hogan, D., Manso, J. M., Shermeyer, J.,
Weir, N., and Lewis, R. (2021). The multi-temporal
urban development spacenet dataset. In Proceedings
of the IEEE/CVF CVPR.
Wu, C., Zhang, L., and Du, B. (2017). Kernel slow feature
analysis for scene change detection. IEEE TGRS.
Xiao, T., Liu, Y., Zhou, B., Jiang, Y., and Sun, J. (2018).
Unified perceptual parsing for scene understanding. In
Proceedings of the ECCV.
Zhan, Y., Fu, K., Yan, M., Sun, X., Wang, H., and Qiu,
X. (2017). Change detection based on deep siamese
convolutional network for optical aerial images. IEEE
Geoscience and Remote Sensing Letters.
Zhang, C., Yue, P., Tapete, D., Jiang, L., Shangguan, B.,
Huang, L., and Liu, G. (2020). A deeply supervised
image fusion network for change detection in high res-
olution bi-temporal remote sensing images. ISPRS
Journal of Photogrammetry and Remote Sensing.
Zhang, M., Xu, G., Chen, K., Yan, M., and Sun, X. (2019).
Triplet-based semantic relation learning for aerial re-
mote sensing image change detection. IEEE Geo-
science and Remote Sensing Letters.
Zhao, H., Shi, J., Qi, X., Wang, X., and Jia, J. (2017). Pyra-
mid scene parsing network. In Proceedings of the
IEEE conference on CVPR.
Zhou, Z., Rahman Siddiquee, M. M., Tajbakhsh, N., and
Liang, J. (2018). Unet++: A nested u-net architecture
for medical image segmentation. In Deep learning in
medical image analysis and multimodal learning for
clinical decision support.
FPCD: An Open Aerial VHR Dataset for Farm Pond Change Detection
869