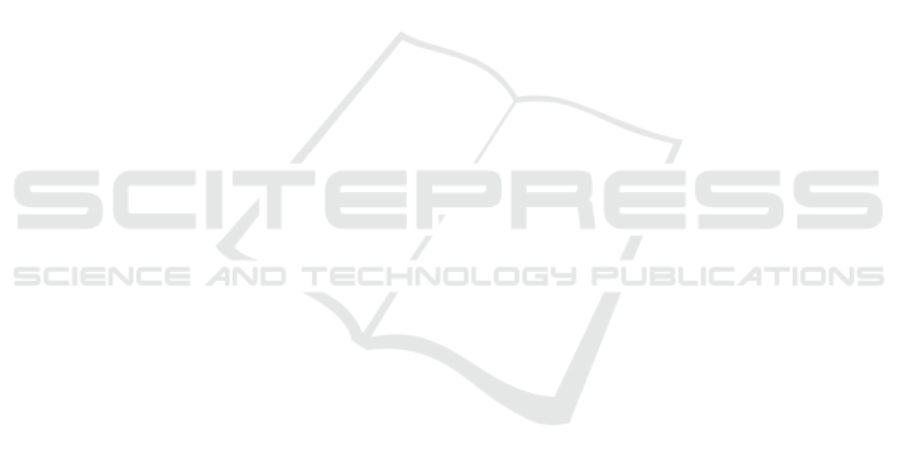
images of driver fatigue, such as yawning. In future
works, we intend to improve this aspect through the
dataset and test the LIAE model proposed by DFL. In
the next step, we intend to develop the integration be-
tween STEP 1 and STEP 2 to automate the process of
generating these datasets.
ACKNOWLEDGMENTS
The authors would like to thank CAPES, CNPq and
the Federal University of Ouro Preto for support-
ing this work. This study was financed in part
by the Coordenac¸
˜
ao de Aperfeic¸oamento de Pes-
soal de N
´
ıvel Superior - Brasil (CAPES) - Finance
Code 001, the Conselho Nacional de Desenvolvi-
mento Cient
´
ıfico e Tecnol
´
ogico (CNPQ) and the Uni-
versidade Federal de Ouro Preto (UFOP).
REFERENCES
Ahmed, A. A. and Echi, M. (2021). Hawk-eye: An ai-
powered threat detector for intelligent surveillance
cameras. IEEE Access, 9:63283–63293.
Alajrami, E., Tabash, H., Singer, Y., and El Astal, M.-T.
(2019). On using ai-based human identification in im-
proving surveillance system efficiency. In 2019 In-
ternational Conference on Promising Electronic Tech-
nologies (ICPET), pages 91–95. IEEE.
Arjovsky, M., Chintala, S., and Bottou, L. (2017). Wasser-
stein generative adversarial networks. In Interna-
tional conference on machine learning, pages 214–
223. PMLR.
Boudjit, K. and Ramzan, N. (2022). Human detection based
on deep learning yolo-v2 for real-time uav applica-
tions. Journal of Experimental & Theoretical Artifi-
cial Intelligence, 34(3):527–544.
Cai, Y., Wang, X., Yu, Z., Li, F., Xu, P., Li, Y., and Li, L.
(2019). Dualattn-gan: Text to image synthesis with
dual attentional generative adversarial network. IEEE
Access, 7:183706–183716.
Dang, H., Liu, F., Stehouwer, J., Liu, X., and Jain, A. K.
(2020). On the detection of digital face manipula-
tion. In Proceedings of the IEEE/CVF Conference
on Computer Vision and Pattern recognition, pages
5781–5790.
Eldrandaly, K. A., Abdel-Basset, M., and Abdel-Fatah, L.
(2019). Ptz-surveillance coverage based on artificial
intelligence for smart cities. International Journal of
Information Management, 49:520–532.
Goodfellow, I., Pouget-Abadie, J., Mirza, M., Xu, B.,
Warde-Farley, D., Ozair, S., Courville, A., and Ben-
gio, Y. (2014). Generative adversarial nets. Advances
in neural information processing systems, 27.
Gorospe, J., Mulero, R., Arbelaitz, O., Muguerza, J., and
Ant
´
on, M.
´
A. (2021). A generalization performance
study using deep learning networks in embedded sys-
tems. Sensors, 21(4):1031.
Guo, C., Huang, D., Zhang, J., Xu, J., Bai, G., and Dong, N.
(2020). Early prediction for mode anomaly in gener-
ative adversarial network training: an empirical study.
Information Sciences, 534:117–138.
Jalalifar, S. A., Hasani, H., and Aghajan, H. (2018).
Speech-driven facial reenactment using conditional
generative adversarial networks. arXiv preprint
arXiv:1803.07461.
Korshunova, I., Shi, W., Dambre, J., and Theis, L. (2017).
Fast face-swap using convolutional neural networks.
In Proceedings of the IEEE international conference
on computer vision, pages 3677–3685.
Kumar, R., Sotelo, J., Kumar, K., de Br
´
ebisson, A., and
Bengio, Y. (2017). Obamanet: Photo-realistic lip-sync
from text. arXiv preprint arXiv:1801.01442.
Li, Y. and Lyu, S. (2018). Exposing deepfake videos
by detecting face warping artifacts. arXiv preprint
arXiv:1811.00656.
Liu, Y., Kong, L., Chen, G., Xu, F., and Wang, Z. (2021).
Light-weight ai and iot collaboration for surveillance
video pre-processing. Journal of Systems Architec-
ture, 114:101934.
Luo, H., Liu, J., Fang, W., Love, P. E., Yu, Q., and Lu, Z.
(2020). Real-time smart video surveillance to man-
age safety: A case study of a transport mega-project.
Advanced Engineering Informatics, 45:101100.
Lv, X., Su, M., and Wang, Z. (2021). Application of face
recognition method under deep learning algorithm in
embedded systems. Microprocessors and Microsys-
tems, page 104034.
Mirsky, Y. and Lee, W. (2021). The creation and detec-
tion of deepfakes: A survey. ACM Computing Surveys
(CSUR), 54(1):1–41.
Morishita, F., Kato, N., Okubo, S., Toi, T., Hiraki, M.,
Otani, S., Abe, H., Shinohara, Y., and Kondo, H.
(2021). A cmos image sensor and an ai accelerator
for realizing edge-computing-based surveillance cam-
era systems. In 2021 Symposium on VLSI Circuits,
pages 1–2. IEEE.
Nath, N. D., Behzadan, A. H., and Paal, S. G. (2020). Deep
learning for site safety: Real-time detection of per-
sonal protective equipment. Automation in Construc-
tion, 112:103085.
Nguyen, H. H., Fang, F., Yamagishi, J., and Echizen, I.
(2019). Multi-task learning for detecting and seg-
menting manipulated facial images and videos. In
2019 IEEE 10th International Conference on Biomet-
rics Theory, Applications and Systems (BTAS), pages
1–8. IEEE.
Pavan Kumar, M. and Jayagopal, P. (2021). Generative ad-
versarial networks: a survey on applications and chal-
lenges. International Journal of Multimedia Informa-
tion Retrieval, 10(1):1–24.
Perov, I., Gao, D., Chervoniy, N., Liu, K., Marangonda, S.,
Um
´
e, C., Dpfks, M., Facenheim, C. S., RP, L., Jiang,
J., et al. (2020). Deepfacelab: Integrated, flexible and
extensible face-swapping framework. arXiv preprint
arXiv:2005.05535.
VISAPP 2023 - 18th International Conference on Computer Vision Theory and Applications
860